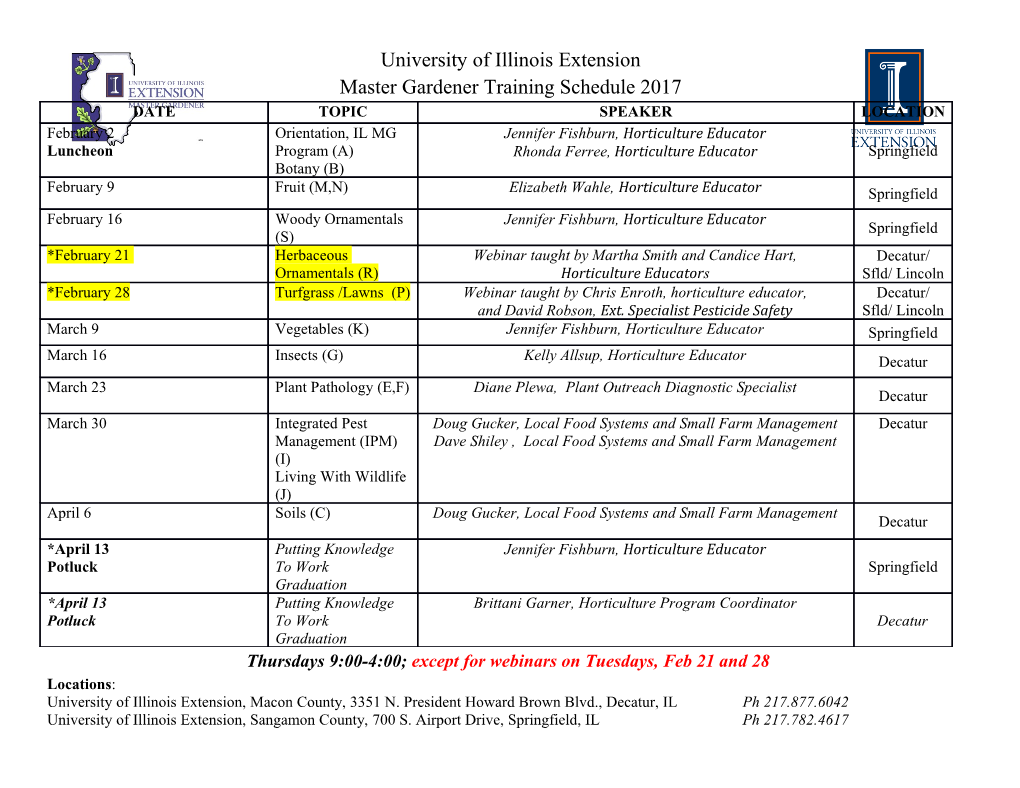
Computational Visual Media DOI 10.1007/s41095-016-0059-z Vol. 2, No. 4, December 2016, 379–388 Research Article LSTM-in-LSTM for generating long descriptions of images Jun Song1, Siliang Tang1, Jun Xiao1, Fei Wu1( ), and Zhongfei (Mark) Zhang2 c The Author(s) 2016. This article is published with open access at Springerlink.com Abstract In this paper, we propose an approach by means of text (description generation) is a for generating rich fine-grained textual descriptions of fundamental task in artificial intelligence, with many images. In particular, we use an LSTM-in-LSTM (long applications. For example, generating descriptions short-term memory) architecture, which consists of an of images may help visually impaired people better inner LSTM and an outer LSTM. The inner LSTM understand the content of images and retrieve images effectively encodes the long-range implicit contextual using descriptive texts. The challenge of description interaction between visual cues (i.e., the spatially- generation lies in appropriately developing a model concurrent visual objects), while the outer LSTM that can effectively represent the visual cues in generally captures the explicit multi-modal relationship images and describe them in the domain of natural between sentences and images (i.e., the correspondence language at the same time. of sentences and images). This architecture is capable There have been significant advances in of producing a long description by predicting one description generation recently. Some efforts word at every time step conditioned on the previously rely on manually-predefined visual concepts and generated word, a hidden vector (via the outer LSTM), and a context vector of fine-grained visual cues (via sentence templates [1–3]. However, an effective the inner LSTM). Our model outperforms state-of-the- image description model should be free of hard art methods on several benchmark datasets (Flickr8k, coded templates and categories. Other efforts Flickr30k, MSCOCO) when used to generate long rich treat the image description task as a multi-modal fine-grained descriptions of given images in terms of retrieval problem (e.g., image–query–text) [4–7]. four different metrics (BLEU, CIDEr, ROUGE-L, and Such methods obtain a descriptive sentence of each METEOR). image by retrieving similarly described images from a large database and then modifying these retrieved Keywords long short-term memory (LSTM); image descriptions based on the query image. Such description generation; computer vision; neural network methods lack the ability to generate descriptions of unseen images. Motivated by recent successes in computer vision 1 Introduction and natural language processing, current image description generation approaches generate more Automatically describing the content of an image reasonable descriptive sentences of given images [8– 10] based on an approach of word-by-word generation 1 College of Computer Science and Technology, Zhejiang via recurrent neural networks (RNN) (e.g., using University, Hangzhou 310027, China. E-mail: J. long short-term memory (LSTM)) since these Song, [email protected]; S. Tang, siliang@cs. approaches store context information in a recurrent zju.edu.cn; J. Xiao, [email protected]; F. Wu, wufei@ layer. Most description generation research only cs.zju.edu.cn ( ). utilizes the image being described to the RNN at the 2 Department of Computer Science, Watson School beginning [10]. By looking at the image only once of Engineering and Applied Sciences, Binghamton University, Binghamton, NY, USA. E-mail: zhongfei@ during word-by-word generation, the precision and cs.binghamton.edu. recall of the predicted noun words (i.e., visual objects Manuscript received: 2016-07-25; accepted: 2016-08-19 in images) decrease rapidly with their position of 379 380 J. Song, S. Tang, J. Xiao, et al. Prediction a boy is #END# ... p0 p1 p2 pT The whole image Decoder Decoder Decoder Decoder Image ... feature LSTM-in-LSTM LSTM-in-LSTM LSTM-in-LSTM LSTM-in-LSTM ... ... Encoder Encoder Encoder Encoder ... One image and its The fine-grained cues w0 w1 w2 wT fine-grained regions DeepCNN Region features #START# a boy monitor Fig. 1 Overview of our approach. The DeepCNN model projects the pixels of an image and its fine-grained regions into a 4096-dimensional feature. The encoder layer encodes the textual words, the whole image, and the visual objects as vectors. The prediction layer outputs one hidden vector at each step which is then used to predict the next word in the decoder layer. While training, the tth word in the sentence is fed into the model to predict the next word (solid lines). While testing, the word predicted at the previous step (t − 1) is fed into the model at step t. occurrence in a sentence (as shown in Fig. 5), since a hidden vector (via the outer LSTM), and the these approaches merely preserve global semantics context vector of fine-grained visual cues (via the at the beginning and disregard the fine-grained inner LSTM). interactions between visual cues which could be Compared with existing methods, the proposed useful if we wish to generate richer, more descriptive LSTM-in-LSTM architecture, as illustrated in Fig. captions. 1, is particularly appropriate for generating rich fine- From the point of view of the mutual utilization of grained long descriptions with appealing diversity, visual and textual contexts during each step of word- owing to its modeling of long-range interactions by-word generation, image description generation between visual cues. methods may in general be categorized into two classes. The first class repeatedly takes advantage 2 Related work of the whole image at each time step of the output word sequence [9]. Such methods may identify the 2.1 Natural language models most interesting salient objects the words refer to; Over the last few years, natural language models however, they may still ignore the fine-detail objects. based on neural networks have been widely used in The second class explicitly learns the the natural language processing domain. Artificial correspondences between visual objects (detected neural networks have been employed to learn a as object-like or regions of attention) and the distributed representation for words which better matching words at each step of generation, captures the semantics of words [13]. Recursive and then generates the next word according to neural networks have been used to encode a natural both the correspondences and the LSTM hidden language sentence as a vector [7]. Palangi et al. [14] vector [11, 12]. Such methods may neglect long- use a recurrent neural network (RNN) with long range interactions between visual cues (e.g., the short-term memory (LSTM) to sequentially take spatially-concurrent visual objects). each word in a sentence, and encode it as a semantic In this paper, we develop a new neural network vector. A recurrent neural network encoder–decoder structure called LSTM-in-LSTM (long short-term architecture has been proposed to encode a source memory) which can generate semantically rich and language sentence, and then decode it into a target descriptive sentences for given images. The LSTM- language [15]. in-LSTM consists of an inner LSTM (encoding 2.2 Deep model for computer vision the implicit long-range interactions between visual cues) and an outer LSTM (capturing the explicit Methods based on deep neural networks have been multi-modal correspondences between images and adopted by a large number of computer vision sentences). This architecture is capable of producing applications. Deep convolutional neural networks a description by predicting one word at each time (DeepCNN) have achieved excellent performance step conditioned on the previously generated word, in image classification tasks (e.g., AlexNet [16], LSTM-in-LSTM for generating long descriptions of images 381 VggNet [17]). Object detection systems based on a as a one-hot representation w1, w2, ··· , wT . This well trained DeepCNN outperform previous works representation is a binary representation which has (RCNN [18], SPPNet [19]). Girshick [20] proposed the same dimension as the vocabulary size and Fast-RCNN which is much faster than RCNN and only one non-zero element. After that, the one-hot SPPNet for object detection during both training representation is transformed into an h-dimensional and testing. vector as follows: 2.3 Image descriptions ωt = Wswt (1) W is a matrix of size h × V , where V is the size There are two main categories of methods for s of the vocabulary. W is randomly initialized and automatically describing an image: retrieval based s learned during the model training. methods and generation based methods. Many For images, we use Fast-RCNN [20] to detect the works try to describe an image by retrieving a visual objects in the image. Fast-RCNN is a fast relevant sentence from a database. They learn framework for object detection based on a deep the co-embedding of images and sentences in a convolutional neural network. This framework is common vector space and then descriptions are trained using a multi-task loss function in a single retrieved which lie close to the image in the training stage, which not only simplifies learning but embedding space [4, 5, 7]. Karpathy et al. [21] also improves the detection accuracy. argue that by using a correspondence model that A threshold τ is set to select the valid visual is based on a combination of image regions and objects from all objects detected by Fast-RCNN. phrases of sentences, the performance of retrieval Visual objects with a detection score higher than τ based image description methods can be boosted. are considered as valid visual objects; the rest are Generation based methods often use fixed templates discarded. The number of the valid objects may be or generative grammars [22]. Other generation different in each image. methods more closely related to our method learn For each image I and each visual object r, we the probability distribution of the next word in a first obtain their 4096-dimensional VGGNet16 [17] sentence based on all previously generated words [8– fc7 features.
Details
-
File Typepdf
-
Upload Time-
-
Content LanguagesEnglish
-
Upload UserAnonymous/Not logged-in
-
File Pages10 Page
-
File Size-