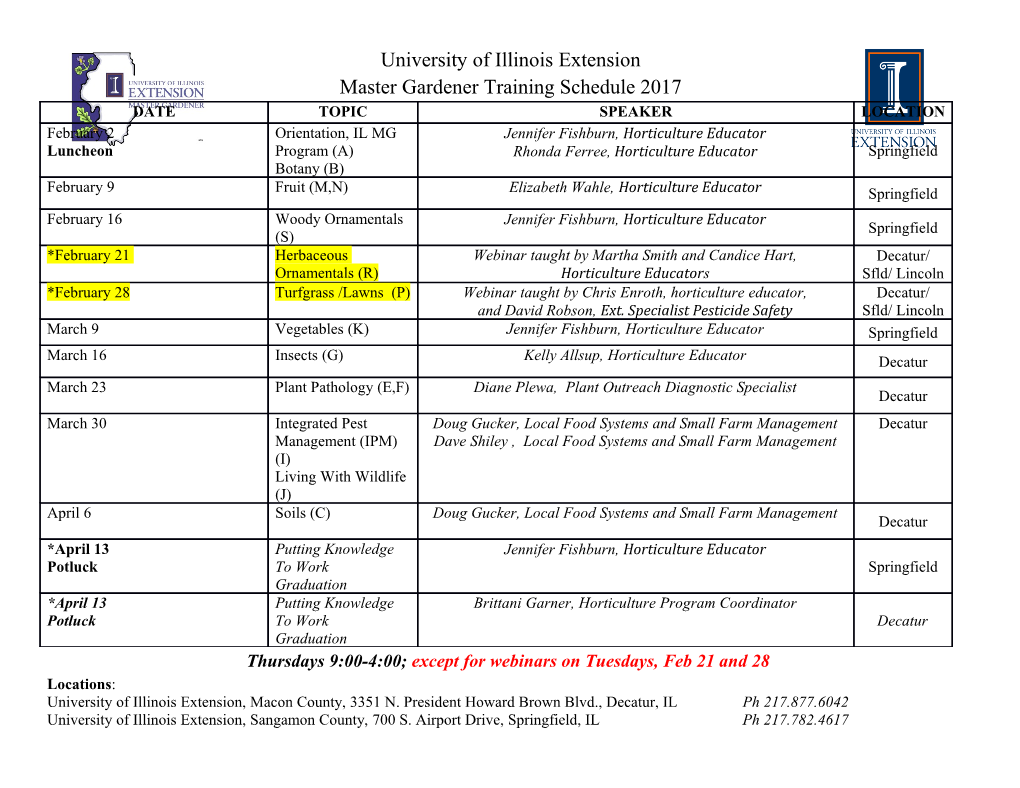
Chapter 47 VECTOR AUTOREGRESSIONS AND COINTEGRATION* MARK W. WATSON Northwestern University and Federal Reserve Bank of Chicago Contents Abstract 2844 1. Introduction 2844 2. Inference in VARs with integrated regressors 2848 2.1. Introductory comments 2848 2.2. An example 2848 2.3. A useful lemma 2850 2.4. Continuing with the example 2852 2.5. A general framework 2854 2.6. Applications 2860 2.7. Implications for econometric practice 2866 3. Cointegrated systems 2870 3.l. Introductory comments 2870 3.21 Representations for the I(1) cointegrated model 2870 3.3. Testing for cointegration in I(1) systems 2876 3.4. Estimating cointegrating vectors 2887 3.5. The role of constants and trends 2894 4. Structural vector autoregressions 2898 4.1. Introductory comments 2898 4.2. The structural moving average model, impulse response functions and variance decompositions 2899 4.3. The structural VAR representation 2900 4.4. Identification of the structural VAR 2902 4.5. Estimating structural VAR models 2906 References 2910 *The paper has benefited from comments by Edwin Denson, Rob Engle, Neil Ericsson, Michael Horvath, Soren Johansen, Peter Phillips, Greg Reinsel, James Stock and students at Northwestern University and Studienzentrum Gerzensee. Support was provided by the National Science Foundation through grants SES-89-10601 and SES-91-22463. Handbook of Econometrics, Volume IV, Edited by R.F. Engle and D.L. McFadden © 1994 Elsevier Science B.V. All rights reserved 2844 M.W. Watson Abstract This paper surveys three topics: vector autoregressive (VAR) models with integrated regressors, cointegration, and structural VAR modeling. The paper begins by developing methods to study potential "unit root" problems in multivariate models, and then presents a simple set of rules designed to help applied researchers conduct inference in VARs. A large number of examples are studied, including tests for Granger causality, tests for VAR lag length, spurious regressions and OLS estimators of cointegrating vectors. The survey of cointegration begins with four alternative representations ofcointegrated systems: the vector error correction model (VECM), and the moving average, common trends and triangular representations. A variety of tests for cointegration and efficient estimators for cointegrating vectors are developed and compared. Finally, structural VAR modeling is surveyed, with an emphasis on interpretation, econometric identification and construction of efficient estimators. Each section of this survey is largely self-contained. Inference in VARs with integrated regressors is covered in Section 2, cointegration is surveyed in Section 3, and structural VAR modeling is the subject of Section 4. 1. Introduction Multivariate time series methods are widely used by empirical economists, and econometricians have focused a great deal of attention at refining and extending these techniques so that they are well suited for answering economic questions. This paper surveys two of the most important recent developments in this area: vector autoregressions and cointegration. Vector autoregressions (VARs) were introduced into empirical economics by Sims (1980), who demonstrated that VARs provide a flexible and tractable frame- work for analyzing economic time series. Cointegration was introduced in a series of papers by Granger (1983), Granger and Weiss (1983) and Engle and Granger (1987). These papers developed a very useful probability structure for analyzing both long-run and short-run economic relations. Empirical researchers immediately began experimenting with these new models, and econometricians began studying the unique problems that they raise for econo- metric identification, estimation and statistical inference. Identification problems had to be confronted immediately in VARs. Since these models don't dichotomize variables into "endogenous" and "exogenous," the exclusion restrictions used to identify traditional simultaneous equations models make little sense. Alternative sets of restrictions, typically involving the covariance matrix of the errors, have been used instead. Problems in statistical inference immediately confronted researchers using cointegrated models. At the heart of cointegrated models are "integrated" variables, and statistics constructed from integrated variables often behave in nonstandard ways. "Unit root" problems are present and a large research effort has attempted to understand and deal with these problems. This paper is a survey of some of the developments in VARs and cointegration that have occurred since the early 1980s. Because of space and time constraints, certain topics have been omitted. For example, there is no discussion of forecasting or data analysis; the paper focuses entirely on structural inference. Empirical Ch. 47: Vector Autoregressions and Cointegration 2845 questions are used to motivate econometric issues, but the paper does not include a systematic survey of empirical work. Several other papers have surveyed some of the material covered here. In particular, the reader is referred to the survey on VARs by Canova (1991) and to surveys on statistical issues in integrated and cointegrated systems by Campbell and Perron (1991), Engle and Yoo (1991), Phillips (1988) and Phillips and Loretan (1989). Excellent textbook treatments of many of the issues discussed here can be found in Banerjee et al. (1993) and Hamilton (1994). Before proceeding, it is useful to digress for a moment and introduce some notation. Throughout this paper, I(d) will denote a variable that is integrated of order d, where d is an integer. For our purposes, an I(d) process can be defined as follows. Suppose that c~(L)xo,t = O(L)~t, where the roots of the polynomial ~b(z) and O(z) are outside the unit circle and ~t is a martingale difference sequence with variance tr 2. In other words, Xo, t follows a covariance stationary and invertible autoregressive moving average (ARMA) process. Let xd,t be defined recursively by Xd,t ---- Zts= 1Xd- 1,~, for d = 1..... Then x d is defined as I(d). This definition says that an I(d) process can be interpreted as a d-fold partial sum of stationary and invertible ARMA process. Many of the statistical techniques surveyed in this chapter were developed to answer questions concerning the dynamic relationship between macroeconomic time series. With this in mind, it is useful to focus the discussion of econometric techniques on a set of concrete economic questions. The questions concern a macroeconomic system composed of eight time series: the logarithms of output (y), consumption (c), investment (i), employment (n), nominal wages (w), money (m), prices (p) and the level of nominal interest rates (r). Economic hypotheses often restrict the Granger (1969) causal structure of the system. A classic example is Hall's (1978) interpretation of the permanent income/ life-cycle model of consumption. In Hall's model, consumption follows a martingale, so that c t_ 1 is an optimal forecast of c r Thus, the model predicts that no variables in the system will Granger-cause consumption. When the data are integrated, some important and subtle statistical issues arise when this proposition is tested. For example, Mankiw and Shapiro (1985) demonstrate that unit root problems plague the regression of Ac t onto Yt- 1: standard critical values for Granger-causality test statistics lead to rejection of the null hypothesis far too frequently when the null is true. On the other hand, Stock and West (1988) show that these unit root problems disappear when Granger causality is tested using the regression of c t onto c~_ 1 and Yt-1, but then reappear in the regression of c, onto ct_" 1 and m r_r The Mankiw-Shapiro/Stock-West results are explained in Section 2 which focuses on the general problem of inference in regression models with integrated regressors. Economic theories often restrict long-run relationships between economic vari- ables. For example, the proposition that money is neutral in the long run implies that exogenous permanent changes in the level of m, have no long-run effect on the level ofy r When the money-output process is stationary, Lucas (1972) and Sargent (1972) show that statistical tests of long-run neutrality require a complete specifi- cation of the structural economic model generating the data. However, when money and output are integrated, Fisher and Seater (1993), show that the neutrality 2846 M.W. Watson proposition is testable without a complete specification of the structural model. The basic idea is that when money and output are integrated, the historical data contain permanent shocks. Long-run neutrality can be investigated by examining the relationship between the permanent changes in money and output. This raises two important econometric questions. First, how can the permanent changes in the variables be extracted from the historical time series? Second, the neutrality proposition involves "exogenous" components of changes in money; can these components be econometrically identified? The first question is addressed in Section 3, where, among other topics, trend extraction in integrated processes is discussed. The second question concerns structural identification and is discussed in Section 4. One important restriction of economic theory is that certain "Great Ratios" are stable. In the eight-variable system, five of these restrictions are noteworthy. The first four are suggested by the standard neoclassical growth model. In response to exogenous growth in productivity and population, the neoclassical growth model predicts that output, consumption and investment will grow in a balanced way. That is, even though Yt, ct, and i t increase permanently in response to increases in productivity and population, there are no permanent shifts in c t - Yt and i t - Yr The model also predicts that the marginal product of capital will be stable in the long run, suggesting that similar long-run stability will be present in ex-post real interest rates, r- Ap. Absent long-run frictions in competitive labor markets, real wages equal the marginal product of labor. Thus, when the production func- tion is Cobb-Douglas (so that marginal and average products are proportional), (w- p)- (y- n) is stable in the long run.
Details
-
File Typepdf
-
Upload Time-
-
Content LanguagesEnglish
-
Upload UserAnonymous/Not logged-in
-
File Pages73 Page
-
File Size-