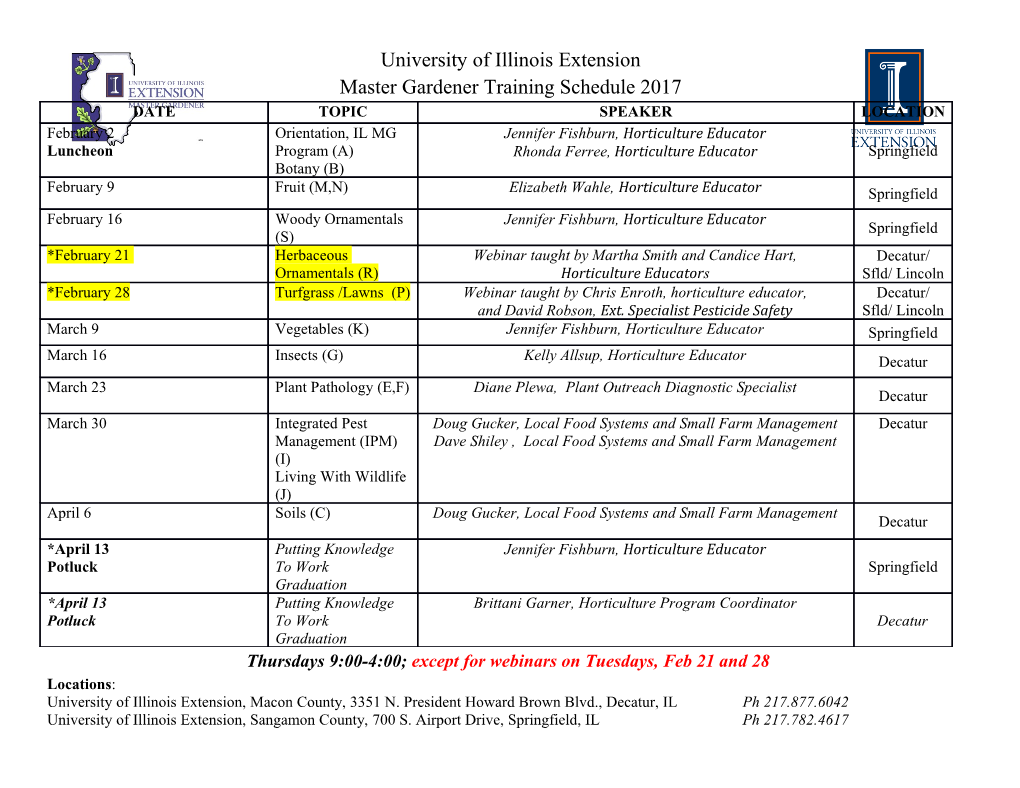
Int. J. Contemp. Math. Sciences, Vol. 7, 2012, no. 38, 1861 - 1869 Estimating Fractional Stochastic Volatility 1 Hoang Thi Phuong Thao Hanoi University of Natural Sciences Vietnam National University 334 Nguyen Trai Str. Thanh Xuan, Hanoi, Vietnam [email protected] Tran Hung Thao Institute of Mathematics Vietnamese Academy of Science and Technology No 18 Hoang-Quoc-Viet Road, Hanoi, Vietnam. [email protected] Abstract The aim of this paper is to find the best state estimation for frac- tional stochastic volatility from point process observations by a method of semimartingale approximation. Mathematics Subjects Classification: 60H30, 91B28 Keywords: State estimation, Fractional stochastic volatility, L2-approximation, Point process 1 Introduction It is known that stochastic volatility models for options and derivatives were developed out of a need to modify the Black-Scholes model, which failed to effectively take the volatility in the price of underlying security into account. The Black-Scholes model assumed that the volatility of the underlying security was constant, while stochastic volatility models categorized the price of the un- derlying security as a random variable or more general, a stochastic process. In its turn, the dynamics of this stochastic process can be driven by some other process (commonly by a Brownian motion). Various models have been created for stochastic volatility such as Heston model, Constant Elasticity of 1This research is funded by Vietnam National Foundation for Science and Technology Development (NAFOSTED) under grant number 101.02-2011.12. 1862 H. T. P. Thao and T. H. Thao Variance model (CEV model), SABR volatility model, GARCH model, 3/2- model, Chen model,. In many situations, the dynamics of the volatility has a long-range effect, for instance changes of very strong bluechips in a stock market. One thinks of a kind of stochastic volatility perturbed by a process of long memory such as a fractional Brownian motion. In this paper we consider the problem of state estimation of a stochastic volatil- ity Vt given by the fractional stochastic differential equation H dVt = −bVtdt + σdBt , H where Bt is a fractional Brownian motion of Liouville form, from a point process observation Yt. H Recall that a fractional Brownian motion Wt with Hurst index H is a centered H H Gaussian process such that its covariance function R(s, t)=EWs Wt is given by 1 R(s, t)= (t2H + s2H −|t − s|2H ) where 0 <H<1. 2 H 1 ,R s, t s, t W H If = 2 ( ) = min( ) and t is the usual standard Brownian motion that is a Markov process. H 1 ,WH For = 2 t is neither a semimartingale nor a Markov process. It is a process of long memory. H According to B. Mandelbrot, Wt has the following representation t H 1 α Wt = [Zt + (t − s) dWs], Γ(1 + α) 0 W α H − 1 where is a standard Brownian motion, = 2 and 0 α α Zt = [(t − s) − (−s) ]dWs. −∞ Since Zt has absolutely continuous trajectories [1] one can see that the term BH t t − s αdW ρ t := 0 ( ) s has long memory in the following sense (see [4]). If n = H H H ∞ E(B1 (Bn+1 − Bn )) then the series n=0 ρn is either divergent or convergent H with very late rate. Bt is not a semimartingale as well and it is known as a fractional Brownian motion of Liouville form. Every stochastic processes here are supposed to be adapted to a filtration (Ft) in a probability space (Ω, F,P). Now we try to estimate the stochastic volatility Vt satisfying H dVt = −bVtdt + σdBt , 0 ≤ t ≤ T, (1.1.1) Estimating fractional stochastic volatility 1863 2 V0 ∼ N(μ0,ν0 ),b and σ are positive constants from an observation given by a point process of intensity λt t Yt = λsds + Mt, (1.1.2) 0 λ E t λ2ds < ∞ M F where t is a progressive process such that 0 s , t is a t- mar- H tingale and V0,Bt and Mt are independent. We will use an approximation approach given in [7] to solve this problem. H 2 Approximation for the fractional process Bt In this section we will make a brief recall on a principal result for L2-approximation of the fractional Brownian motion (refer to [7]). For every >0 we define t H, H− 1 Bt = (t − s + ) 2 dWs, (2.2.1) 0 H 1 , <H< , ≤ t ≤ T. with = 2 0 1 0 H, Lemma 2.1. The process (Bt ,t≥ 0) is a semimartingale with respect to the W H, natural filtration Ft = σ(Ws,s≤ t). In fact, Bt can be expressed as t H, α Bt = α ϕsds + Wt, (2.2.2) 0 where t α−1 1 ϕt = (t − u + ) dWu,α= H − , 0 <H<1. (2.2.3) 0 2 H, H 2 Theorem 2.1. (See[7]) Bt converges to Bt in L (Ω) when tends to 0. This convergence is uniform with respect to t ∈ [0,T]. Moreover we have H, H α+ 1 ||Bt − Bt ||L2(Ω) ≤ C(α) 2 where C(α) depends only on α. By this theorem we can see now any fractional stochastic dynamical sys- tem driven by a fractional Brownian notion can be approximated in L2 by a system governed by a semimartingale Bt , where the traditional stochas- tic calculus can be applied. Then the remaining work is to prove the con- vergence of approximate solutions to the exact solution. Notice that the exact solution of (1.1) can be defined as an adapted process Vt satifying V −b t V ds BH ,V ∼ N μ ,ν2 . t = 0 s + t 0 ( 0 0 ) And our method of estimating stochastic volatility Vt is included in this con- text. 1864 H. T. P. Thao and T. H. Thao 3 Approximate Fractional Stochastic Volatil- ity Instead of (1.1) and (1.2) we consider the following estimating problem: The system dynamics for volatility is given by H, dVt = −bVt dt + σdBt , 0 ≤ t ≤ T (3.3.1) 2 V0 = V0 ∼ N(μ0,ν0 ). And the observation dynamics is t Yt = λsds + Mt, 0 ≤ t ≤ T. (3.3.2) 0 H, By replacing Bt in (3.1) by its expression in (2.2) we have α dVt =[−bVt + σαϕt]dt + σdWt. (3.3.3) We can see that (3.3) can be splitted into two equations ,1 ,1 α dVt = −bVt dt + σdWt, (3.3.4) ,2 ,2 dVt =[−bVt + σαϕt]dt. (3.3.5) Where (3.4) is a traditional stochastic Langevin equation and (3.5) is in fact an ordinary differential equation. And the solution Vt of (3.3) should be ,1 ,2 Vt = Vt + Vt . So the state estimation problem is splitted into two problems as well Y ,1 Y ,2 Y E(Vt |Ft )=E(Vt |Ft )+E(Vt |Ft ). Notation: Y (i) The state estimation of a process Xt based on the information Ft given by an observation process Yt will be denoted by Xˆt or πt(X). More Y general, the notation πt(f) stands for the state estimation E(f(Xt)|Ft ), where f is a bounded and continuous function, f ∈ Cb(R). Recall that Y Ft = σ(Ys,s ≤ t) is the σ-algebra generated by all random variable Ys with 0 ≤ s ≤ t. (ii) Suppose that the probability P is obtained from a probability Q by an absolutely continuous change of measure Q → P such that nt = Yt − t Estimating fractional stochastic volatility 1865 Y is a (Q, Ft )- martingale. A Bayes formula gives us Y Y EQ(XtLt|Ft ) EP (Xt|Ft )= , EQ(Lt) where dP t L E |F Y λ Y − λ ds. t = [dQ t ]= s s exp (1 s) 0≤s≤t 0 Denote by σ(Xt)orX˜t the unnormalized state estimation of Xt, given by Y X˜t = σ(Xt)=EQ(LtXt|Ft ), or more general Y σ(f)=EQ(Ltf(Xt)|Ft ), for f ∈ Cb(R). ,1 4 Estimating Vt ,1 Y Theorem 4.1. (i) The state estimation πt(f)=E[f(Vt )|Ft ] for the sys- tem dynamics (3.4) with the point process observation (3.2) is given by t α 2 ,1 ,1 ( σ) ,1 πt(f)=π0(f)+ πs[−bVt f (Vt )+ f (Vt )]ds 0 2b t −1 + πs (λ)[πs−(λf) − πs(f)πs−(λ)]dms, (4.4.1) 0 m Y − t λ ds, f ∈ C2 R . where t = t 0 s b ( ) ,1 Y (ii) The unnormalized state estimation σt(f)=EQ[Ltf(Vt )|Ft ] is given by t α 2 ,1 ,1 ( σ) ,1 σt(f)=σ0(f)+ σs[−bVt f (Vt )+ f (Vt )]ds 0 2b t + [σs−(λf) − σs(f)]dμs, (4.4.2) 0 2 where μt = Yt − t, f ∈ Cb (R). 1866 H. T. P. Thao and T. H. Thao ,1 Proof. It is known that the solution Vt is in fact an Ornstein-Uhlenbeck process that is a Markov process and its semigroup is defined by a family (Pt,t≥ 0) of operations on bounded Borelian function f. ασ 2 −bt ( ) −2bt (Ptf)(x)= f[e x + 1 − e y]μ(dy), (4.4.3) 2b Ê where μ is a standard Gaussian measure on R 1 −y2 μ(dy)=√ exp ( )dy. 2π 2 ,1 It is obvious that lim(Ptf)(x)=f(x) then Vt is a Feller process. The corre- t0 sponding infinitesimal operator At is given by α 2 1 ( σ) (Atf)(x) = lim (Ptf − f)(x)=−bxf (x)+ f (x). (4.4.4) t→0 t 2b According to a result from [5], the state estimation πt(f) for a Fellerian system Y t λ ds M process from a point process observation t = 0 s + t is given by t t −1 πt(f)=π0(f)+ πs(Af)ds + πs (λ)[πs−(λf) − πs(f)πs−(λ)]dms, 0 0 m Y − t π λ ds, π−1 where t = t 0 s( ) provided t = 0 and the unnormalized state estimation σt(f) is given by t t σt(f)=σ0(f)+ σs(Af)ds + [σs−(λf) − σs(f)]dμs, 0 0 where μt = Yt − t.
Details
-
File Typepdf
-
Upload Time-
-
Content LanguagesEnglish
-
Upload UserAnonymous/Not logged-in
-
File Pages9 Page
-
File Size-