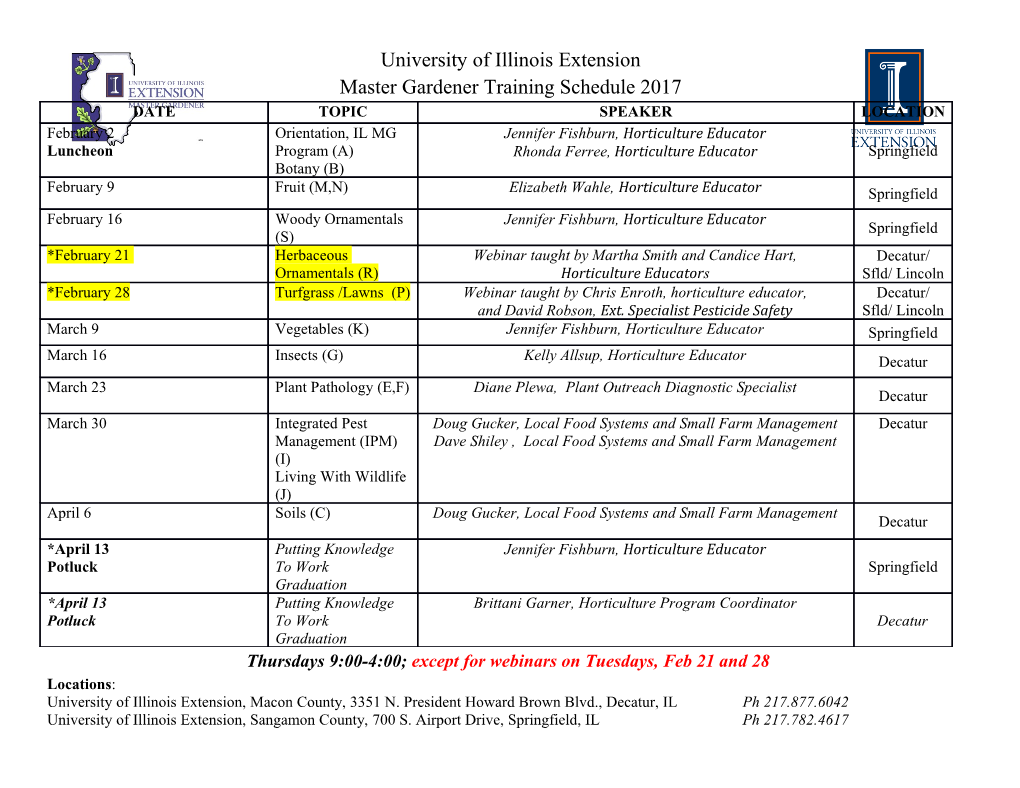
Longitudinal and Panel Data: Analysis and Applications for the Social Sciences by Edward W. Frees Longitudinal and Panel Data: Analysis and Applications for the Social Sciences Brief Table of Contents Chapter 1. Introduction PART I - LINEAR MODELS Chapter 2. Fixed Effects Models Chapter 3. Models with Random Effects Chapter 4. Prediction and Bayesian Inference Chapter 5. Multilevel Models Chapter 6. Random Regressors Chapter 7. Modeling Issues Chapter 8. Dynamic Models PART II - NONLINEAR MODELS Chapter 9. Binary Dependent Variables Chapter 10. Generalized Linear Models Chapter 11. Categorical Dependent Variables and Survival Models Appendix A. Elements of Matrix Algebra Appendix B. Normal Distribution Appendix C. Likelihood-Based Inference Appendix D. Kalman Filter Appendix E. Symbols and Notation Appendix F. Selected Longitudinal and Panel Data Sets Appendix G. References This draft is partially funded by the Fortis Health Insurance Professorship of Actuarial Science. © 2003 by Edward W. Frees. All rights reserved To appear: Cambridge University Press (2004) October, 2003 Longitudinal and Panel Data: Analysis and Applications for the Social Sciences Table of Contents Table of Contents i Preface vi 1. Introduction 1.1 What are longitudinal and panel data? 1-1 1.2 Benefits and drawbacks of longitudinal data 1-4 1.3 Longitudinal data models 1-9 1.4 Historical notes 1-13 PART I - LINEAR MODELS 2. Fixed Effects Models 2.1 Basic fixed effects model 2-1 2.2 Exploring longitudinal data 2-5 2.3 Estimation and inference 2-10 2.4 Model specification and diagnostics 2-14 2.4.1 Pooling test 2-14 2.4.2 Added variable plots 2-15 2.4.3 Influence diagnostics 2-16 2.4.4 Cross-sectional correlation 2-17 2.4.5 Heteroscedasticity 2-18 2.5 Model extensions 2-19 2.5.1 Serial correlation 2-20 2.5.2 Subject-specific slopes 2-21 2.5.3 Robust estimation of standard errors 2-22 Further reading 2-23 Appendix 2A - Least squares estimation 2-24 2A.1 Basic Fixed Effects Model – Ordinary Least Squares Estimation 2-24 2A.2 Fixed Effects Model – Generalized Least Squares Estimation 2-24 2A.3 Diagnostic Statistics 2-25 2A.4 Cross-sectional Correlation 2-26 2. Exercises and Extensions 2-27 3. Models with Random Effects 3.1 Error components / random intercepts model 3-1 3.2 Example: Income tax payments 3-7 3.3 Mixed effects models 3-12 3.3.1 Linear mixed effects model 3-12 3.3.2 Mixed linear model 3-15 3.4 Inference for regression coefficients 3-16 3.5 Variance component estimation 3-20 3.5.1 Maximum likelihood estimation 3-20 3.5.2 Restricted maximum likelihood 3-21 3.5.3 MIVQUE estimators 3-23 Further reading 3-25 Appendix 3A – REML calculations 3-26 3A.1 Independence Of Residuals And Least Squares Estimators 3-26 3A.2 Restricted Likelihoods 3-26 3A.3 Likelihood Ratio Tests And REML 3-28 3. Exercises and Extensions 3-30 4. Prediction and Bayesian Inference 4.1 Prediction for one-way ANOVA models 4-2 4.2 Best linear unbiased predictors (BLUP) 4-4 4.3 Mixed model predictors 4-7 4.3.1 Linear mixed effects model 4-7 4.3.2 Linear combinations of global parameters and subject- specific effects 4-7 4.3.3 BLUP residuals 4-8 4.3.4 Predicting future observations 4-9 4.4 Example: Forecasting Wisconsin lottery sales 4-10 4.4.1 Sources and characteristics of data 4-11 4.4.2 In-sample model specification 4-13 4.4.3 Out-of-sample model specification 4-15 4.4.4 Forecasts 4-16 4.5 Bayesian inference 4-17 4.6 Credibility theory 4-20 4.6.1 Credibility theory models 4-21 4.6.2 Credibility theory ratemaking 4-22 Further reading 4-25 Appendix 4A – Linear unbiased prediction 4-26 4A.1 Minimum Mean Square Predictor 4-26 4A.2 Best Linear Unbiased Predictor 4-26 4A.3 BLUP Variance 4-27 4. Exercises and Extensions 4-28 5. Multilevel Models 5.1 Cross-sectional multilevel models 5-1 5.1.1 Two-level models 5-1 5.1.2 Multiple level models 5-5 5.1.3 Multiple level modeling in other fields 5-6 5.2 Longitudinal multilevel models 5-6 5.2.1 Two-level models 5-6 5.2.2 Multiple level models 5-10 5.3 Prediction 5-11 5.4 Testing variance components 5-13 Further reading 5-15 Appendix 5A – High order multilevel models 5-16 5. Exercises and Extensions 5-19 6. Random Regressors 6.1 Stochastic regressors in non-longitudinal settings 6-1 6.1.1 Endogenous stochastic regressors 6-1 6.1.2 Weak and strong exogeneity 6-3 6.1.3 Causal effects 6-4 6.1.4 Instrumental variable estimation 6-5 6.2 Stochastic regressors in longitudinal settings 6-7 6.2.1 Longitudinal data models without heterogeneity terms 6-7 6.2.2 Longitudinal data models with heterogeneity terms and strictly exogenous regressors 6-8 6.3 Longitudinal data models with heterogeneity terms and 6-11 sequentially exogenous regressors 6.4 Multivariate responses 6-16 6.4.1 Multivariate regressions 6-16 6.4.2 Seemingly unrelated regressions 6-17 6.4.3 Simultaneous equations models 6-18 6.4.4 Systems of equations with error components 6-20 6.5 Simultaneous equation models with latent variables 6-22 6.5.1 Cross-sectional models 6-23 6.5.2 Longitudinal data applications 6-26 Further reading 6-29 Appendix 6A – Linear projections 6-30 7. Modeling Issues 7.1 Heterogeneity 7-1 7.2 Comparing fixed and random effects estimators 7-4 7.2.1 A special case 7-7 7.2.2 General case 7-9 7.3 Omitted variables 7-12 7.3.1 Models of omitted variables 7-13 7.3.2 Augmented regression estimation 7-14 7.4 Sampling, selectivity bias, attrition 7-17 7.4.1 Incomplete and rotating panels 7-17 7.4.2 Unplanned nonresponse 7-18 7.4.3 Non-ignorable missing data 7-21 7. Exercises and Extensions 7-24 8. Dynamic Models 8.1 Introduction 8-1 8.2 Serial correlation models 8-3 8.2.1 Covariance structures 8-3 8.2.2 Nonstationary structures 8-4 8.2.3 Continuous time correlation models 8-5 8.3 Cross-sectional correlations and time-series cross-section 8-7 models 8.4 Time-varying coefficients 8-9 8.4.1 The model 8-9 8.4.2 Estimation 8-10 8.4.4 Forecasting 8-11 8.5 Kalman filter approach 8-13 8.5.1 Transition equations 8-14 8.5.2 Observation set 8-15 8.5.3 Measurement equations 8-15 8.5.4 Initial conditions 8-16 8.5.5 Kalman filter algorithm 8-16 8.6 Example: Capital asset pricing model 8-18 Appendix 8A – Inference for the time-varying coefficient model 8-23 8A.1 The Model 8-23 8A.2 Estimation 8-23 8A.3 Prediction 8-25 PART II - NONLINEAR MODELS 9. Binary Dependent Variables 9.1 Homogeneous models 9-1 9.1.1 Logistic and probit regression models 9-2 9.1.2 Inference for logistic and probit regression models 9-5 9.1.3 Example: Income tax payments and tax preparers 9-7 9.2 Random effects models 9-9 9.3 Fixed effects models 9-13 9.4 Marginal models and GEE 9-16 Further reading 9-20 Appendix 9A – Likelihood calculations 9-20 9A.1 Consistency Of Likelihood Estimators 9-21 9A.2 Computing Conditional Maximum Likelihood Estimators 9-22 9. Exercises and Extensions 9-23 10. Generalized Linear Models 10.1 Homogeneous models 10-1 10.1.1 Linear exponential families of distributions 10-1 10.1.2 Link functions 10-2 10.1.3 Estimation 10-3 10.2 Example: Tort filings 10-5 10.3 Marginal models and GEE 10-8 10.4 Random effects models 10-12 10.5 Fixed effects models 10-16 10.5.1 Maximum likelihood estimation for canonical links 10-16 10.5.2 Conditional maximum likelihood estimation for canonical links 10-17 10.5.3 Poisson distribution 10-18 10.6 Bayesian inference 10-19 Further reading 10-22 Appendix 10A – Exponential families of distributions 10-23 10A.1 Moment Generating Functions 10-23 10A.2 Sufficiency 10-24 10A.3 Conjugate Distributions 10-24 10A.4 Marginal Distributions 10-25 10. Exercises and Extensions 10-27 11. Categorical Dependent Variables and Survival Models 11.1 Homogeneous models 11-1 11.1.1 Statistical inference 11-2 11.1.2 Generalized logit 11-2 11.1.3 Multinomial (conditional) logit 11-4 11.1.4 Random utility interpretation 11-6 11.1.5 Nested logit 11-7 11.1.6 Generalized extreme value distribution 11-8 11.2 Multinomial logit models with random effects 11-8 11.3 Transition (Markov) models 11-10 11.4 Survival models 11-18 Appendix 11A. Conditional likelihood estimation for 11-21 multinomial logit models with random effects APPENDICES Appendix A. Elements of Matrix Algebra A-1 A.1 Basic Definitions A-1 A.2 Basic Operations A-1 A.3 Further Definitions A-2 A.4 Matrix Decompositions A-2 A.5 Partitioned Matrices A-3 A.6 Kronecker (Direct) Products A-4 Appendix B. Normal Distribution A-5 Appendix C. Likelihood-Based Inference A-6 C.1 Characteristics of Likelihood Functions A-6 C.2 Maximum Likelihood Estimators A-6 C.3 Iterated Reweighted Least Squares A-8 C.4 Profile Likelihood A-8 C.5 Quasi-Likelihood A-8 C.6 Estimating Equations A-9 C.7 Hypothesis Tests A-11 C.8 Information Criteria A-12 C.9 Goodness of Fit Statistics A-13 Appendix D.
Details
-
File Typepdf
-
Upload Time-
-
Content LanguagesEnglish
-
Upload UserAnonymous/Not logged-in
-
File Pages364 Page
-
File Size-