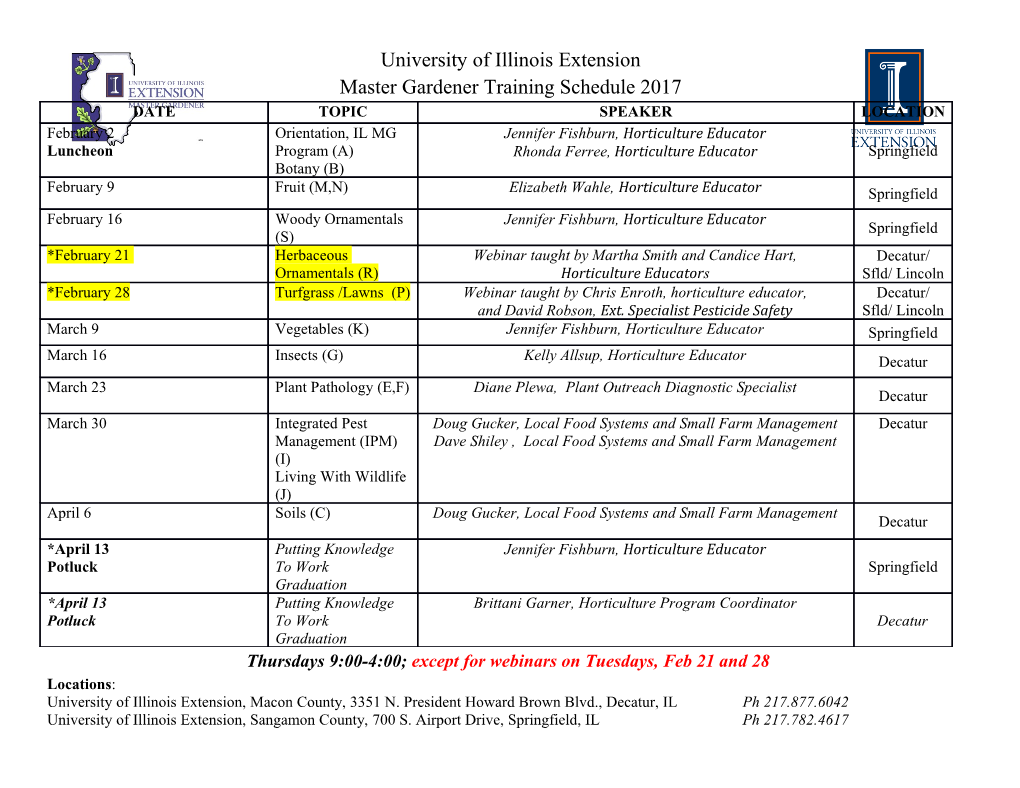
Brain mechanisms of affect and learning Jenna M. Reinen Submitted in partial fulfillment of the Requirements for the degree of Doctor of Philosophy In the Graduate School of Arts and Sciences COLUMBIA UNIVERSITY 2014 © 2014 Jenna M. Reinen All rights reserved Brain mechanisms of affect and learning by Jenna M. Reinen is licensed under a Creative Commons Attribution-NonCommercial-NoDerivatives 4.0 International License. ii ABSTRACT Brain mechanisms of affect and learning Jenna M. Reinen Learning and affect are considered empirically separable, but these constructs bidirectionally interact. While it has been demonstrated that dopamine supports the informational component of reward learning, the term “reward” inherently infers that a subjective positive experience is necessary to drive appetitive behavior. In this dissertation, I will first review the ways in which dopamine operates on the levels of physiology and systems neuroscience to support learning from both positive and negative outcomes, as well as how this framework may be employed to study mechanism and disease. I will then review the ways in which learning may interact with or be supported by other brain systems, starting with affective networks and extending into systems that support memory and other types of broader decision making processes. Finally, my introduction will discuss a disease model, schizophrenia, and how applying questions pertaining to learning theory may contribute to understanding symptom- related mechanisms. The first study (Chapter 2) will address the way in which affective and sensory mechanisms may alter pain-related decisions. I will demonstrate that subjects will choose to experience a stimulus that incorporates a moment of pain relief over a shorter stimulus that encompasses less net pain, and will suggest that the positive prediction error associated with the pain relief may modulate explicit memory in such a way that impacts later decision making. iii In the second study (Chapter 3), I will examine reward learning in patients with schizophrenia, and demonstrate selective learning deficits from gains as opposed to losses, as well as relationships in performance to affective and motivational symptoms. The third study (Chapter 4) will extend this disease model to a novel cohort of subjects who perform the same reward learning task while undergoing functional MRI. The data from this chapter will reveal deficits in the patient group during choice in orbitofrontal cortex, as well as an abnormal pattern of learning signal responses during feedback versus outcome, particularly in orbitofrontal cortex, a finding that correlates with affective symptoms in medial PFC. Taken together, these data demonstrate that learning is comprised of both informational and affective processes that incorporate input from dopaminergic midbrain neurons and its targets, as well as integration from other affective, mnemonic, and sensory regions to support healthy learning, emotion, and adaptive behavior. iv Table of Contents List of Charts, Graphs, and Illustrations iii List of Tables iv Acknowledgements v Chapter 1: Introduction 1 I. Rationale: dopamine and learning 2 II. Affective processing 7 III. Memory, valuation, and decision making 12 IV. The negative symptoms of schizophrenia 14 V. Overview of the current research 18 Chapter 2: Affective and sensory mechanisms impacting the valuation of pain 21 Introduction 22 Methods 24 Results 30 Discussion 35 Chapter 3: Patients with schizophrenia are impaired when learning in the context 42 of pursuing rewards Introduction 43 Methods 45 Results 49 Discussion 55 Supplementary text 58 i Chapter 4: Neural mechanisms of choice, anticipation, and outcome during 65 reward learning in schizophrenia Introduction 66 Methods 69 Results 78 Discussion 90 Chapter 5: General Discussion and Conclusions 96 Behavioral indicators of affect as a modulator of learning 97 How does dopamine contribute to learning, motivation, and affect? 97 Implications for negative symptoms of schizophrenia 102 Limitations and Future Directions 107 References 108 ii List of Charts, Graphs, and Illustrations Figure 2.1. Associative learning and decision making phases of Experiment 1 27 Figure 2.2. Example stimulation profiles for each type of pain stimulus in Experiment 1 29 Figure 2.3. Example stimulation profiles for each type of pain stimulus across Experiment 2 29 Figure 2.4. Mean online ratings for each stimulus type across subjects 30 Figure 2.5. Summary of Subject Ratings, Experiment 1 32 Figure 2.6. Predicted versus reported pain for each rating category 34 Figure 2.7. Summary ratings for subjects who made and did not make (b) online ratings in Experiment 35 Figure 3.1. Experimental Task Structure 48 Figure 3.2. Probabilistic aspect of the reward paradigm 48 Figure 3.3. Mean optimal choice across blocks 52 Figure 3.4. Differences in performance for each group and condition 52 Figure S3.1. Logistic regression model 64 Figure 4.1. Probabilistic structure 73 Figure 4.2. Reaction time 79 Figure 4.3. Mean optimal choice across blocks in fMRI study 80 Figure 4.4. Model parameters 81 Figure 4.5. Choice value in the gain condition in healthy controls 83 Figure 4.6. Figure 4.6. Response to verbal feedback prediction error in the striatum in controls and patients 86 Figure 4.7. Response to monetary outcome prediction error in the striatum in controls and patients 88 Figure 4.8. Relative response to prediction error during verbal feedback > monetary outcome 89 iii List of Tables Table 3.1. Patient and Control Demographics 46 Table S3.1. Correlations between age and each dependent measure in each Condition 61 Table S3.2. Correlations between education and each dependent measure in each condition 61 Table 4.1. Participant Demographics 70 Table 4.2 Chance of reward outcome 74 Table 4.3. Responses to choice value in regions of interest 83 Table 4.4. Responses to verbal feedback prediction error in regions of interest 85 Table 4.5. Responses to reward outcome prediction error in regions of interest 87 iv ACKNOWLEDGMENTS The exceptional and inspiring individuals with whom I have been lucky enough to work have defined my experience at Columbia. I am beyond grateful for the amazing instruction, mentorship, and research training I have received, and consider myself lucky to have been exposed to the ideas and projects that originate here. In particular, I am grateful to the late Ed Smith for allowing me to help him to conduct a large-scale research project exploring the negative symptoms of schizophrenia, and for providing me with thoughtful research suggestions, edits, words of wisdom, and a solid laugh along the way. His inspiration, warmth, and infectious enthusiasm for cognitive neuroscience will not be forgotten. I also am especially thankful to Daphna Shohamy for extraordinary mentorship theoretically, analytically, and interpersonally. From practical advice for navigating the academic landscape to framing a paper with solid mechanistic hypotheses, Daphna has provided me not only with thorough research training, but continually inspires her lab to focus on the greater goal of our scientific pursuits with a collegial spirit of honesty and respect. In addition, Tor Wager’s creative and analytically thorough approach to brain imaging are nothing short of inspirational and progressive, and I am thankful that I’ve had the opportunity to collaborate and learn from him. Further, I’m thrilled to have Deanna Barch join my committee and bring with her an expertise on the cognitive neuroscience of schizophrenia, as well as Kevin Ocshner and Dean Mobbs, both of whom are generous to offer their time and insight on affective neuroscience. And v finally, I’ve learned a great deal from L. Fredrik Jarskog and Anissa Abi-Dargham, whose collaborative clinical expertise added an important dimension to my work. I also count myself lucky for having been able to learn among an amazing group of graduate students and post docs. I extend my sincere thanks to Elliott Wimmer, who taught me a great deal about computational analysis with great patience, accuracy, and good humor, and to Juliet Davidow, a dear and wise friend who has seen me through the agony and ecstasy of data analysis and graduate school life. And also to Raphael Gerraty, E. Kendall Braun, Nina Rouhani, Brad Doll, Teal Eich, Ajay Satpute, Lauren Atlas, Katherine Duncan, Jared Van Snellenberg, and all the post docs and graduate students who have provided me with help, ideas, and encouragement along the way. Next, there are a number of research assistants and lab managers who were critical contributors to the studies in this dissertation: Katie Insel, a dear and respected colleague who struggled and succeeded along with me to collect data from a patient population, Jochen Weber, who has sat patiently by me while I learned NeuroElf, and also to Sergio F. M. Zenisek, Damon Abraham, Rob Kribs, and Ashley Lee, all of whom I am delighted to call colleagues and friends. And to my friends, family, and loved ones who have—despite a few good- natured jokes—been beside me the whole way: Daria, Peter, and Wes Rockholz, Marybeth Robb and the entire Robb family, Claudia Gerola, Stephie Frommer Rojas, Megan Robb, Kimberly Kress Isserlis, Carla Rothenberg, Natalie Johnston, Nicole Arnot, Rahia Mashoodh, and Ljubica Chatman. And finally, to my father, Jeff Reinen, who passed away long ago, but whose warmth, curiosity, honesty, and passionate search for truth endures as inspiration. vi Chapter 1: Introduction 1 Introduction I. Rationale: Dopamine and Learning One of the most widely studied topics in behavioral and neural science addresses how animals learn to associate a predictive cue in the environment with good or bad outcomes. Rescorla and Wagner proposed a model that addresses how, through repeated associations, an animal learns computationally to anticipate an inherently rewarding outcome from a predictive cue. Specifically, it was hypothesized that in order to track the value of the cue over time, dopamine neurons evaluate the difference between the reward expected and the reward received (Rescorla and Wagner, 1972).
Details
-
File Typepdf
-
Upload Time-
-
Content LanguagesEnglish
-
Upload UserAnonymous/Not logged-in
-
File Pages133 Page
-
File Size-