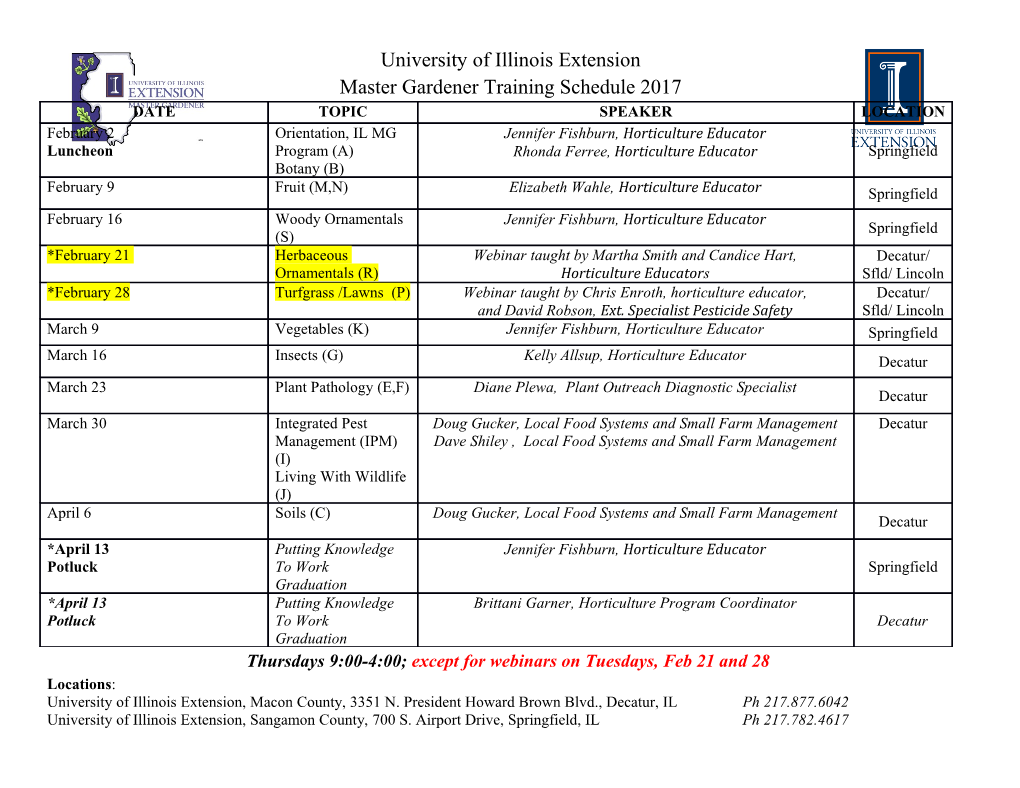
Making Sense of the Science of WM Zachary Hunter Bing Center for WM Dana-Farber Cancer Institute Harvard Medical School Bing Center for WM Harvard Research at the Medical Dana-Farber School Cancer Institute e Bing Center for WM Research at the Dana-Farber Cancer Institute Understanding Genetics If you only had four letters to work with, what kind of story could you tell? Genomics is easy… ➢ Deoxyribonucleic Acid (DNA) is made of complex molecules called nucleotides. There are 4 types abbreviated A,T,C,G. ➢ Nucleotide bases form stables pairs: A-T and C-G. Two complimentary strands of these bases form DNA. ➢ DNA is broken into 23 long strands known as chromosomes. ➢ The sections of the DNA that contain instructions on how to build proteins are called genes. ➢ Genes in the DNA are transcribed into a single strand of similar nucleotides called Ribonucleic Acid (RNA) and this “message” is processed by the cell and turned into protein. Something easy with ~ 3,000,000,000 pieces can be really complicated… For context, computers operate with just two “bases” 0 and 1. With enough 0s and 1s it turns out you can make computer’s do some pretty impressive and complicated stuff. DNA has 4 bases, x 3 Billion with a lot more chemical and spatial annotation. This makes IBM’s Watson look simple by comparison, even if it does beat us at Jeopardy DNA – RNA – Protein • Transcribed • Stores Provides from DNA information structure, • Encodes signaling, • Used as a instructions and carries template to make out most for RNA protein cellular work • Genes are regions of the DNA that are transcribed into RNA • RNA carries the DNA code out into the rest of the cell where it can be used as instructions to make protein How to make it all fit… 3 billion base pairs strung end to end is about 40 inches in length. This quantity of DNA resides inside the nucleus of every cell in your body Needless to say, this is a tightly controlled process. Our apologies, the DNA you are looking for is temporarily unavailable… Laura, B. (2008) Epigenomics: The new tools in studying complex disease. Nature Education Introducing the “omes” The Genome The Transcriptome The Proteome • Transcribed • Stores Provides from DNA information structure, • Encodes signaling, • Used as a instructions and carries template to make out most for RNA protein cellular work The Epigenome The genome as the cell’s operating system The Environment The operating system of your Protein computer (Windows/Max OSX/Linux) provides a set of tools to allow programs to be loaded and run. Every laptop may have the same operating system, but no one uses their RNA DNA laptop in exactly the same way DNA to Protein… translation please? ➢ Proteins are folded chains of amino acids ➢ Just like there are 4 bases that make of DNA, there are 20 different amino acids ➢ Groups of 3 DNA base pairs, called codons, code code for specific amino acids for example: DNA:CTC -> RNA:CUC -> Leucine ➢ T (thymidine) in the DNA is transcribed as U (uracil) in the RNA. DNA to Protein… translation please? DNA Amino Acid Translation XGAT GAT TAC CTG DDYL ATG ATT ACC TGC MITC Frame-shift The Genomics of WM Understanding the WM “Operating System” Mutation and Cancer Cancer can be caused by accidental changes in the genome known as mutations As these accumulate, genes start to gain new functions, or lose old ones At the point that the cells start to multiply in an uncontrolled way, we call it cancer A single base pair TLR4 IL1R mutation the gene MYD88 is found in over MYD88 Signaling Wait…95% of Didn’tWM I promise that T I R A thisNFKB would Nuclear Translocationbe easy? in P The BCWM.1 Cell Line MYD88 MYD88 BTK I RA 4 K AK 4 IR I RA 1 K AK 1 IR <- This doesn’t look easy! 2 TA B B TRAF6 A 1 T TAK1 NEMO Control MYD88 Inhibitor IKKα IKKβ Proteasomal IκBα Degrada on p50 p65 Nuclear Transloca on and Signaling Treon et al NEJM 2012 Don’t Sweat the details unless necessary… Simple Complex Ouch… TLRs TLR4 IL1R T I R MYD88 A P MYD88 MYD88 IRAK1/4 BTK BTK I RA 4 K AK 4 IR I RA 1 K AK 1 IR IkBα 2 TA p50 p65 B B TRAF6 A 1 T TAK1 NEMO IKKα IKKβ growth survival Proteasomal IκBα NFKBp50 p65 Degrada on p50 p65 Nuclear Transloca on and Signaling MYD88 L265P in WM/IGM MGUS METHOD TISSUE WM IGM MGUS Treon WGS/Sanger BM CD19+ 91% 10% Xu AS-PCR BM CD19+ 93% 54% Gachard PCR BM 70% Varettoni AS-PCR BM 100% 47% Landgren Sanger BM 54% Jiminez AS-PCR BM 86% 87% Poulain PCR BM CD19+ 80% Argentou PCR-RFLP BM 92% 1/1 MGUS Willenbacher Sanger BM 86% Mori AS-PCR/BSiE1 BM 80% Ondrejka AS-PCR BM 100% Ansell WES/AS-PCR BM 97% Patkar AS-PCR BM 85% Updated from Treon and Hunter. Blood. 2013;121(22):4434-6. 17 A single base pair TLR4 IL1R mutation the gene MYD88 is found in over MYD88 Signaling 90% of WM T I R A NFKB Nuclear Translocation in P The BCWM.1 Cell Line MYD88 MYD88 BTK I RA 4 K AK 4 IR I Ibrutinib RA 1 K AK 1 IR 2 TA B B TRAF6 A 1 T TAK1 NEMO Control MYD88 Inhibitor IKKα IKKβ Proteasomal IκBα Degrada on p50 p65 Nuclear Transloca on and Signaling Guang Yang, PhD Yang et al. Blood 2013 WHIM-like CXCR4 mutations in Waldenstrom’s Macroglobulinemia • 30-40% of WM patients CXCL12/SDF-1a • Occur in the C-terminal domain (same site as WHIM patients) • Nonsense and frameshift mutations Most common • Associated with high mutation is S338X IGM, hyperviscosity. • Multiple CXCR4 352 S338X mutations can be present within an individual patient. Hunter et al, Blood 2013; Rocarro et al, Blood 2014: Poulain et al, Blood 2016; Poulain et al, CCR 2016; Xu et al, BJH 2016; Varettoni et al, Haematologica 2017. Hunter et al, Blood 2013 WHIM Syndrome Like Mutations in CXCR4 Wu et al, Science, 2010 Using Mutation Status to Understand Responses to Ibrutinib Serum IgM Response Hemoglobin Response 5 5 . 1 1 8 8 . s l 1 1 s l e e v v e e L L e e n i n l i l e e s s a 6 6 a . B B 1 1 0 0 o o . t t 1 1 e e v v a a l l e e R R 4 4 . e e minor response s 1 1 s n n o o p p s s e e 5 5 R . R 0 0 par al response n i M b g 2 2 o I . l 1 1 g m o u m r e e S H t t s s e e B B very good 0 0 0 0 . par al response 1 1 0 0 L265P WT L265P WHIM WT WT MYD88L265PCXCR4 MYD88 WHIMCXCR4 MYD88 WTCXCR4 MYD88L265PL265PCXCR4WT MYD88L2WHIM65PCXCR4WHIM MYD88WWTTCXCR4WT Treon et al, NEJM 2015 Hunter et al, Blood Advances 2018 Mutations in MYD88WT WM Hunter et al, Blood Advances 2018 Ibrutinib in MYD88WT WM Serum IgM Response Somatic Deletions on Chromosome 6q Whole GenomeSequencing Deletions on Chromosome 6q Chromosome on Deletions 0 1 s t PLEKHG1 9 n e i t a HIVEP2 8 P d signaling and BCL2CXCR4 MYD88, for genesregulatory target Macroglobulinemia in common deletionsare 6q Chromosome Luisa Maria e HLA-DQA/B BCLAF1 ARID1B t 7 c e f f COL19A1 BACH2 FOXO3 A 6 f o r CD83 EEF1A1 PRDM1 MAN1A1 TNFAIP3 CCR6 5 e b m Detection Threshold IBTK u 4 N 3 Guerrera Waldenström’s 1 1 3 3 2 1 3 1 3 1 2 1 3 1 2 1 3 1 3 1 2 3 1 2 3 1 2 3 . 3 . 3 3 . 3 2 3 5 1 6 7 . 5 5 5 4 4 2 2 1 1 2 2 1 4 4 6 6 2 3 3 4 4 4 5 5 5 2 1 1 1 2 2 2 1 2 2 2 2 2 2 2 2 2 2 2 1 1 1 1 1 1 1 2 2 2 2 2 2 2 2 2 p q q q q q q 2 2 2 p p p p p p p p p p p p q q q q q q q q q q q q q p q q Chromosome 6 , and and , : MYD88Mutant WM MYD88WT WM Guererra et al, Haematologica 2018 Guererra et al, Haematologica 2018 Overlapping signatures between deletion 6q and CXCR4WHIM Gene Chrom Start End LogFC Del6q LogFC CXCR4mut FAM110C chr2 38813 41627 -3.61 -3.29 WNK2 chr9 95947211 95947892 -1.88 -4.92 SGCD chr5 155135062 155135118 -1.87 -3.26 CCDC141 chr2 179694483 179699167 -1.67 -1.30 PKHD1L1 chr8 110374705 110374882 -1.56 -1.47 EML6 chr2 54952148 54952395 -1.49 -1.03 ZNF214 chr11 7020548 7022786 -1.39 -1.85 SYTL2 chr11 85405264 85406383 -1.32 -1.30 C11orf92 chr11 111164113 111169391 -1.31 -0.91 IL17RB chr3 53880576 53880675 -1.26 -2.04 ZNF215 chr11 6947653 6947916 -1.19 -1.29 ZNF804A chr2 185463092 185463797 -1.12 -0.69 LINC00271 chr6 135818938 135819138 -0.99 -0.69 CDK14 chr7 90095737 90095827 -0.76 -0.57 FOXO3 chr6 108881025 108881218 -0.75 -0.54 CYP4V2 chr4 187112673 187113191 -0.69 -0.53 IGF2R chr6 160390130 160390427 -0.53 -0.43 EPS15 chr1 51819934 51822518 -0.39 -0.37 HRK chr12 117299026 117299271 1.84 2.02 Putting it all together : Signaling Networks in WM How we can use machine learning and bid data to better understand WM Negative Feedback Loops: Self Regulating Biological Networks A centrifugal governor is a mechanical analog to biological negative feedback loops.
Details
-
File Typepdf
-
Upload Time-
-
Content LanguagesEnglish
-
Upload UserAnonymous/Not logged-in
-
File Pages33 Page
-
File Size-