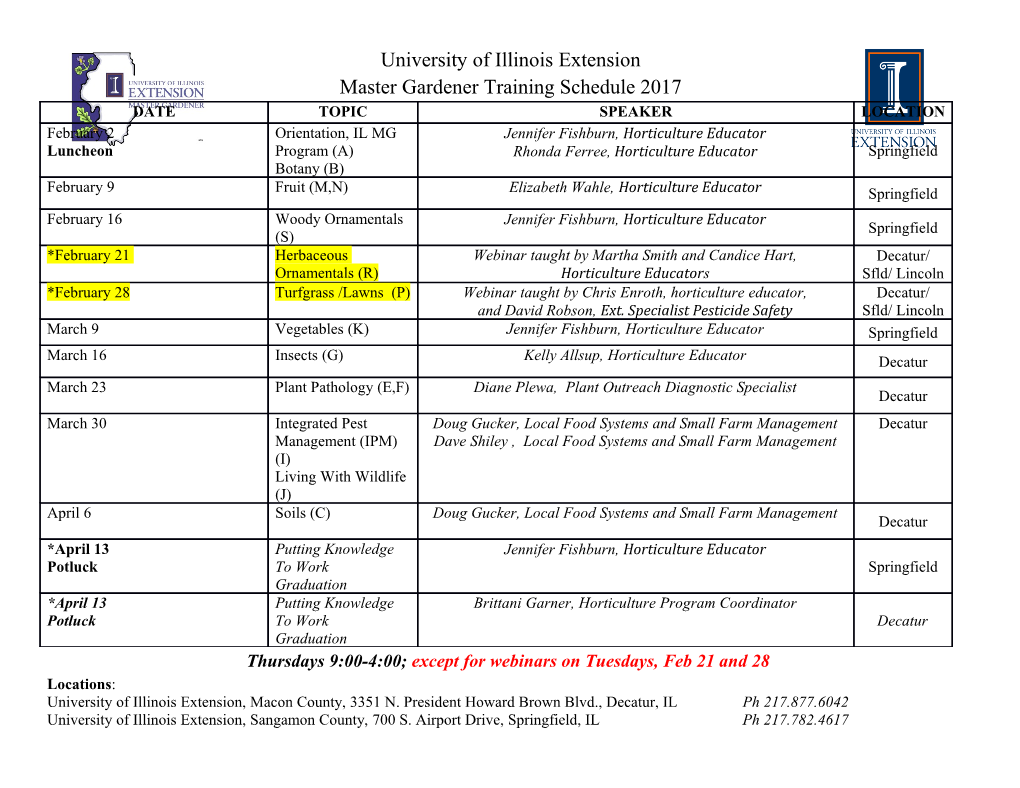
Int. J. Metrol. Qual. Eng. 8, 14 (2017) International Journal of © G.M. Mahmoud and R.S. Hegazy, published by EDP Sciences, 2017 Metrology and Quality Engineering DOI: 10.1051/ijmqe/2017014 Available online at: www.metrology-journal.org RESEARCH ARTICLE Comparison of GUM and Monte Carlo methods for the uncertainty estimation in hardness measurements Gouda M. Mahmoud* and Riham S. Hegazy National Institute of Standards, Tersa St, El-Haram, Box 136 Code 12211, Giza, Egypt Received: 7 January 2017 / Accepted: 2 May 2017 Abstract. Monte Carlo Simulation (MCS) and Expression of Uncertainty in Measurement (GUM) are the most common approaches for uncertainty estimation. In this work MCS and GUM were used to estimate the uncertainty of hardness measurements. It was observed that the resultant uncertainties obtained with the GUM and MCS without correlated inputs for Brinell hardness (HB) were ±0.69 HB, ±0.67 HB and for Vickers hardness (HV) were ±6.7 HV, ±6.5 HV, respectively. The estimated uncertainties with correlated inputs by GUM and MCS were ±0.6 HB, ±0.59 HB and ±6 HV, ±5.8 HV, respectively. GUM overestimate a little bit the MCS estimated uncertainty. This difference is due to the approximation used by the GUM in estimating the uncertainty of the calibration curve obtained by least squares regression. Also the correlations between inputs have significant effects on the estimated uncertainties. Thus the correlation between inputs decreases the contribution of these inputs in the budget uncertainty and hence decreases the resultant uncertainty by about 10%. It was observed that MCS has features to avoid the limitations of GUM. The result analysis showed that MCS has advantages over the traditional method (GUM) in the uncertainty estimation, especially that of complex systems of measurement. MCS is relatively simple to be implemented. Keywords: uncertainty / normal distribution / Monte Carlo / guideline for uncertainty of measurement / correlation 1 Introduction two main limitations. Firstly, the way that the coverage interval is constructed to contain values of the measurand with a stipulated coverage probability is approximate. The guide to the expression of measurement uncertainty fi (GUM, JCGM 100) and the propagation of distributions by Secondly, insuf cient guidance is given for the multivariate a Monte Carlo method (GUMS1, JCGM 101) are two of the case in which there is more than one measurand [3]. Figure 1 most widely used documents concerning measurement shows the schematic to explain the main idea of GUM uncertainty evaluation in metrology. Both documents which mainly depends on the uncertainty propagations [4]. describe three phases: (a) the construction of a measure- Monte Carlo Simulation (MCS) is the second method to ment model, (b) the assignment of probability distribu- estimate the uncertainty of measurements, where evaluat- tions to quantities, and (c) a computational phase that ing the measurement uncertainty by the MCS method can specifies the distribution for the quantity of interest. The be carried out by establishments of the model equation for two approaches described in these two documents agree in the measurand in function of the individual parameters of the first two phases but employ different computational influence, then selecting the significant sources of uncer- approaches, with the GUM using linearization to simplify tainty, identification of the probability density functions the calculations [1]. The guide to the Expression of corresponding to each source of uncertainty selected, and Uncertainty in Measurement (GUM) requires, “that the selecting the number Monte Carlo trials, and finally results of a measurement have been corrected for all calculating the M results by applying the equation that was recognized significant systematic effects and that every defined for the measurand [5]. effort has been made to identify such effects”, before the MCS process is illustrated in Figure 2a and b in issue of evaluating their uncertainty is tackled [2]. GUM comparison with the propagation of uncertainties used by provides a framework and procedure for evaluating and the GUM. expressing measurement uncertainty. GUM procedure has Figure 2a shows an illustration representing the propagation of uncertainties. In this case, three input * Corresponding author: [email protected] quantities are presented x1, x2 and x3 along with their This is an Open Access article distributed under the terms of the Creative Commons Attribution License (http://creativecommons.org/licenses/by/4.0), which permits unrestricted use, distribution, and reproduction in any medium, provided the original work is properly cited. 2 G.M. Mahmoud and R.S. Hegazy: Int. J. Metrol. Qual. Eng. 8, 14 (2017) 2.2 Uncertainty in measurements with and without x1, u (x1) correlation x2, u (x2) f(X) y, u(y) If the functional relationship between the measurand Y and the input quantities X in a measurement process is given x3, u (x3) by [6]; Y ¼ fðX ; X ; X ; ...; X Þ: ð3Þ Fig. 1. GUM and propagation of uncertainties. 1 2 3 N respective uncertainties u(x1), u(x2) and u(x3). As can be The function f is used to calculate the output estimates: y, noted, only the main moments (expectation and standard of the measurand; Y, using the estimates of X1, X2, X3, … , deviation) of the input quantities are used in the XN for the values of the N input quantities. propagation. In Figure 2b, while propagating density (PDFs), no approximations are made and the whole y ¼ fðx1; x2; x3; ...; xN Þ: ð4Þ information contained on the input distributions is propagated to the output [5]. The conventional estimation can be illustrated using a The aims of this present research article are to inves- simple equation with y as a continuous function of x1, x2. y tigate the differences between GUM and MCS as methods is approximated using a polynomial approximation or a of uncertainty estimation of hardness and to detect the 2nd order Taylor’s series expansion about the means: effects of inputs correlation on the uncertainty budget. ∂f ∂f y ¼ fðx1 ; x2 Þþ ðx1 À x1 Þþ ðx2 À x2 ÞþZ; ð5Þ 2 Theoretical principle ∂x1 ∂x2 2.1 Uncertainty classifications where x1 ; x2 are mean values and Z is the remainder: " To investigate the difference between GUM and MCS, the 1 ∂2f ∂2f resultant budget uncertainties of Brinnell and Vickers Z ¼ ðx À x Þ2 þ ðx À x Þ2 2! ∂x 2 1 1 ∂x 2 2 2 hardness (HV) measurements were used as an application. 1 2 # For statistical analysis normal PDFs were proposed. The ∂2f þ 2 ðx À x Þðx À x Þ : ð6Þ uncertainty estimated from normal distribution is believed ∂x ∂x 2 1 1 2 2 to lie in the interval defined by U with a level of confidence 1 2 of approximately 95%. For normal distribution, m is the expectation or mean of the distribution, and the shaded As the partial derivatives are computed at the mean values x1 ; x2 they are the same for all i =1,2,…, N. All the higher areas represent ±1.96 standard uncertainty ustnd about the terms are normally neglected at Z = 0, so equation (5) mean, and ±ustnd, encompasses about 95% of the distribution (see Fig. 3). becomes; Each input source of the uncertainty should be fi ∂f ∂f classi ed as type A or type B where type A evaluation y ¼ fðx1 ; x2 Þþ ðx1 À x1 Þþ ðx2 À x2 Þ: ð7Þ of standard uncertainty is based on any valid statistical ∂x1 ∂x2 method for treating data and can be calculated by the following equations: If f(x1, x2) is a linear function, then the second order partial derivatives in equation (6), are zero, so Z = 0. Both 1 Xn linearity and small uncertainty are prerequisites of x ¼ X ¼ X ; ð1Þ i i n i;k conventional method of uncertainty estimation described k¼1 below. The standard deviations s(X1), s(X2) are referred by GUM [7], as the standard uncertainties associated with "#1=2 the input estimates X , X . The standard uncertainty in y Xn 1 2 1 2 can be obtained by Taylor equation [8]; uðxiÞ¼sðXiÞ¼ ðXi;k À XiÞ ; ð2Þ nðn À 1Þ vffiffiffiffiffiffiffiffiffiffiffiffiffiffiffiffiffiffiffiffiffiffiffiffiffiffiffiffi k¼1 u u 1 XN uðyÞ¼sðyÞ¼t ðy ÀyÞ2 where Xi is an input quantity whose value is estimated from N i n independent observations X of X obtained under the i¼1 i,k i sffiffiffiffiffiffiffiffiffiffiffiffiffiffiffiffiffiffiffiffiffiffiffiffiffiffiffiffiffiffiffiffiffiffiffiffiffiffiffiffiffiffiffiffiffiffiffiffiffiffiffiffiffiffiffiffiffiffiffiffiffiffiffiffiffiffiffiffiffiffiffiffiffiffiffiffiffiffiffiffiffiffiffiffiffiffiffiffiffiffiffiffiffiffiffiffiffiffiffiffi same conditions of measurement, and the standard ∂f 2 ∂f 2 ∂f ∂f uncertainty u(x ) to be associated with x is the estimated ¼ sðx Þ2 þ sðx Þ2 þ2 sðx ;x Þ: i i ∂x 1 ∂x 2 ∂x ∂x 1 2 standard deviation of the mean. For type B standard 1 2 1 2 uncertainty, the evaluation of standard uncertainty is ð8Þ usually based on scientific judgment using all of the relevant information available, that is, the uncertainty is This equation gives the uncertainty as a standard deviation either obtained from an outside source, or obtained from an irrespective of whether or not the measurements of x1, x2 assumed distribution. are independent and of the nature of the probability G.M. Mahmoud and R.S. Hegazy: Int. J. Metrol. Qual. Eng. 8, 14 (2017) 3 sffiffiffiffiffiffiffiffiffiffiffiffiffiffiffiffiffiffiffiffiffiffiffiffiffiffiffiffiffiffiffiffiffiffiffiffiffiffiffiffiffiffiffiffiffiffiffiffiffiffiffiffiffiffiffiffiffiffiffiffiffiffiffiffiffiffiffiffiffiffiffiffiffiffiffiffiffiffiffiffiffiffiffiffiffiffiffiffiffiffiffiffiffiffiffiffiffiffiffiffiffiffiffiffiffiffiffiffiffiffiffiffiffiffiffiffi ∂f 2 ∂f 2 ∂f ∂f uðyÞ¼sðyÞ¼ sðx Þ2 þ sðx Þ2 þ 2 r sðx Þsðx Þ: ð9Þ 1 2 x1;x2 1 2 ∂x1 ∂x2 ∂x1 ∂x2 x1, u (x1) g(x1) y, u(y) g(x ) x2, u (x2) f(X) 2 f(X) x3, u (x3) g(x3) a) Propagation of uncertainties b) Propagation of PDF Fig. 2. Schematic to the main idea of MCS. measurand is believed to lie and is given by: UðyÞ¼kuðyÞ; ð11Þ where k is the coverage factor on the basis of the confidence required for the interval, y ± UðyÞ: ð12Þ If it was considered that y = x1 + x2 is a linear operation it was reported that there is compatibility between MCS and GUM and it was demonstrated that at correlated or uncorrelated inputs MCS gives identical results as given in Fig.
Details
-
File Typepdf
-
Upload Time-
-
Content LanguagesEnglish
-
Upload UserAnonymous/Not logged-in
-
File Pages9 Page
-
File Size-