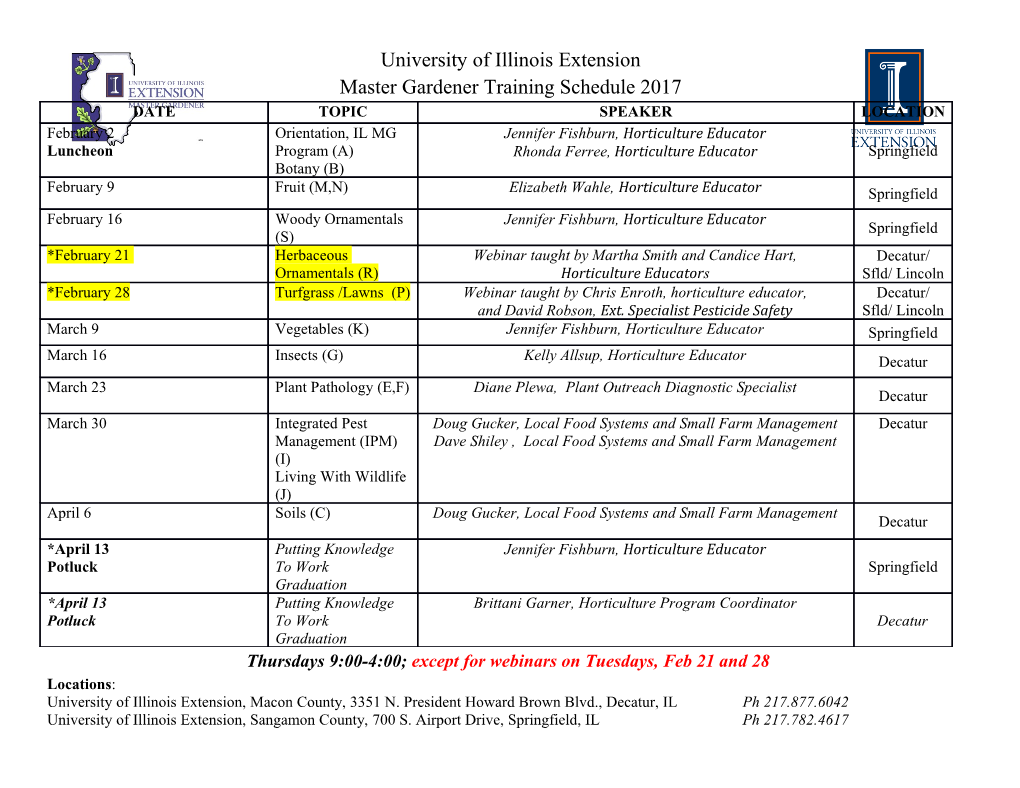
Extracting Entailing Words from Small Corpora for Ontology Building Aurelie Herbelot Computer Laboratory University of Cambridge J.J. Thompson Avenue, Cambridge, United Kingdom [email protected] Abstract Pantel and Lin (2002) followed in her path using distributional similarity to extract large clusters of This paper explores the extraction of con- related words from corpora. ceptual clusters from a small corpus, given The main advantage of the clustering method is user-defined seeds. We use the distribu- that it allows users to find hyponymic relations that tional similarity hypothesis (Harris, 1968) are not explicitly mentioned in the corpus. One to gather similar terms, using semantic major drawback is that the extracted clusters must features as context. We attempt to pre- then be appropriately named - a task that Pantel and serve both precision and recall by using Ravichandran (2004) showed has no simple solu- a bootstrapping algorithm with reliability tion. Furthermore, although mining a corpus for all calculations proposed by Pantel and Pen- its potential clusters may be a good way to extract nacchiotti (2006). Precision of up to large amounts of information, it is not a good way to 78% is achieved for our best query over a answer specific user needs. For instance, if I wish to 16MB corpus. We find, however, that re- compile a list of all animals, cities or motion verbs in sults are dependent on initial settings and my corpus, I must mine the whole text, hope that my propose a partial solution to automatically query will be answered by one of the retrieved clus- select appropriate seeds. ters and identify the correct group. Finally, previous work has suggested that clustering is only reliable 1 Introduction for large corpora: Pantel and Pennacchiotti (2006) The entailment task can be described as finding pairs claim that it is not adequate for corpora under 100 of words so that one (the entailed word) can replace million words. the other (the entailing word) in some contexts. Or This paper proposes a user-driven approach to in other words, it consists in finding words in a hy- clustering where example seeds are given to the sys- ponym/hypernym relation. This is of course also tem, patterns extracted for those seeds and similar the task of ontology extraction when applied to the words subsequently returned, following the typical is-a relationship. Hence, entailment tools such as entailment scenario. The obvious difficulty, from an those based on the distributional similarity hypoth- ontology extraction point of view, is to overcome esis (Harris, 1968) can also be used in ontology ex- data sparsity without compromising precision (the traction, as shown by Pantel and Lin (2002) in a clus- original handful of seeds might not produce many tering task. accurate patterns). We therefore investigate the use The clustering method in ontology extraction was of bootstrapping on one hand in order to raise the pioneered by Caraballo (1999). She showed that number of extractions and of semantic features with semantically-related words could be clustered to- reliability calculations on the other hand to help gether by mining coordinations and conjunctions. maintain precision to an acceptable level. The next section reviews relevant previous work lationships which were filtered for taxonomic pairs. and includes a description of the piece of work (The filtering simply consisted in checking whether which motivated the research presented here. We the hyponym and hypernym were animal names, then describe our algorithm and experimental setup. using a list compiled from Wikipedia article titles.) Results for the whole corpus (16MB) and a 10% A careful evaluation was performed, both manually subset are presented and discussed. Problems re- on a subset of the results and automatically on the lating to initial setting sensitivity are noted, and a whole extracted file using the NCBI1 taxonomy. We partial solution proposed. We finally conclude with reported a precision of 88.5% and a recall of 20%. avenues for future work. This work highlighted the fact that the dictionaries of animal names that we had at our disposal (both 2 Previous Work and Motivation the list extracted from Wikipedia and the NCBI itself) were far from comprehensive and therefore The clustering method represents so far a marginal affected our recall. approach in a set of ontology extraction techniques In this paper, we attempt to remedy the short- dominated by the lexico-syntactic pattern-matching comings of our dictionaries and investigate a min- method (Hearst, 1992). Clustering was initially pro- ing algorithm which returns conceptual clusters out posed by Caraballo (1999) who used conjunction of a small, consistent corpus. The fairly convention- and coordination to cluster similar words. She ob- alised aspect of Wikipedia articles (the structure and tained a precision of 33% on her hyponymy extrac- vocabulary become standardised with usage) tends tion task. to produce good, focused contexts for certain types Pantel and Lin (2002), following from Cara- of words or relationships, and this partly overcomes ballo’s work, proposed their ‘clustering by com- the data sparsity problem. We therefore propose the mittee’ algorithm, using distributional similarity to realistic task of finding clusters of terms that a reader cluster similar words. Their algorithm distinguishes of biological texts might be interested in. Here, between various senses of a word. Pantel and specifically, we focus on animal names, geograph- Ravichandran (2004) report that the algorithm has ical areas (i.e. potential animal habitats) and parts a precision of 68% over a 3GB corpus (the figure of the animal body. We reuse the Wikipedia corpus is calculated over the relation between clusters and from our previous work - 16MB of plain text - and their automatically generated names.) apply to it distributional similarity using semantic On the entailment front, Geffet and Dagan (2005) features, with a bootstrapping algorithm proposed also used distributional similarity over an 18 million by Pantel and Pennacchiotti (2006). word corpus and obtained up to 74% precision with a novel feature weighting function (RFF) and an In- 3 The Algorithm clusion Testing algorithm which uses the the k char- The aim of the algorithm is to find words that are acteristic features common to two words in an en- similar to the seeds provided by the user. In order to tailment relation. achieve this, we use the distributional similarity hy- Our own investigation derives from a pothesis (Harris, 1968) which states that words that previous ontology extraction project (Her- appear in the same context are semantically related. belot and Copestake, 2006) on Wikipedia Our ‘context’ consists here of the semantic triples (http://www.wikipedia.org/). That in which a word appears, with the semantics of the project focused on uncovering taxonomic rela- text referring to its RMRS representation (Copes- tionships in a corpus consisting of over 12,000 take, 2004). So for instance, in the sentence ‘the Wikipedia pages on animals. We extracted a cat chased a green mouse’, the word ‘mouse’ has a semantic representation of the text in RMRS form context comprising two triples: (Copestake, 2004) and manually defined patterns lemma:chase pos:v arg:ARG2 var:mouse pos:n characteristic of the taxonomic relationship, also which indicates that ‘mouse’ is object of ‘chase’ and in RMRS format. Matching those patterns to the text’s semantics allowed us to return hyponymic re- 1www.ncbi.nlm.nih.gov/entrez/query.fcgi?db=Taxonomy lemma:green pos:j arg:ARG1 var:mouse pos:n P (f, i) is the probability that they appear together. which indicates that the argument of ‘green’ is Pointwise Mutual Information is known for pro- ‘mouse’. ducing scores in favour of rare events. In order Let’s assume that ‘mouse’ is one of the seeds to counterbalance this effect, our figures are multi- provided to the system. We can transform its plied by the discount factor suggested in Pantel and context into generic features by replacing the slots Ravichandran (2004): containing the seed with a hole: lemma:chase pos:v arg:ARG2 lemma:mouse pos:n becomes c min (c , c ) d = if ∗ i f (2) lemma:chase pos:v arg:ARG2 lemma:hole cif + 1 min (ci, cf ) + 1 pos:n. Then, every time a generic feature is encoun- where cif is the cooccurrence count of an instance tered in the text, we can hypothesise that whichever and a feature, ci the frequency count of instance i word fills the hole position is semantically similar to and cf the frequency count of feature f. our seed: if we encounter the triple lemma:chase We then find the reliability of the feature as: pos:v arg:ARG2 var:bird pos:n, we hypothe- P pmi(i,f) sise that ‘bird’ belongs to the same semantic class iI max ∗ ri r = pmi (3) as ‘mouse’. (We assume that a match on any one f |I| feature - as opposed to the whole context – is suffi- cient to hypothesise the presence of an instance.) where rf and ri are the reliabilities of the feature and We initially extract all the features that include of an instance respectively, and I is the total number one of the seeds presented to the system. We filter of instances extracted by f. those features so that semantically weak relations, Initially, the seeds have reliability 1 and all the such as the one between a preposition and its argu- other words reliability 0. We then select features ment, are discarded: triples containing a preposition with n-best reliabilities. Those features are used to or quantifier as the head or argument of the relation extract new instances, the reliability of which is cal- are deleted. At the moment, we are also leaving culated in the same fashion as for the initial patterns: conjunction aside.
Details
-
File Typepdf
-
Upload Time-
-
Content LanguagesEnglish
-
Upload UserAnonymous/Not logged-in
-
File Pages8 Page
-
File Size-