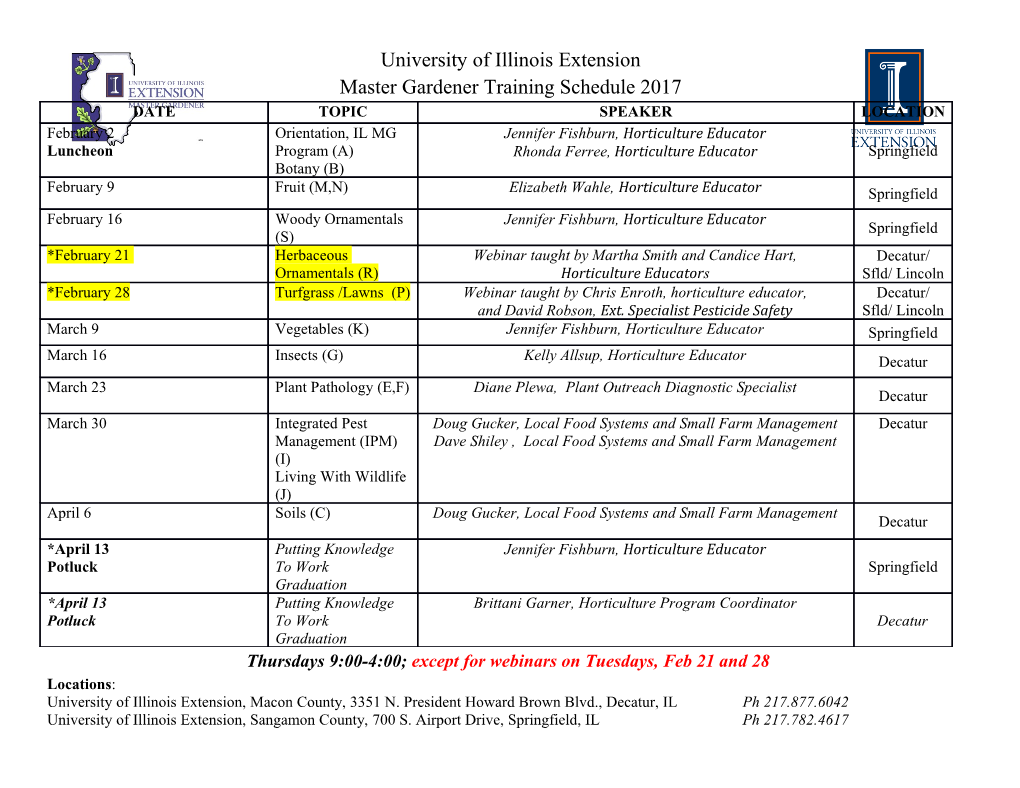
CHAPTER 3 Ecological informatics: current scope and feature areas F. Recknagel School of Earth and Environmental Sciences, University of Adelaide, Adelaide, Australia. 1 Introduction Ecological informatics (ecoinformatics) is an interdisciplinary framework for the management, analysis and synthesis of ecological data by advanced computational technology [1]. Manage- ment of ecological data aims at facilitating data standardization, retrieval and sharing by means of metadata and object-oriented programming [2–6]. Analysis and synthesis of ecological data aim at elucidating principles of information processing, structuring and functioning of ecosys- tems, and forecasting of ecosystem behaviours by means of bio-inspired computation and hybrid models [1, 7–10]. Ecological informatics currently undergoes the process of consolidation as a discipline. It corresponds to and partially overlaps with the well-established disciplines of bioinformatics and ecological modelling, but is taking its distinct shape and scope. In Fig. 1, a comparison is made between ecological informatics and bioinformatics. Even though both are based on the same computational technology, their focus is different. Bioinformatics focuses very much on determining gene function and interaction [11, 12], protein structure and function [13, 14] as well as phenotypes of organisms utilizing DNA microarray, genomic, physiological and meta- bolic data [15] (Fig. 1a). In contrast, ecological informatics focuses on determining genotypes of populations by utilizing genomic, phenotypic and environmental data [16–18] as well as structure and functioning of ecosystems by utilizing community, environmental and climate data [19–23] (Fig. 1b). A comparison is made between ecological modelling and ecological informatics in Fig. 2. Even though both rely on similar ecological data, they adopt different approaches in utilizing the data. Whilst ecological modelling processes ecological data top down by ad hoc designed statis- tical or mathematical modelling methods [24, 25], ecological informatics infers multivariate equations and rule sets from ecological data patterns bottom up by computational techniques. The cross-sectional area between ecological modelling and ecological informatics refl ects a new generation of hybrid models that enable to predict emergent ecosystem structures and behav- iours, and ecosystem evolution [9, 26–30]. Typically hybrid models embody biologically-inspired computation in deterministic ecological models [32]. WIT Transactions on State of the Art in Science and Engineering, Vol 34, © 2009 WIT Press www.witpress.com, ISSN 1755-8336 (on-line) doi:10.2495/978-1-84564-207-5/03 42 Handbook of Ecological Modelling and Informatics a. DNA Microarray Data ORGANISM Gene Function and Interaction Genomic Sequence Data ORGANS Protein Structure and Function CELLS Physiological Data Phenotypes of Organisms GENES Metabolic Data b. Genomic Sequence Data AUTOTROPHIC PRODUCERS Competition / Mutualism HETEROTROPHIC CONSUMERS Phenotypic Competition Data Competition Competition Predation / M > Genotypes of / Mutualis utua m Populations Competition lism / Mutualism Decomposition Competition Community / Mutualism Data > Predation > Structure and Functioning of Ecosystems Environmental DECOMPOSERS < Decomposition Data on Competition / Mutualism Competiti / Mutualism Competition Climate Data / Mutualism Figure 1: Ecological informatics versus bioinformatics. (a) Scope of bioinformatics (modifi ed from Oltavai and Barabasi [31]), (b) Scope of ecoinformatics. WIT Transactions on State of the Art in Science and Engineering, Vol 34, © 2009 WIT Press www.witpress.com, ISSN 1755-8336 (on-line) Ecological Informatics: Current Scope and Feature Areas 43 Ecological Modelling Ecological Informatics Differential Equations High Performance Computing Thermodynamics Biologically-Inspired Computation Multivariate Statistics Object-Oriented Data Warehousing Heuristics Metadata Concepts Semantic Webs GIS Hybrid Approach Top-Down Bottom-Up Mathematical Computational Approach Approach e.g. Statistical, e.g. Neural, Cellular, Process-based Evolutionary Ecology Biosphere Ecosystems Communities Populations Organisms Cells Genes Figure 2: Ecological informatics vs. ecological modelling. 2 Feature areas Current research in ecological informatics focuses on four major feature areas: understanding information processing and evolution in ecosystems, computational management of ecological data, computational analysis and synthesis of ecological data and hybrid modelling of ecological data. Great efforts are undertaken to address feature area (1) by studying both intraspecifi c popula- tion adaptations to changing climate and habitat conditions [33–36] as well as interspecifi c pop- ulation relationships controlled by info-chemicals and allelopathy [37–41]. Feature area (2) aims at standardised archiving of highly complex and fragmented eco- logical data to allow ecological data sharing. The ecological metadata language EML (http://knb. WIT Transactions on State of the Art in Science and Engineering, Vol 34, © 2009 WIT Press www.witpress.com, ISSN 1755-8336 (on-line) 44 Handbook of Ecological Modelling and Informatics ecoinformatics.org/software/eml/) is an example for developing computational tools based on metadata concepts [2, 6] that will facilitate ecological data warehousing and sharing at global scale. Feature area (3) is being largely stimulated by both the availability of complex ecological data including genomic and phenotypic data and the development of bio-inspired computational tech- niques. The study of population genomics in their natural habitats without the need for isolation and laboratory cultivation of individual species has led to the new research area of ecogenomics [18] that promises to determine the impact of environmental and climate changes on biodiversity. Bio-inspired computational techniques prove to be superior in unraveling highly complex ecological data, coping with distinct nonlinearities and inducing predictive models by learning from temporal and spatial patterns. Recknagel and Cao [42] address the state of the art of eco- logical informatics by artifi cial neural networks, evolutionary algorithms and object-oriented programming. Research into hybrid modelling in feature area (4) promises ecosystem models with improved accuracy and generality. Cao and Recknagel [32] provide a case study for multi-objective opti- misation of process and parameter representations in process-based ecosystem models by the embodiment of evolutionary algorithms in ordinary differential equations for food web dynamics and nutrient cycles in lakes. 3 Future directions Making informed decisions on preserving biodiversity and sustainable environments in spite of pollution, eutrophication and climate change is of vital importance for the habitat ‘earth’ in the 21st century. Ecological informatics is challenged to contribute ecological understanding and tools for integrating, analysing and synthesising the wealth of ecological knowledge and data for making informed decision at local, regional and global scales. It is anticipated that at the next stage, ecological informatics will distinctively focus on: (1) improved understanding of information processing in ecosystems [43]; (2) integrated analysis of genomic, phenotypic and ecological data to better understand biodiversity and ecosystem behav- iour in response to habitat and climate changes; (3) integration and visualisation ecological data by GIS and remote sensing at regional and global scale [44]; (4) facilitating data sharing by www-based generic data warehouses tailored for ecosystem categories at the global scale [45], and (5) implementing hybrid model libraries generic for ecosystem categories at the global scale by object-oriented programming and interactive www-access. References [1] Recknagel, F. (ed.), Ecological Informatics. Understanding Ecology by Biologically-In- spired Computation, Springer-Verlag: Berlin, 2003. [2] Michener, W.K., Brunt, J.W., Helly, J.J., Kirchner, T.B. & Stanford, S.G., Nongeospatial metadata for the ecological sciences. Ecological Applications, 7(1), pp. 330–342, 1997. [3] Dolk, D.R., Integrated model management in the data warehouse area. European Journal of Operational Research, 1222, pp. 1999–1218, 2000. [4] Sen, A., Metadata management: past, present and future. Decision Support Systems, 1043, pp. 1–23, 2003. [5] Eleveld, M.A., Schrimpf, W.B.H. & Siegert, A.G., User requirements and information defi - nition for the virtual coastal and marine data warehouse. Ocean & Coastal Management, 46, pp. 487–505, 2003. WIT Transactions on State of the Art in Science and Engineering, Vol 34, © 2009 WIT Press www.witpress.com, ISSN 1755-8336 (on-line) Ecological Informatics: Current Scope and Feature Areas 45 [6] Michener, W.K., Meta-information concepts for ecological data management. Ecological Infor- matics, 1, pp. 3–7, 2006. [7] Fielding, A., Machine Learning Methods for Ecological Applications, Kluwer: Dordrecht, 1999. [8] Lek, S. & Guegan, J.-F. (eds), Artifi cial Neuronal Networ ks. Application to Ecology and Evolution, Springer: Berlin, 2000. [9] Grimm, V. & Railsback, S.F., Individual-Based Modelling and Ecology, Princeton Univer- sity Press: Princeton, NJ, 2005. [10] Recknagel, F. (ed.), Ecological Informatics. Scope, Techniques and Applications, 2nd edn, Springer-Verlag: Berlin, 2006. [11] Overbeck, R., Fonstein, M., D’Souza, M., Pusch, G.D. & Maltsev, N., The use of gene clusters to infer functional coupling. Proceedings
Details
-
File Typepdf
-
Upload Time-
-
Content LanguagesEnglish
-
Upload UserAnonymous/Not logged-in
-
File Pages7 Page
-
File Size-