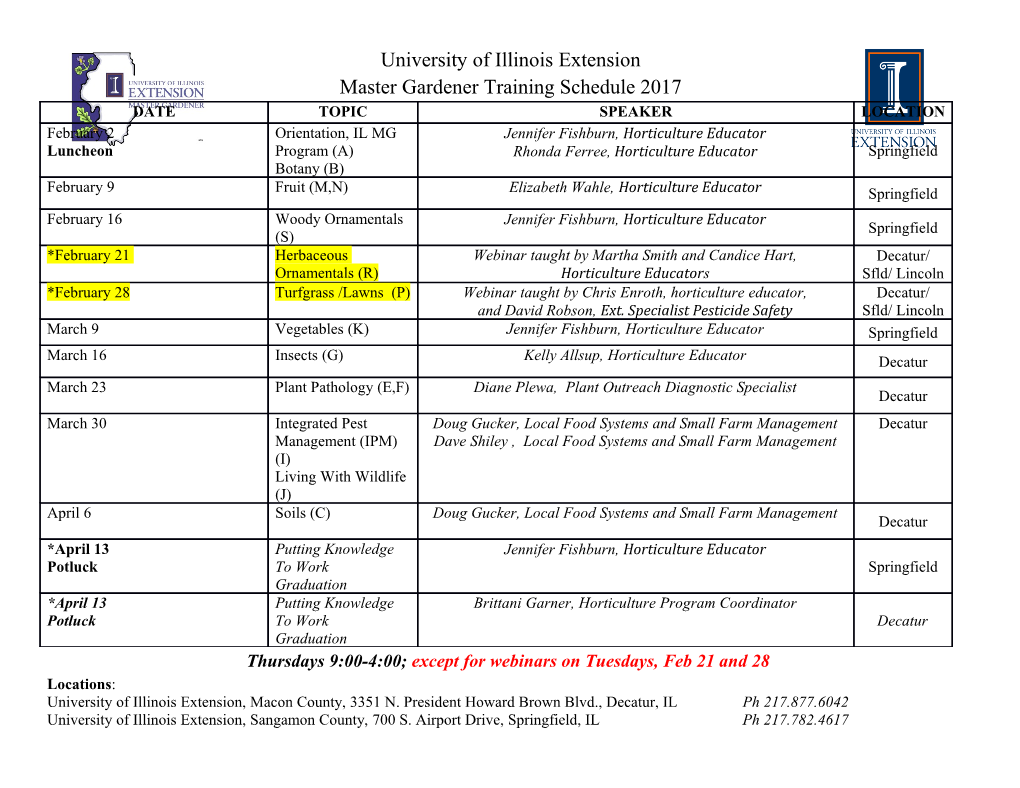
Sparse Optimization Lecture: Sparse Recovery Guarantees Instructor: Wotao Yin July 2013 Note scriber: Zheng Sun online discussions on piazza.com Those who complete this lecture will know • how to read different recovery guarantees • some well-known conditions for exact and stable recovery such as Spark, coherence, RIP, NSP, etc. • indeed, we can trust `1-minimization for recovering sparse vectors 1 / 35 The basic question of sparse optimization is: Can I trust my model to return an intended sparse quantity? That is • does my model have a unique solution? (otherwise, different algorithms may return different answers) • is the solution exactly equal to the original sparse quantity? • if not (due to noise), is the solution a faithful approximate of it? • how much effort is needed to numerically solve the model? This lecture provides brief answers to the first three questions. 2 / 35 What this lecture does and does not cover It covers basic sparse vector recovery guarantees based on • spark • coherence • restricted isometry property (RIP) and null-space property (NSP) as well as both exact and robust recovery guarantees. It does not cover the recovery of matrices, subspaces, etc. Recovery guarantees are important parts of sparse optimization, but they are not the focus of this summer course. 3 / 35 Examples of guarantees Theorem (Donoho and Elad [2003], Gribonval and Nielsen [2003]) m×n For Ax = b where A 2 R has full rank, if x satisfies 1 −1 kxk0 ≤ 2 (1 + µ(A) ), then `1-minimization recovers this x. Theorem (Candes and Tao [2005]) If x is k-sparse and A satisfies the RIP-based condition δ2k + δ3k < 1, then x is the `1-minimizer. Theorem (Zhang [2008]) m×n IF A 2 R is a standard Gaussian matrix, then with probability at least 1 − exp(−c0(n − m)) `1-minimization is equivalent to `0-minimization for all x: c2 m kxk < 1 0 4 1 + log(n=m) where c0; c1 > 0 are constants independent of m and n. 4 / 35 How to read guarantees Some basic aspects that distinguish different types of guarantees: • Recoverability (exact) vs stability (inexact) • General A or special A? • Universal (all sparse vectors) or instance (certain sparse vector(s))? • General optimality? or specific to model / algorithm? • Required property of A: spark, RIP, coherence, NSP, dual certificate? • If randomness is involved, what is its role? • Condition/bound is tight or not? Absolute or in order of magnitude? 5 / 35 Spark First questions for finding the sparsest solution to Ax = b 1. Can sparsest solution be unique? Under what conditions? 2. Given a sparse x, how to verify whether it is actually the sparsest one? Definition (Donoho and Elad [2003]) The spark of a given matrix A is the smallest number of columns from A that are linearly dependent, written as spark(A). rank(A) is the largest number of columns from A that are linearly independent. In general, spark(A) 6= rank(A) + 1; except for many randomly generated matrices. Rank is easy to compute (due to the matroid structure), but spark needs a combinatorial search. 6 / 35 Spark Theorem (Gorodnitsky and Rao [1997]) If Ax = b has a solution x obeying kxk0 < spark(A)=2, then x is the sparsest solution. • Proof idea: if there is a solution y to Ax = b and x − y 6= 0, then A(x − y) = 0 and thus kxk0 + kyk0 ≥ kx − yk0 ≥ spark(A) or kyk0 ≥ spark(A) − kxk0 > spark(A)=2 > kxk0. • The result does not mean this x can be efficiently found numerically. m×n • For many random matrices A 2 R , the result means that if an algorithm returns x satisfying kxk0 < (m + 1)=2, the x is optimal with probability 1. • What to do when spark(A) is difficult to obtain? 7 / 35 General Recovery - Spark Rank is easy to compute, but spark needs a combinatorial search. However, for matrix with entries in general positions, spark(A) = rank(A) + 1. m×n For example, if matrix A 2 R (m < n) has entries Aij ∼ N (0; 1), then rank(A) = m = spark(A) − 1 with probability 1. m×n In general, 8 full rank matrix A 2 R (m < n), any m + 1 columns of A is linearly dependent, so spark(A) ≤ m + 1 = rank(A) + 1: 8 / 35 Coherence Definition (Mallat and Zhang [1993]) The (mutual) coherence of a given matrix A is the largest absolute normalized inner product between different columns from A. Suppose A = [a1 a2 ··· an]. The mutual coherence of A is given by ja>a j µ(A) = max k j : k;j;k6=j kakk2 · kaj k2 • It characterizes the dependence between columns of A • For unitary matrices, µ(A) = 0 • For matrices with more columns than rows, µ(A) > 0 • For recovery problems, we desire a small µ(A) as it is similar to unitary matrices. • For A = [Φ Ψ] where Φ and Ψ are n × n unitary, it holds n−1=2 ≤ µ(A) ≤ 1 • µ(A) = n−1=2 is achieved with [I F], [I Hadamard], etc. m×n −1=2 • if A 2 R where n > m, then µ(A) ≥ m . 9 / 35 Coherence Theorem (Donoho and Elad [2003]) spark(A) ≥ 1 + µ−1(A): Proof sketch: • A¯ A with columns normalized to unit 2-norm • p spark(A) • B a p × p minor of A¯ >A¯ P • jBiij = 1 and j6=i jBij j ≤ (p − 1)µ(A) −1 P • Suppose p < 1 + µ (A) ) 1 > (p − 1)µ(A) ) jBiij > j6=i jBij j; 8i • ) B 0 (Gershgorin circle theorem) ) spark(A) > p. Contradiction. 10 / 35 Coherence-base guarantee Corollary −1 If Ax = b has a solution x obeying kxk0 < (1 + µ (A))=2, then x is the unique sparsest solution. Compare with the previous Theorem If Ax = b has a solution x obeying kxk0 < spark(A)=2, then x is the sparsest solution. m×n −1 p For A 2 R where m < n, (1 + µ (A)) is at most 1 + m but spark can be 1 + m. spark is more useful. Assume Ax = b has a solution with kxk0 = k < spark(A)=2. It will be the unique `0 minimizer. Will it be the `1 minimizer as well? Not necessarily. −1 However, kxk0 < (1 + µ (A))=2 is a sufficient condition. 11 / 35 Coherence-based `0 = `1 Theorem (Donoho and Elad [2003], Gribonval and Nielsen [2003]) If A has normalized columns and Ax = b has a solution x satisfying 1 kxk < 1 + µ−1(A) ; 0 2 then this x is the unique minimizer with respect to both `0 and `1. Proof sketch: • Previously we know x is the unique `0 minimizer; let S := supp(x) • Suppose y is the `1 minimizer but not x; we study e := y − x • e must satisfy Ae = 0 and kek1 ≤ 2keS k1 > −1 • A Ae = 0 ) jej j ≤ (1 + µ(A)) µ(A)kek1; 8j • the last two points together contradict the assumption p Result bottom line: allow kxk0 up to O( m) for exact recovery 12 / 35 The null space of A P p1=p • Definition: kxkp := i jxij . • Lemma: Let 0 < p ≤ 1. If k(y − x)S¯kp > k(y − x)S kp then kxkp < kykp. Proof: Let e := y − x. p p p p kykp = kx + ekp = kxS + eS kp + keS¯kp = p p p p p p kxkp + (keS¯kp − keS kp) + (kxS + eS kp − kxS kp + keS kp). Last term is nonnegative for 0 < p ≤ 1. p p So, a sufficient condition is keS¯kp > keS kp. • If the condition holds for 0 < p ≤ 1, it also holds for q 2 (0; p]. • Definition (null space property NSP(k; γ)). Every nonzero e 2 N (A) satisfies keS k1 < γkeS¯k1 for all index sets S with jSj ≤ k. 13 / 35 The null space of A Theorem (Donoho and Huo [2001], Gribonval and Nielsen [2003]) o Basis pursuit minfkxk1 : Ax = bg uniquely recovers all k-sparse vectors x from measurements b = Axo if and only if A satisfies NSP(k; 1). Proof. Sufficiency. Pick any k-sparse vector xo. Let S := supp(xo) and S¯ = Sc. For any non-zero h 2 N (A), we have A(xo + h) = Axo = b and 0 0 kx + hk1 = kxS + hS k1 + khS¯k1 0 ≥ kxS k1 − khS k1 + khS¯k1 (1) 0 = kx k1 + (khS¯k1 − khS k1) : 0 0 o NSP(k; 1) of A guarantees kx + hk1 > kx k1, so x is the unique solution. o Necessity. The inequality (1) holds with equality if sign(xS ) = −sign(hS ) and hS has a sufficiently small scale. Therefore, basis pursuit to uniquely recovers all k-sparse vectors xo, NSP(k; 1) is also necessary. 14 / 35 The null space of A • Another sufficient condition (Zhang [2008]) for kxk1 < kyk1 is 2 1 ky − xk1 kxk0 < : 4 ky − xk2 Proof: p p p keS k1 ≤ jSjkeS k2 ≤ jSjkek2 = kxk0kek2: Then, the above sufficient condition ky − xk1 > 2k(y − x)S k1 is given the above inequality. 15 / 35 Null space Theorem (Zhang [2008]) Given x and b = Ax, min kxk1 s.t. Ax = b recovers x uniquely if 2 1 kek1 kxk0 < min 2 : e 2 N (A) n f0g : 4 kek2 Comments: p • We know 1 ≤ kek1=kek2 ≤ n for all e 6= 0. The ratio is small for sparse p vectors but we want it large, i.e., close to n and away from 1. • Fact: in most subspaces, the ratio is away from 1 • In particular, Kashin, Garvaev, and Gluskin showed that a randomly drawn (n − m)-dimensional subspace V satisfies p kek1 c1 m ≥ p ; e 2 V; e 6= 0 kek2 1 + log(n=m) with probability at least 1 − exp(−c0(n − m)), where c0; c1 > 0 are independent of m and n.
Details
-
File Typepdf
-
Upload Time-
-
Content LanguagesEnglish
-
Upload UserAnonymous/Not logged-in
-
File Pages35 Page
-
File Size-