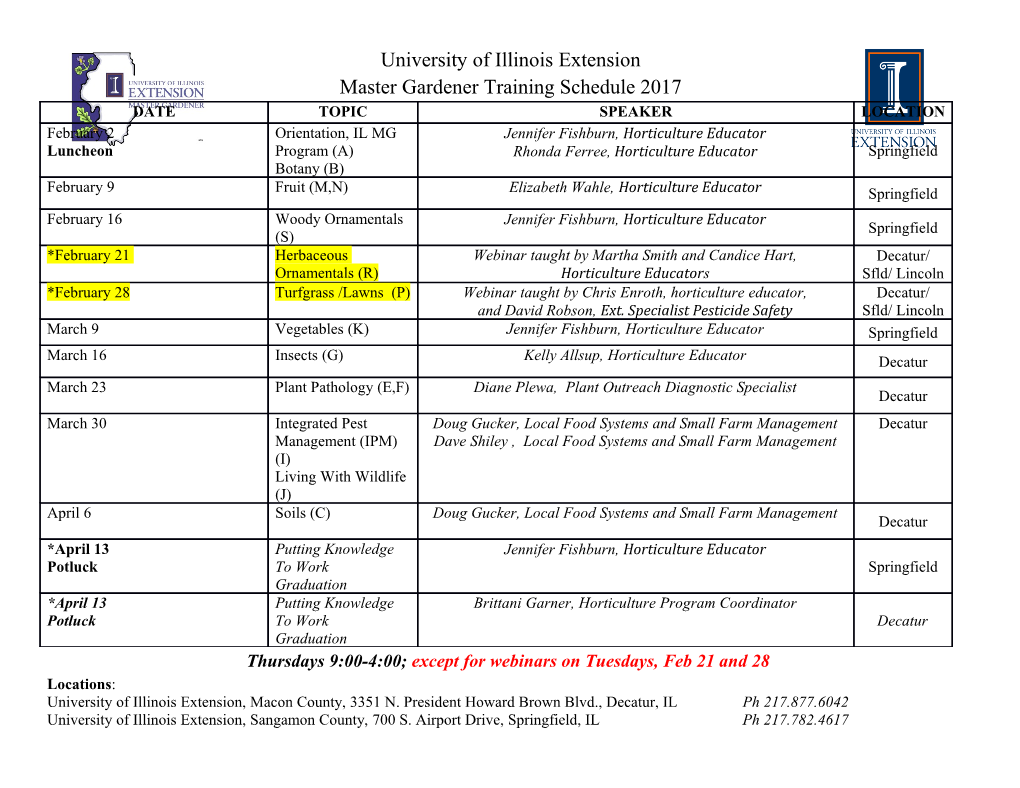
Lecture 9: Epipolar Geometry Professor Fei‐Fei Li Stanford Vision Lab Fei-Fei Li Lecture 9 - 1 21‐Oct‐11 What we will learn today? •Why is stereo useful? • Epipolar constraints • Essential and fundamental matrix •Estimating F (Problem Set 2 (Q2)) • Rectification Reading: [HZ] Chapters: 4, 9, 11 [FP] Chapters: 10 Fei-Fei Li Lecture 9 - 2 21‐Oct‐11 Recovering structure from a single view Pinhole perspective projection P p Ow Scene C Calibration rig Camera K Why is it so difficult? Intrinsic ambiguity of the mapping from 3D to image (2D) Fei-Fei Li Lecture 9 - 3 21‐Oct‐11 Recovering structure from a single view Pinhole perspective projection Lazebnik S. slide Courtesy Intrinsic ambiguity of the mapping from 3D to image (2D) Fei-Fei Li Lecture 9 - 4 21‐Oct‐11 Two eyes help! Fei-Fei Li Lecture 9 - 5 21‐Oct‐11 Two eyes help! ? x1 x2 K =known K =known R, T O2 O1 This is called triangulation Fei-Fei Li Lecture 9 - 6 21‐Oct‐11 Triangulation 2 2 • Find X that minimizes d ( x1 , P1 X ) d ( x 2 , P2 X ) X x2 x1 O1 O2 Fei-Fei Li Lecture 9 - 7 21‐Oct‐11 Stereo‐view geometry • Correspondence: Given a point in one image, how can I find the corresponding point x’ in another one? • Camera geometry: Given corresponding points in two images, find camera matrices, position and pose. • Scene geometry: Find coordinates of 3D point from its projection into 2 or multiple images. This lecture (#9) Fei-Fei Li Lecture 9 - 8 21‐Oct‐11 Stereo‐view geometry Next lecture (#10) • Correspondence: Given a point in one image, how can I find the corresponding point x’ in another one? • Camera geometry: Given corresponding points in two images, find camera matrices, position and pose. • Scene geometry: Find coordinates of 3D point from its projection into 2 or multiple images. Fei-Fei Li Lecture 9 - 9 21‐Oct‐11 What we will learn today? •Why is stereo useful? • Epipolar constraints • Essential and fundamental matrix •Estimating F • Rectification Reading: [HZ] Chapters: 4, 9, 11 [FP] Chapters: 10 Fei-Fei Li Lecture 9 - 10 21‐Oct‐11 Epipolar geometry P p1 p2 e1 e2 O1 O2 • Epipolar Plane • Epipoles e1, e2 • Baseline = intersections of baseline with image planes = projections of the other camera center • Epipolar Lines = vanishing points of camera motion direction Fei-Fei Li Lecture 9 - 11 21‐Oct‐11 Example: Converging image planes Fei-Fei Li Lecture 9 - 12 21‐Oct‐11 Example: Parallel image planes P e2 p 1 p2 e2 O1 O2 • Baseline intersects the image plane at infinity • Epipoles are at infinity • Epipolar lines are parallel to x axis Fei-Fei Li Lecture 9 - 13 21‐Oct‐11 Example: Parallel image planes Fei-Fei Li Lecture 9 - 14 21‐Oct‐11 Example: Forward translation e2 O2 e1 O2 •The epipoles have same position in both images • Epipole called FOE (focus of expansion) Fei-Fei Li Lecture 9 - 15 21‐Oct‐11 Epipolar Constraint ‐ Two views of the same object ‐ Suppose I know the camera positions and camera matrices ‐ Given a point on left image, how can I find the corresponding point on right image? Fei-Fei Li Lecture 9 - 16 21‐Oct‐11 Epipolar Constraint •Potential matches for p have to lie on the corresponding epipolar line l’. •Potential matches for p’ have to lie on the corresponding epipolar line l. Fei-Fei Li Lecture 9 - 17 21‐Oct‐11 Epipolar Constraint P u u p M P v p M P v 1 1 p’ p O R, T O’ M KI 0 M' KR T Fei-Fei Li Lecture 9 - 18 21‐Oct‐11 Epipolar Constraint P p’ p O R, T O’ K1 and K2 are known M KI 0 (calibrated cameras) M' KR T M I 0 M ' R T Fei-Fei Li Lecture 9 - 19 21‐Oct‐11 Epipolar Constraint P p’ p O R, T O’ T (R p) T p T(R p) 0 Perpendicular to epipolar plane Fei-Fei Li Lecture 9 - 20 21‐Oct‐11 Cross product as matrix multiplication 0 az ay bx ab a 0 a b [a ]b z x y ay ax 0 bz “skew symmetric matrix” Fei-Fei Li Lecture 9 - 21 21‐Oct‐11 Triangulation P p’ p O R, T O’ T T p T(R p) 0 p T R p 0 (Longuet‐Higgins, 1981) E = essential matrix Fei-Fei Li Lecture 9 - 22 21‐Oct‐11 Triangulation P p 1 p2 l1 l2 e e1 2 O1 O2 • E p2 is the epipolar line associated with p2 (l1 = E p2) T T • E p1 is the epipolar line associated with p1 (l2 = E p1) • E is singular (rank two) T • E e2 = 0 and E e1 = 0 • E is 3x3 matrix; 5 DOF Fei-Fei Li Lecture 9 - 23 21‐Oct‐11 Triangulation P p’ p O O’ u P M P p M KI 0 v unknown Fei-Fei Li Lecture 9 - 24 21‐Oct‐11 Triangulation P The image part with relationship ID rId8 was not found in the file. p K 1 p p’ p O O’ T 1 T 1 p T R p 0 (K p) T R K p 0 T T 1 pT F p 0 p K T R K p 0 Fei-Fei Li Lecture 9 - 25 21‐Oct‐11 Triangulation P p’ p O O’ pT F p 0 F = Fundamental Matrix (Faugeras and Luong, 1992) Fei-Fei Li Lecture 9 - 26 21‐Oct‐11 Triangulation P p 1 p2 e e1 2 O1 O2 • F p2 is the epipolar line associated with p2 (l1 = F p2) T T • F p1 is the epipolar line associated with p1 (l2 = F p1) • F is singular (rank two) T • F e2 = 0 and F e1 = 0 • F is 3x3 matrix; 7 DOF Fei-Fei Li Lecture 9 - 27 21‐Oct‐11 Why is F useful? T p l’ = F p ‐ Suppose F is known ‐ No additional information about the scene and camera is given ‐ Given a point on left image, how can I find the corresponding point on right image? Fei-Fei Li Lecture 9 - 28 21‐Oct‐11 Why is F useful? •F captures information about the epipolar geometry of 2 views + camera parameters •MORE IMPORTANTLY: F gives constraints on how the scene changes under view point transformation (without reconstructing the scene!) •Powerful tool in: •3D reconstruction •Multi‐view object/scene matching Fei-Fei Li Lecture 9 - 29 21‐Oct‐11 What we will learn today? •Why is stereo useful? • Epipolar constraints • Essential and fundamental matrix •Estimating F (Problem Set 2 (Q2)) • Rectification Reading: [HZ] Chapters: 4, 9, 11 [FP] Chapters: 10 Fei-Fei Li Lecture 9 - 30 21‐Oct‐11 Estimating F The Eight‐Point Algorithm (Longuet‐Higgins, 1981) P (Hartley, 1995) p’ p O O’ u u T P p v P p v p F p 0 1 1 Fei-Fei Li Lecture 9 - 31 21‐Oct‐11 Estimating F pT F p 0 Let’s take 8 corresponding points Fei-Fei Li Lecture 9 - 32 21‐Oct‐11 Estimating F Fei-Fei Li Lecture 9 - 33 21‐Oct‐11 Estimating F W f • Homogeneous system W f 0 •Rank 8 A non‐zero solution exists (unique) •If N>8 Lsq. solution by SVD! ˆ F f 1 Fei-Fei Li Lecture 9 - 34 21‐Oct‐11 Estimating F pT Fˆ p 0 The estimated Fmay^^ have full rank (det(F) ≠0) (F should have rank=2 instead) Find F that minimizes F Fˆ 0 Frobenius norm (*) Subject to det(F)=0 SVD (again!) can be used to solve this problem (*) Sqrt root of the sum pf squares of all entries Fei-Fei Li Lecture 9 - 35 21‐Oct‐11 Example Data courtesy of R. Mohr and B. Boufama. Fei-Fei Li Lecture 9 - 36 21‐Oct‐11 Example Mean errors: 10.0pixel 9.1pixel Fei-Fei Li Lecture 9 - 37 21‐Oct‐11 Normalization Is the accuracy in estimating F function of the ref. system in the image plane? E.g. under similarity transformation (T = scale + translation): qi Ti pi qi Ti pi Does the accuracy in estimating F change if a transformation T is applied? Fei-Fei Li Lecture 9 - 38 21‐Oct‐11 Normalization •The accuracy in estimating F does change if a transformation T is applied •There exists a T for which accuracy is maximized Why? Fei-Fei Li Lecture 9 - 39 21‐Oct‐11 Normalization Lsq solution W f 0, by SVD f 1 F ‐ SVD enforces Rank(W)=8 ‐ Recall the structure of W: Highly un‐balance (not well conditioned) ‐Values of W must have similar magnitude More details HZ pag 108 Fei-Fei Li Lecture 9 - 40 21‐Oct‐11 Normalization IDEA: Transform image coordinate system (T = translation + scaling) such that: •Origin = centroid of image points •Mean square distance of the data points from origin is 2 pixels qi Ti pi qi Ti pi (normalization) Fei-Fei Li Lecture 9 - 41 21‐Oct‐11 The Normalized Eight‐Point Algorithm 0. Compute Ti and Ti’ 1. Normalize coordinates: qi Ti pi qi Ti pi 2. Use the eight‐point algorithm to compute F’q from the points q and q’ . ii T q Fq q 0 3. Enforce the rank‐2 constraint. Fq det( Fq ) 0 T 4. De‐normalize Fq: F T FqT Fei-Fei Li Lecture 9 - 42 21‐Oct‐11 Example Mean errors: 10.0pixel 9.1pixel Without transformation Mean errors: 1.0pixel 0.9pixel With transformation Fei-Fei Li Lecture 9 - 43 21‐Oct‐11 What we will learn today? •Why is stereo useful? • Epipolar constraints • Essential and fundamental matrix •Estimating F • Rectification Reading: [HZ] Chapters: 4, 9, 11 [FP] Chapters: 10 Fei-Fei Li Lecture 9 - 44 21‐Oct‐11 Rectification P p p’ OO’ • Make two camera images “parallel” • Correspondence problem becomes easier Fei-Fei Li Lecture 9 - 45 21‐Oct‐11 Rectification P v v e 2 p p’ e2 y u u z KK O x O • Parallel epipolar lines • Epipoles at infinity Let’s see why….
Details
-
File Typepdf
-
Upload Time-
-
Content LanguagesEnglish
-
Upload UserAnonymous/Not logged-in
-
File Pages67 Page
-
File Size-