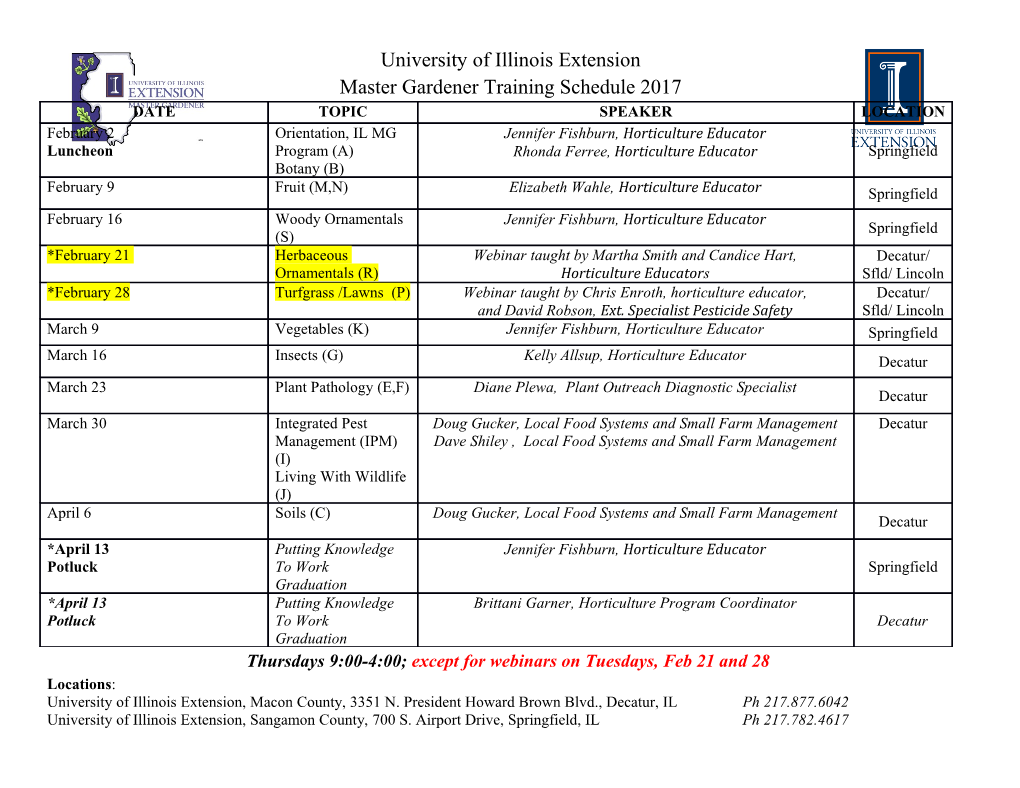
Recap Bayes Risk Consistency Summary Recap Bayes Risk Consistency Summary . Last Lecture . Biostatistics 602 - Statistical Inference Lecture 16 • What is a Bayes Estimator? Evaluation of Bayes Estimator • Is a Bayes Estimator the best unbiased estimator? . • Compared to other estimators, what are advantages of Bayes Estimator? Hyun Min Kang • What is conjugate family? • What are the conjugate families of Binomial, Poisson, and Normal distribution? March 14th, 2013 Hyun Min Kang Biostatistics 602 - Lecture 16 March 14th, 2013 1 / 28 Hyun Min Kang Biostatistics 602 - Lecture 16 March 14th, 2013 2 / 28 Recap Bayes Risk Consistency Summary Recap Bayes Risk Consistency Summary . Recap - Bayes Estimator Recap - Example • θ : parameter • π(θ) : prior distribution i.i.d. • X1, , Xn Bernoulli(p) • X θ fX(x θ) : sampling distribution ··· ∼ | ∼ | • π(p) Beta(α, β) • Posterior distribution of θ x ∼ | • α Prior guess : pˆ = α+β . Joint fX(x θ)π(θ) π(θ x) = = | • Posterior distribution : π(p x) Beta( xi + α, n xi + β) | Marginal m(x) | ∼ − • Bayes estimator ∑ ∑ m(x) = f(x θ)π(θ)dθ (Bayes’ rule) | α + x x n α α + β ∫ pˆ = i = i + α + β + n n α + β + n α + β α + β + n • Bayes Estimator of θ is ∑ ∑ E(θ x) = θπ(θ x)dθ | θ Ω | ∫ ∈ Hyun Min Kang Biostatistics 602 - Lecture 16 March 14th, 2013 3 / 28 Hyun Min Kang Biostatistics 602 - Lecture 16 March 14th, 2013 4 / 28 Recap Bayes Risk Consistency Summary Recap Bayes Risk Consistency Summary . Loss Function Optimality Loss Function Let L(θ, θˆ) be a function of θ and θˆ. • Squared error loss L(θ,ˆ θ) = (θˆ θ)2 The mean squared error (MSE) is defined as − MSE = Average Loss = E[L(θ, θˆ)] MSE(θˆ) = E[θˆ θ]2 − which is the expectation of the loss if θˆ is used to estimate θ. ˆ Let θ is an estimator. • Absolute error loss • If θˆ = θ, it makes a correct decision and loss is 0 L ˆ ˆ • If θˆ = θ, it makes a mistake and loss is not 0. (θ) = θ θ ̸ | − | • A loss that penalties overestimation more than underestimation L(θ, θˆ) = (θˆ θ)2I(θˆ < θ) + 10(θˆ θ)2I(θˆ θ) − − ≥ Hyun Min Kang Biostatistics 602 - Lecture 16 March 14th, 2013 5 / 28 Hyun Min Kang Biostatistics 602 - Lecture 16 March 14th, 2013 6 / 28 Recap Bayes Risk Consistency Summary Recap Bayes Risk Consistency Summary . Risk Function - Average Loss Alternative definition of Bayes Risk R(θ, θˆ) = E[L(θ, θˆ(X)) θ] | R(θ, θˆ)π(θ)dθ = E[L(θ, θˆ(X))]π(θ)dθ If L(θ, θˆ) = (θˆ θ)2, R(θ, θˆ) is MSE. ∫Ω ∫Ω − An estimator with smaller R(θ, θˆ) is preferred. = f(x θ)L(θ, θˆ(x))dx π(θ)dθ | . ∫Ω [∫ ] Definition : Bayes Risk X . = f(x θ)L(θ, θˆ(x))π(θ)dx dθ Bayes risk is defined as the average risk across all values of θ given prior Ω | ∫ [∫X ] π(θ) = π(θ x)m(x)L(θ, θˆ(x))dx dθ R(θ, θˆ)π(θ)dθ Ω | ∫ [∫X ] . ∫Ω ˆ The Bayes rule with respect to a prior π is the optimal estimator with = L(θ, θ(X))π(θ x)dθ m(x)dx Ω | respect to a Bayes risk, which is defined as the one that minimize the ∫X [∫ ] Bayes risk. Hyun Min Kang Biostatistics 602 - Lecture 16 March 14th, 2013 7 / 28 Hyun Min Kang Biostatistics 602 - Lecture 16 March 14th, 2013 8 / 28 Recap Bayes Risk Consistency Summary Recap Bayes Risk Consistency Summary . Posterior Expected Loss Bayes Estimator based on squared error loss L(θ,ˆ θ) = (θˆ θ)2 − Posterior expected loss = (θ θˆ)2π(θ x)dθ Posterior expected loss is defined as − | ∫Ω = E[(θ θˆ)2 X = x] π(θ x)L(θ, θˆ(x))dθ − | Ω | So, the goal is to minimize E[(θ θˆ)2 X = x] ∫ − | An alternative definition of Bayes rule estimator is the estimator that E (θ θˆ)2 X = x = E (θ E(θ x) + E(θ x) θˆ)2 X = x minimizes the posterior expected loss. − | − | | − | [ ] [ ] = E (θ E(θ x))2 X = x + E (E(θ x) θˆ)2 X = x − | | | − | [ 2 ] = E [(θ E(θ x))2 X = x] + E(θ x) θˆ − | | | − [ ] which is minimized when θˆ =[ E(θ x). ] | Hyun Min Kang Biostatistics 602 - Lecture 16 March 14th, 2013 9 / 28 Hyun Min Kang Biostatistics 602 - Lecture 16 March 14th, 2013 10 / 28 Recap Bayes Risk Consistency Summary Recap Bayes Risk Consistency Summary . Summary so far Bayes Estimator based on absolute error loss Loss function L(θ, θˆ) ˆ ˆ • e.g. (θˆ θ)2, θˆ θ Suppose that L(θ, θ) = θ θ . The posterior expected loss is − | − | | − | Risk function R(θ, θˆ) is average of L(θ, θˆ) across all x ∈ X E[L(θ, θˆ(x))] = θ θˆ(x) π(θ x)dθ • For squared error loss, risk function is the same to MSE. | − | | ∫Ω ˆ Bayes risk Average risk across all θ, based on the prior of θ. = E[ θ θ X = x] |ˆ − || • Alternatively, average posterior error loss across all θ = (θ θˆ)π(θ x)dθ + ∞(θ θˆ)π(θ x)dθ x . − − | θˆ − | ∈ X ∫−∞ ∫ Bayes estimator θˆ = E[θ x]. Based on squared error loss, | ∂ E[L(θ, θˆ(x))] = 0, and θˆ is posterior median. • Minimize Bayes risk ∂θˆ • Minimize Posterior Expected Loss Hyun Min Kang Biostatistics 602 - Lecture 16 March 14th, 2013 11 / 28 Hyun Min Kang Biostatistics 602 - Lecture 16 March 14th, 2013 12 / 28 Recap Bayes Risk Consistency Summary Recap Bayes Risk Consistency Summary . Two Bayes Rules Example i.i.d. • X , , Xn Bernoulli(p). 1 ··· ∼ Consider a point estimation problem for real-valued parameter θ. • π(p) Beta(α, β) . ∼ • The posterior distribution follows Beta( xi + α, n xi + β). For squared error loss, the posterior expected loss is − • (θ θˆ)2π(θ x)dθ = E[(θ θˆ)2 X = x] Bayes estimator that minimizes posterior∑ expected squared∑ error loss − | − | is the posterior mean ∫Ω xi + α ˆ pˆ = This expected value is minimized by θ = E(θ x). So the Bayes rule α + β + n | ∑ .estimator is the mean of the posterior distribution. • Bayes estimator that minimizes posterior expected absolute error loss For absolute error loss, the posterior expected loss is E( θ θˆ X = x). As is the posterior median ˆ | − || .shown previously, this is minimized by choosing θ as the median of π(θ x). | θˆ n Γ(α + β + ) xi+α 1 n xi+β 1 1 p − (1 p) − − dp = Γ( xi + α)Γ(n xi + β) − 2 ∫0 − ∑ ∑ ∑ ∑ Hyun Min Kang Biostatistics 602 - Lecture 16 March 14th, 2013 13 / 28 Hyun Min Kang Biostatistics 602 - Lecture 16 March 14th, 2013 14 / 28 Recap Bayes Risk Consistency Summary Recap Bayes Risk Consistency Summary . Asymptotic Evaluation of Point Estimators Tools for proving consistency When the sample size n approaches infinity, the behaviors of an estimator are unknown as its asymptotic properties. Definition - Consistency • Use definition (complicated) . Let Wn = Wn(X , , Xn) = Wn(X) be a sequence of estimators for • Chebychev’s Inequality 1 ··· . We say W is consistent for estimating if W P under τ(θ) n τ(θ) n τ(θ) 2 2 → Pr( Wn τ(θ) ϵ) = Pr((Wn τ(θ)) ϵ ) .Pθ for every θ Ω. | − | ≥ − ≥ ∈ E W 2 P [ n τ(θ)] Wn τ(θ) (converges in probability to τ(θ)) means that, given any −2 → ≤ ϵ ϵ > 0. 2 MSE(Wn) Bias (Wn) + Var(Wn) = 2 = 2 lim Pr( Wn τ(θ) ϵ) = 0 ϵ ϵ n →∞ | − | ≥ lim Pr( Wn τ(θ) < ϵ) = 1 Need to show that both Bias(Wn) and Var(Wn) converges to zero n →∞ | − | When Wn τ(θ) < ϵ can also be represented that Wn is close to τ(θ). | − | Consistency implies that the probability of Wn close to τ(θ) approaches to 1 as n goes to . Hyun Min Kang∞ Biostatistics 602 - Lecture 16 March 14th, 2013 15 / 28 Hyun Min Kang Biostatistics 602 - Lecture 16 March 14th, 2013 16 / 28 Recap Bayes Risk Consistency Summary Recap Bayes Risk Consistency Summary . Theorem for consistency Weak Law of Large Numbers . Theorem 10.1.3 . Theorem 5.5.2 If Wn is a sequence of estimators of τ(θ) satisfying . Let X1, , Xn be iid random variables with E(X) = µ and • limn > Bias(Wn) = 0. ··· 2 Var(X) = σ < . Then Xn converges in probability to µ. − ∞ ∞ • limn > Var(Wn) = 0. P − ∞ .i.e. Xn µ. → .for all θ, then Wn is consistent for τ(θ) Hyun Min Kang Biostatistics 602 - Lecture 16 March 14th, 2013 17 / 28 Hyun Min Kang Biostatistics 602 - Lecture 16 March 14th, 2013 18 / 28 Recap Bayes Risk Consistency Summary Recap Bayes Risk Consistency Summary . Consistent sequence of estimators Example . Theorem 10.1.5 . Let Wn is a consistent sequence of estimators of τ(θ). Let an, bn be Problem sequences of constants satisfying . X1, , Xn are iid samples from a distribution with mean µ and variance 1. limn an = 1 2 ··· →∞ σ < . 2. limn bn = 0. ∞ →∞ 1. Show that Xn is consistent for µ. Then Un = anWn + bn is also a consistent sequence of estimators of τ(θ). 1 n 2 2 . 2. Show that (Xi X) is consistent for σ . n i=1 − 1 n 2 2 . 3. Show that (Xi X) is consistent for σ . Continuous Map Theorem . n ∑1 i=1 − . − If Wn is consistent for θ and g is a continuous function, then g(Wn) is ∑ .consistent for g(θ).
Details
-
File Typepdf
-
Upload Time-
-
Content LanguagesEnglish
-
Upload UserAnonymous/Not logged-in
-
File Pages7 Page
-
File Size-