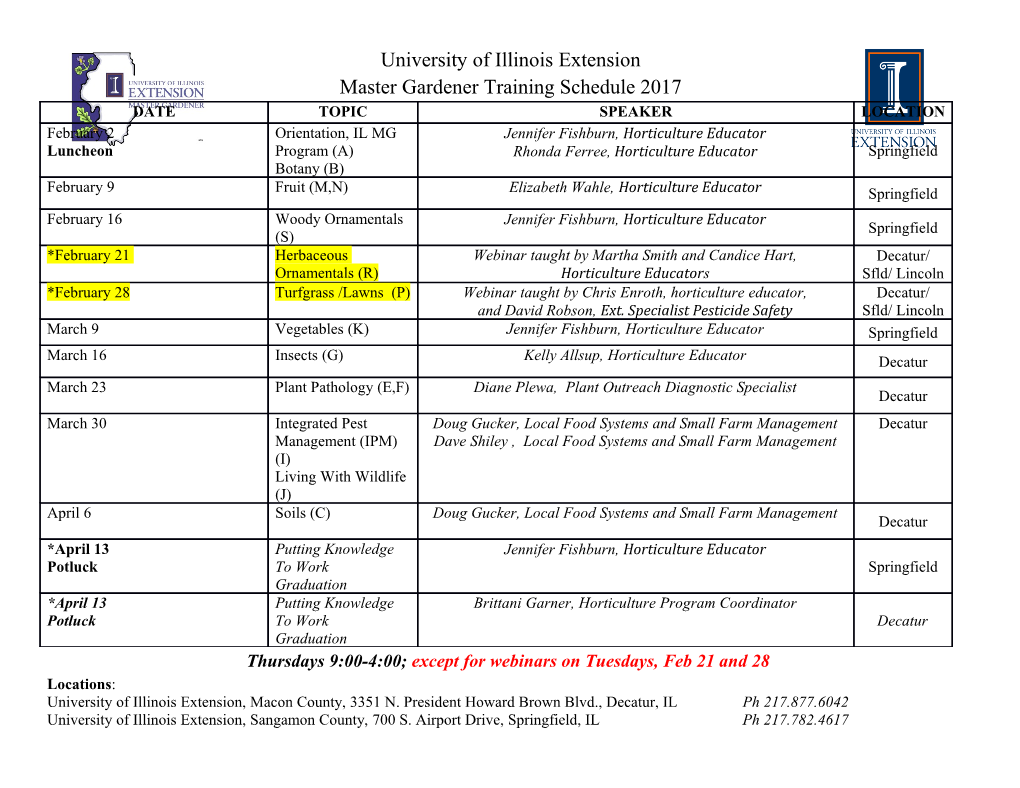
Genetics and Molecular Biology, 23, 4, 739-743 (2000)Null expectations of spatial correlograms 739 METHODOLOGY Null expectation of spatial correlograms under a stochastic process of genetic divergence with small sample sizes Mariana Pires de Campos Telles1,3 and José Alexandre Felizola Diniz-Filho2 Abstract An Ornstein-Uhlenbeck process was used to simulate the exponential relationship between genetic divergence and geographic distances, as predicted by stochastic processes of population differentiation, such as isolation-by-distance, stepping-stone or coalescence models. These simulations were based only on the spatial coordinates of the local populations that defined a spatial unweighted pair-group method using arithmetic averages (UPGMA) link among them. The simulated gene frequency surfaces were then analyzed using spatial autocorrelation procedures and Nei’s genetic distances, constructed with different numbers of variables (gene frequencies). Stochastic divergence in space produced strong spatial patterns at univariate and mutivariate levels. Using a relatively small number of local populations, the correlogram profiles varied considerably, with Manhattan distances greater than those defined by other simulation studies. This method allows one to establish a range of correlogram profiles under the same stochastic process of spatial divergence, thereby avoiding the use of unnecessary explanations of genetic divergence based on other microevolutionary processes. INTRODUCTION tionship also holds for coalescence models, in which the main determinant of divergence is genetic drift within lo- Patterns and processes of genetic divergence among cal populations, that accumulates variance through time and local populations have recently been investigated using not through spatially structured gene flow (Nielsen et al., spatial correlograms to describe autocorrelation of gene 1998; Avise, 2000). frequencies for increasing classes of spatial distance (Sokal Sokal and Wartenberg (1983) showed that isolation- and Jacquez, 1991). Simulations have shown that this tech- by-distance correlograms have similar profiles, with an nique is a powerful tool for studying the microevolution- exponential decrease between autocorrelation and geo- ary processes of genetic divergence (Sokal and Wartenberg, graphic distances, despite the low correlation among sur- 1981; Sokal et al., 1989, 1997; Epperson, 1995a,b). faces. Testing the similarity of correlograms and compari- One of the random processes most widely used to son of the results with correlations among gene frequen- describe the present genetic divergence among local popu- cies are then analogous to the Lewontin-Krakauer test of lations is isolation-by-distance (Wright, 1943) and its dis- neutrality based on the homogeneity of the FST statistics continuous version, the stepping-stone model (Kimura and (Kimura, 1983; Barbujani, 1987). Sokal and Wartenberg Weiss, 1964). The basic isolation-by-distance model is (1983) and Sokal (1986) proposed that the same stochas- usually modeled in a unidimensional distribution space tic spatial process could generate correlograms with Man- through the Morton-Malecot equation hattan distances of less than 0.1 or 0.2 (produced using uncorrelated gene frequencies). However, a critical aspect φ (d) = ac -bd of this proposal is that if the sample size (number of lo- cal populations) used to construct the correlograms is where φ (d) is the “kinship” between populations located at small, their profiles can vary considerably, even if they a geographic distance d, and a and b are constants that can were generated by the same stochastic process of popu- be interpreted as a function of the demographic and ge- lation divergence. netic characteristics of the populations (Wijsman and In this paper, we used an Ornstein-Uhlenbenck (O-U) Cavalli-Sforza, 1984). In both models, a negative exponen- stochastic process to simulate exponential relationships tial relationship between genetic similarity and spatial dis- between genetic divergence and geographic distance, in a tance is generated by drift in local populations and is con- manner analogous to isolation-by-distance, stepping-stone strained by migration effects at short distances. This rela- or coalescence models. For a specified sample size and 1Programa de Pós-graduação em Agronomia, Escola de Agronomia, Universidade Federal de Goiás, Caixa Postal 131, 74001-970 Goiânia, GO, Brasil. E-mail: [email protected] 2Departamento de Biologia Geral, ICB, Universidade Federal de Goiás, Caixa Postal 131, 74001-970 Goiânia, GO, Brasil. 3Departamento de Zootecnia, Universidade Católica de Goiás, Av. Universitária, 1440, Caixa Postal 86, 74605-010 Goiânia, GO, Brasil. Send correspondence to J.A.F.D.-F. E-mail: [email protected] 740 Telles and Diniz-Filho spatial distribution of local populations, this relatively population differentiation. In the O-U process, the evolu- simple and general method allows definition of the null tionary changes were constrained by a constant force to- expectation of variation for correlograms under a simple ward a central point (the adaptive peak of p), and the stochastic process of spatial divergence among local popu- pairwise covariance among local populations (VB, equiva- lations. lent to φ (d)) was related to distance (d) by the exponential relationship MATERIAL AND METHODS σ2 α -2αd ε VB = /2 (1 - e ) + We simulated gene frequencies for 13 local popula- tions distributed geographically as shown in Figure 1. These where σ2 is the variance of evolutionary change under a sto- localities were used in an isozyme study of Eugenia chastic process, and α is the restraining force. The vari- dysenterica DC (Myrtaceae) population genetics in a re- ance of evolutionary changes per unit of time (or space) in gion of the Brazilian “cerrado” (Telles, 2000), and served the simulations indicates the range of deviations of p from to illustrate the procedure developed here. Simulations its “ancestral” value, and is a function of the variance of p were performed with the PDAP software (phenotypic di- (see below) and of the maximum distance between pairs of versity analysis program - Díaz-Uriarte and Garland, 1996) local populations (dmax). Following Díaz-Uriarte and Gar- α based on an unweighted pair-group method using arithmetic land (1996), the restraining force was set to 2/dmax, in averages (UPGMA) clustering of geographic distances order to produce a moderate exponential relationship be- (Sneath and Sokal, 1973), designed to represent the tween VB and geographic distance. There is an equivalence pairwise distances among local populations in a tree for- between the previously defined Malecót-Morton equation mat. The cophenetic correlation of clustering was very high and the O-U process, with the constants a and b being ex- (r = 0.96), which ensured that running simulations with pressed in terms of σ2, α and e. PDAP across this tree simulated a spatial diffusion pro- Since the O-U process “forgets” past events (in this cess. Thus, the branch lengths of UPGMA based on geo- case, long-range spatial patterns), it reduces the level of graphic distances must be interpreted as a parameter re- “spatial inertia” in the data. At each instant of divergence in lated to the expected amount of gene flow among local time or space, the stochastic variation in p can be pushed populations. On the other hand, if one wishes to simulate a to the adaptive peak if the value of gene frequencies be- spatially structured coalescence model (Avise, 2000), these comes too high or too low, which explains the exponential branch lengths are a direct estimate of the divergence time decrease of genetic divergence when distantly related popu- between local populations. lations are compared (Felsenstein, 1988). The assumption An O-U stochastic process (Felsenstein, 1988; Mar- of a constant population size through time, present in most tins, 1995; Hansen and Martins, 1996) was then used to models of population differentiation, is approximated in generate 500 distributions of gene frequencies p maintain- the O-U model used here by the constant variance in evo- ing an exponential relationship between genetic divergence lutionary changes throughout the process. and geographic distances represented in UPGMA cluster- All simulations started with an initial gene frequency ing, as expected by the Malecót-Morton equation for iso- (the adaptive peak) of 0.5 ± 0.04, so that most values of lation-by-distance and by other stochastic processes of gene frequencies would fall between zero and 1.0 (values of p assume a normal distribution). However, since PDAP was originally developed to generate variation in continu- ous traits, we also used the boundary option of the pro- -15.8 gram to constrain the variation between zero and 1.0 (the -16.2 “replace” algorithm; Díaz-Uriarte and Garland, 1996). Each gene frequency vector simulated represented -16.6 surfaces of variation in geographic space (sensu Sokal and -17.0 Oden, 1978a,b), and was analyzed and interpreted using stan- -17.4 dard procedures of spatial autocorrelation (Sokal and Oden, 1978a,b; Sokal and Jacquez, 1991). Moran’s I coefficients Latitude (south) -17.8 were estimated in four geographic distance classes in or- -18.2 der to generate the spatial correlograms. A Pearson corre- -18.6 lation coefficient was calculated between Moran’s I values -49.8 -49.4 -49.0 -48.6 -48.2 -47.8 -47.4 -47.0 and the upper limit of the distance class in order to evalu- Longitude (west) ate the linearity of the decrease
Details
-
File Typepdf
-
Upload Time-
-
Content LanguagesEnglish
-
Upload UserAnonymous/Not logged-in
-
File Pages6 Page
-
File Size-