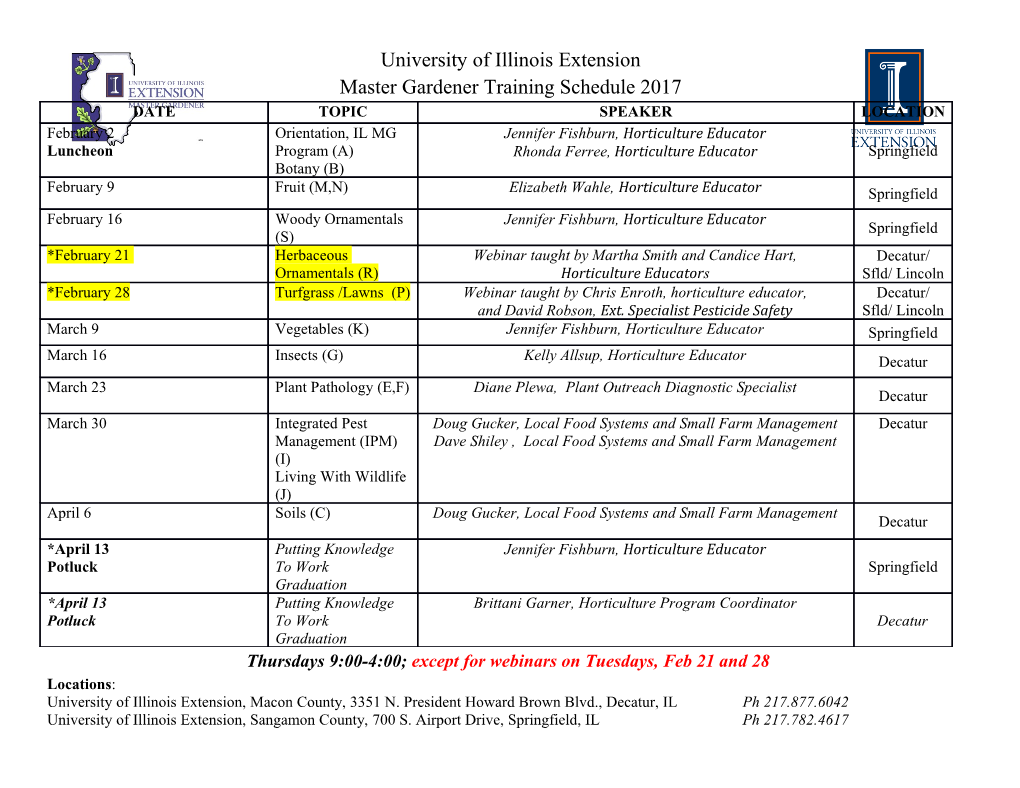
Spatial Databases and Spatial Indexing Techniques Timos Sellis Computer Science Division Department of Electrical and Computer Engineering National Technical University of Athens Zografou 15773, GREECE Tel: +30-1-772-1601 FAX: +30-1-772-1659 e-mail: [email protected] Spatial Database Systems Timos Sellis Spatial Databases and Spatial Indexing Techniques Timos Sellis National Technical University of Athens e-mail: [email protected] Aalborg, June 1998 Outline • Data Models • Algebra • Query Languages • Data Structures • Query Processing and Optimization • System Architecture • Open Research Issues Spatial Database Systems 1 1 Spatial Database Systems Timos Sellis Introduction : Spatial Database Management Systems (SDBMS) QUESTION “What is a Spatial Database Management System ?” ANSWER • SDBMS is a DBMS • It offers spatial data types in its data model and query language • support of spatial relationships / properties / operations • It supports spatial data types in its implementation • efficient indexing and retrieval • support of spatial selection / join Spatial Database Systems 2 Applications of SDBMS Traditional GIS applications • Socio-Economic applications • Urban planning • Route optimization, market analysis • Environmental applications • Fire or Pollution Monitoring • Administrative applications • Public networks administration • Vehicle navigation Spatial Database Systems 3 2 Spatial Database Systems Timos Sellis Applications of SDBMS (cont'd) Novel applications • Image and Multimedia databases • shape configuration and similarity issues • medical databases • Time-series databases • management of time intervals • Traditional DBMS • data warehouses Spatial Database Systems 4 SDBMS Requirements • Manipulation of very large amounts of data e.g. terabytes of data per day from satellite images • Data distinction spatial and non-spatial (alphanumeric) data • Complex spatial relationships and operations topological, directional, metric relationships, spatial join operation Spatial Database Systems 5 3 Spatial Database Systems Timos Sellis SDBMS Requirements (cont'd) • Complex spatial relationships (topological, directional,metric) • “Find all cities adjacent to a river” • “Find all dark shapes left to the heart” • “Find the 5 closest hospitals with respect to a given location” • Spatial join: An expensive operation • “Find the 5 closest hospitals with respect to any highway” Spatial Database Systems 6 SDBMS Issues of Interest • Data Models • Algebras • Query Languages • Data Structures • System Architectures Spatial Database Systems 7 4 Spatial Database Systems Timos Sellis Spatial Data Models • Two main approaches for spatial representation • raster model R (image-based partition of space) R R R H R R R R R • vector model (object-based partition of space) Y-Axis House River X-Axis Spatial Database Systems 8 Raster Model • RASTER MODEL subdivision of space into cells of regular size and shape (i.e., regular tessellation) square triangular hexagonal Spatial Database Systems 9 5 Spatial Database Systems Timos Sellis Raster Model (cont'd) • RASTER MODEL (cont'd) • each cell is assigned the value of the attribute it represents • each cell in a raster file is assigned only one value • different attributes are stored in separate files (layers) Spatial Database Systems 10 Raster Model (cont'd) • RASTER MODEL (cont'd) Example: land cover land layer Area water resources water layer topography topography layer Spatial Database Systems 11 6 Spatial Database Systems Timos Sellis Vector Model • VECTOR MODEL • subdivision of the space based on geographic features position (i.e., irregular tessellation) • features are represented by (2-D space): • Points (x,y) • Lines (x1,y1, x2,y2, ..., xn,yn) • Regions (x1,y1, ..., xn,yn, x1,y1) ... referred to a common coordinate system (X,Y) Spatial Database Systems 12 Vector Model (cont'd) • VECTOR MODEL (cont'd) • Layer-based model: features organised into separate layers (files) based on their properties • Feature-based model: features organised into one layer (file) and characterised by a code (closer to O- O approach) Spatial Database Systems 13 7 Spatial Database Systems Timos Sellis Spatial Algebra Spatial operations • Local • information retrieval (e.g. point-in-polygon query) • classification and recoding • measurement (e.g. area, perimeter) • polygon overlay / spatial join Spatial Database Systems 14 Spatial Algebra (cont'd) Spatial operations (cont'd) • Zonal • spatial selection • Focal • proximity determination • (e.g. Voronoi diagrams) • interpolation Spatial Database Systems 15 8 Spatial Database Systems Timos Sellis Spatial Query Languages • Database query languages are the tools that end-users most often use to interact with a spatial database system. • Such a language should be: • powerful enough to express a query involving both spatial and non-spatial components • simple enough to use effectively as an interface between user and system Spatial Database Systems 16 Spatial Query Languages (cont'd) Basic queries: Spatial selection • Find all rivers within a specified area • Find all cities within a 100Km distance from Athens Spatial join • Find all cities within a 10Km distance from any shoreline. Spatial Query Languages • New languages ‘from-the-scratch’ (e.g. GEO-SAL [SH91]) • Extensions of well-known languages such as SQL, QUEL (e.g. GEOQL [OSM89], PSQL [RFS88]) Spatial Database Systems 17 9 Spatial Database Systems Timos Sellis PSQL • Pictorial Structured Query Language (PSQL) [RFS88]: An SQL extension, which supports: • spatial entity types (point, segment, region), and • spatial operators • topological (e.g. overlaps, covers, within) • directional (e.g. north_of, south_of) Spatial Database Systems 18 PSQL (cont'd) PSQL Syntax: SELECT < attribute-list > FROM < relation-list > ON < picture-list > WHERE < condition > Example: SELECT state, state_region, population_density FROM states, cities ON us_map WHERE state_region overlap circle (location, 1500) AND city_name = “Washington, D.C.” Spatial Database Systems 19 10 Spatial Database Systems Timos Sellis Spatial Data Structures Requirements • Specialized data structures are necessary, for performance, uniformity, etc. • Point- and non-point objects need to be efficiently indexed and retrieved • Support of several spatial relationships is necessary Spatial Database Systems 20 Spatial Data Structures (cont'd) Examples • Raster Model: • Quadtrees • Vector Model: • K-D-B-trees, Quadtrees, Grid Files (for points), • R-trees and variations (for non-point objects) Spatial Database Systems 21 11 Spatial Database Systems Timos Sellis Data Structures, Raster Model • Quadtrees [Sam84] Data set Representation Quadtree root 0 1 20 21 0123 3 22 23 20 21 22 23 Spatial Database Systems 22 Data Structures, Vector Model • Using approximations instead of the exact geometry of shapes e.g. the Minimum Bounding Rectangle (MBR) NO FI IC SW Example: UK DE IR GE PL NL CZ BE LU FR AU RO HU CH BU YU AL PO SP IT GR Spatial Database Systems 23 12 Spatial Database Systems Timos Sellis Data Structures, Vector Model (cont'd) • Two-step query processing • Filter step: based on objects’ approximations to output the candidate set • Refinement step: comparison of actual objects’ geometric shapes to output the answer set Spatial Database Systems 24 Data Structures, Vector Model (cont'd) • Several indexing methods (a survey in [GG95]) • R-tree family: the most popular ones e.g. R- [Gut84], R+- [SRF87], R*- [BKSS90] etc. • Numerous applications (“trees have grown everywhere” [SRF97]) Multimedia / medical / time-series databases, data warehouses, ... Spatial Database Systems 25 13 Spatial Database Systems Timos Sellis R-Trees An example of R-trees K A F G J B D E H I ABC M DE F G HI J K LMN N L C Spatial Database Systems 26 R-Trees (cont'd) An example of R-trees K A F G J B D E H I ABC range query M point query DE F G HI J K LMN N L C Spatial Database Systems 27 14 Spatial Database Systems Timos Sellis Packed R-trees • Problems with random insertions • Goal: • minimal coverage of leaf nodes • minimal overlap of intermediate nodes • Sorting & packing of spatial objects improves search & space performance by 1-2 orders of magnitude • Starting point for R+-trees, R*-trees, Hilbert R-trees, & Cubetrees VLDB 97 28 R+-trees K A F G P J B D E I H ABC P M DE F G I J K L MN GH L N C May add more levels to the tree .....but it is faster VLDB 97 29 15 Spatial Database Systems Timos Sellis What has been done since 1987? • Lots of other improvements and extensions to the basic structure (R*-tree, Hilbert R-tree, TV-Tree, P- and JP- Tree, and many more) • Commercial systems are incorporating them • Has given rise to lots of interesting other research • More packing algorithms • Spatial joins • Direction queries • Parallelization • Nearest-neighbor queries • Analysis of algorithms and structures VLDB 97 30 Nearest Neighbor Searching downward pruning MINDIST MBR1 MBR2 M11 P M22 M13 M21 NN is there M12 MINMAXDIST VLDB 97 31 16 Spatial Database Systems Timos Sellis Analysis of R-trees • Uniformity assumption • BUT: pessimistic + unrealistic • Solution: FRACTALS - What is the fractal dimension? •≈“intrinsic” dimensionality • Nominal dimension = 2 • “Intrinsic” dimension = 1 VLDB 97 32 Analysis of R-trees Non-integer fractal dimensions • e.g. sierpinski triangle • fractal dimension = log3/log2 = 1.59 VLDB 97 33 17 Spatial Database Systems Timos Sellis Analysis of R-trees Are real data sets fractal? • Coastlines ( fd = 1.1 - 1.58 - e.g. Norway !) • Mamalian brain surface (2.7)
Details
-
File Typepdf
-
Upload Time-
-
Content LanguagesEnglish
-
Upload UserAnonymous/Not logged-in
-
File Pages36 Page
-
File Size-