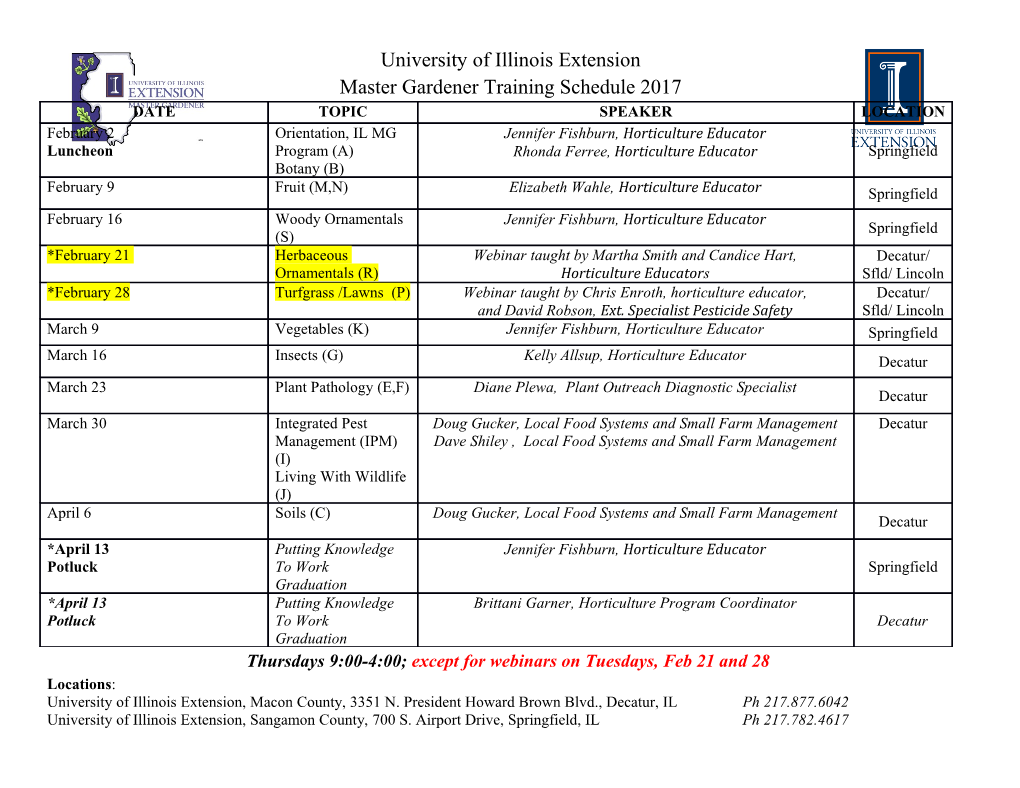
Caption Crawler: Enabling Reusable Alternative Text Descriptions using Reverse Image Search Darren Guinness Edward Cutrell Meredith Ringel Morris University of Colorado Boulder Microsoft Research Microsoft Research Boulder CO, USA Redmond WA, USA Redmond WA, USA [email protected] [email protected] [email protected] ABSTRACT adopted. Prior investigations examining alt text have Accessing images online is often difficult for users with demonstrated coverage of about 50% of images in heavily vision impairments. This population relies on text trafficked websites [6]. However, alt text coverage is also a descriptions of images that vary based on website authors’ feature used to rank pages in search engines [24] and can accessibility practices. Where one author might provide a often be abused, meaning that the alt text might simply state descriptive caption for an image, another might provide no the image’s filename or say “img” or “a picture in this page” caption for the same image, leading to inconsistent [11]. This often means that an image on one domain might experiences. In this work, we present the Caption Crawler have a high-quality description, while another site might system, which uses reverse image search to find existing have a poor-quality description or none at all for the same captions on the web and make them accessible to a user’s image, leading to an inconsistent browsing experience for screen reader. We report our system’s performance on a set screen reader users. of 481 websites from alexa.com’s list of most popular sites to estimate caption coverage and latency, and also report Researchers have been working to increase online image blind and sighted users’ ratings of our system’s output accessibility through a large range of approaches. The quality. Finally, we conducted a user study with fourteen approaches focused on captioning images can be divided into screen reader users to examine how the system might be used three categories: crowdsourcing, machine-generated, and for personal browsing. hybrid systems. Crowdsourcing captioning methods rely on human annotators in order to help describe a photo [4, 8, 9, Author Keywords 27, 34]. Machine-generated captioning approaches rely on Accessibility; vision impairment; image captioning; screen machine learning, usually using trained computer vision readers; alternative text; alt text. models to recognize objects and relationships in an image ACM Classification Keywords and to produce a caption [12, 13, 17, 26, 33, 37]. Hybrid K.4.2. [Computers and society]: Social issues – assistive approaches often rely on a combination of automatically technologies for persons with disabilities. generated descriptions or tags with human editing [6, 28, 29] to reduce time and financial costs associated with recruiting INTRODUCTION human crowd workers. Understanding images on the web can be a difficult task for people with vision impairments. One accessibility practice Our work contributes to the effort to improve the availability used to make images more accessible is to describe images of image captions using a fully-automated approach, but using alternative text, often abbreviated as alt text, and unlike prior automated systems we do not rely on computer specified within the “alt” attribute of the “img” element in vision. Instead, our insight is that many images appear in HTML. According to the WCAG 2.0 standard, alt text several places across the web; our Caption Crawler system specifies an HTML property that provides a short text finds cases where some copies of an image have alt alternative to the image that should contain any words in the attributes, and propagate this alt text to copies of the image image and must convey the overall meaning of the image that lack the description, thereby producing human-quality [36]. However, this practice has not been universally captioning. Our research explores the feasibility of increasing access to shared images online using existing Permission to make digital or hard copies of all or part of this work for personal or classroom use is granted without fee provided that copies are search infrastructure without incurring additional costs in not made or distributed for profit or commercial advantage and that copies human labeling time or other resources. bear this notice and the full citation on the first page. Copyrights for components of this work owned by others than the author(s) must be This paper presents three main contributions: honored. Abstracting with credit is permitted. To copy otherwise, or republish, to post on servers or to redistribute to lists, requires prior 1. To provide an understanding of caption coverage in specific permission and/or a fee. Request permissions 2017, we present an updated investigation into the alt from [email protected]. text coverage on 481 sites sampled from alexa.com’s list CHI 2018, April 21–26, 2018, Montreal, QC, Canada of the most popular websites [1] in ten categories. © 2018 Copyright is held by the owner/author(s). Publication rights licensed to ACM. ACM 978-1-4503-5620-6/18/04 $15.00 2. We developed Caption Crawler, a working prototype into three categories: machine-generated, human-generated, based on a browser plugin that allows users to browse and hybrid approaches. their favorite websites while image captions from other Machine-Generated Captions pages with the same image are dynamically loaded into Recent advances in computer vision through deep learning, the browser in the background. In addition, if more than specifically vision-to-language systems such as [13, 33], one description is found, users can access the caption have enabled the creation of automatically-generated queue to gain additional details from other captions captions. For instance, the technology described in [13] led retrieved for an image. to Microsoft’s CaptionBot API [10], which generates 3. To test the performance, utility, and usability of our natural-language descriptions of images. However, studies of system, we provide results from both automated system the output of vision-to-language systems indicate that they tests and user tests with both sighted and visually are still too error prone to be reliable for people who are VI impaired (VI) users. We found that automated vision-to- [20, 29]. Facebook’s Automated Alternative Text system language techniques such as CaptionBot [10] performed uses a more conservative approach to harnessing the output worse than alt text found on the web, and that our of computer vision by generating a series of tags that apply Caption Crawler system was able to significantly reduce to an image with high probability (e.g., “This image may the number of uncaptioned images in a page. We also contain the sun, people, the ocean.”) [37]. found that users enjoyed having and using the caption queue to gain a broader understanding of image content Telleen-Lawton et al. [32] wrote about the use of content- with additional captions. based image search for image retrieval. They presented a series of models along with descriptive user scenarios and RELATED WORK made suggestions about the types of performance metrics Image Accessibility on the Web that were important in each context. Keysers et al. developed Ramnath et al.’s recent analysis of social image sharing a system to automatically add alternative text to images platforms shows a near exponential growth of photo based on previously stored images found to be similar. The uploading and hosting on the web. The authors highlight that system used content similarity measures to find a set of relying on human annotators to caption the web will likely visually similar images that contained labels in their not be able to keep up with the rise in photos online, and database. The system then used these labels to predict propose an automated image captioning system as a scalable possible descriptions for the unlabeled image and could give solution [26]. Morris et al. surveyed blind Twitter users to simple labels such as the color information, as well as the investigate how their use, needs, and goals with the platform presence of people in the photo and their location [17]. have evolved over the years [22]. This work and studies of Similarly, Zhang et al [38] used visually similar image blind users on Facebook [35, 37] demonstrate the interest in results to build a set of words or tags associated with the social media of blind users, but also highlight access issues image. They then used this set of tags in a search query, in the platforms and a trend toward these media becoming expanding the original set of tags with commonly used words increasingly image-centric. found in the resulting set of documents after the text search Bigham et al. examined both sighted and blind users’ query had been run. The expanded set of tags was then browsing activities over the course of a week for a real-world associated with the image and could be used to supply view at the behaviors and accessibility issues related to additional context. general browsing. The study demonstrated that about half of Elzer et al. created a system that automatically gave all images contained alt text on the original source, and that descriptions for bar graphs for VI users in their web blind individuals were more likely to click on an image that browsing. This system used computer vision to determine contained descriptive alt text [3]. In general, studies of high-level descriptions including the plot title and trends of consumer and government websites repeatedly find high the data [12]. levels of non-compliance in providing alt text descriptions Human-Generated Captions for online images, with high proportions of descriptions that Von Ahn et al. first introduced the idea of human-powered are missing completely or of very poor quality (e.g., captioning, using the ESP game to motivate online workers filenames, the word “image”) [14, 18, 19, 23, 25].
Details
-
File Typepdf
-
Upload Time-
-
Content LanguagesEnglish
-
Upload UserAnonymous/Not logged-in
-
File Pages11 Page
-
File Size-