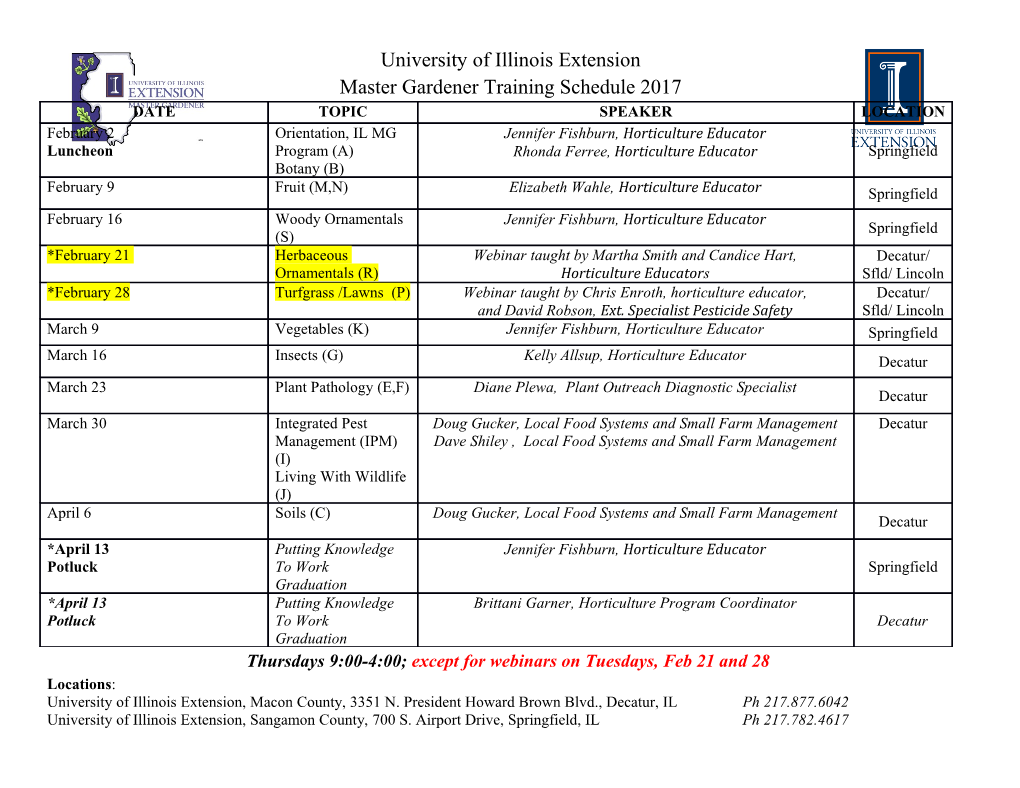
computers and electronics in agriculture 61 (2008) 63–78 available at www.sciencedirect.com journal homepage: www.elsevier.com/locate/compag Autonomous robotic weed control systems: A review D.C. Slaughter ∗, D.K. Giles, D. Downey University of California, Biological and Agricultural Engineering, Davis, CA 95616, United States article info abstract Article history: Autonomous robotic weed control systems hold promise toward the automation of one of Received in revised form agriculture’s few remaining unmechanized and drudging tasks, hand weed control. Robotic 22 March 2007 technology may also provide a means of reducing agriculture’s current dependency on her- Accepted 2 May 2007 bicides, improving its sustainability and reducing its environmental impact. This review describes the current status of the four core technologies (guidance, detection and identifica- Keywords: tion, precision in-row weed control, and mapping) required for the successful development Robot of a general-purpose robotic system for weed control. Of the four, detection and identifica- Weed recognition tion of weeds under the wide range of conditions common to agricultural fields remains the Machine vision greatest challenge. A few complete robotic weed control systems have demonstrated the Geospatial technology potential of the technology in the field. Additional research and development is needed to Pest management fully realize this potential. Precision agriculture © 2007 Elsevier B.V. All rights reserved. 1. Introduction ranth (Amaranthus spinosus L.) in lettuce fields reduced head weight and quality. Hodgson (1968) found that two Canada 1.1. Importance of weed control in crop production thistle (Cirsium arvense (L.) Scop.) shoots/m2 reduced wheat yields by 15%. The presence of weeds at harvest may inter- Weed control is a significant issue in agricultural crop produc- fere with some types of harvesting techniques or defoliation tion. Weeds compete with crop plants for moisture, nutrients of cotton. The presence of weed seed in the harvested grain or and sunlight and can have a detrimental impact on crop yields staining of cotton fiber by contact with weeds at harvest can and quality if uncontrolled. A number of studies have doc- reduce the quality and value of the crop. umented the yield loss associated with weed competition. A number of factors affect the magnitude of yield and qual- Monaco et al. (1981) reported 71%, 67%, 48%, and 48% yield ity loss including, competitiveness of crop and weeds present, reductions in direct-seeded tomato when jimsonweed (Datura density of crop and weed plants, time of emergence of the stramonium L.), tall morning glory (Ipomoea L.), common cock- weeds relative to the crop, duration of the weed presence, and lebur (Xanthium strumarium L.), and large crabgrass (Digitaria proximity of the weeds relative to the crop plants (Weiner, sanguinalis (L.) Scop.), respectively, were present in the row at 1982; Pike et al., 1990). For example, Keeley and Thullen (1989, a density of 11 weed plants/m2. Roberts et al. (1977) found 1991) found that when Johnsongrass (Sorghum halepense L.) or that season-long competition from mixed stands of grass and barnyardgrass (Echinochloa crus-galli L.) were allowed to com- broadleaf weeds at 65 weeds/m2 resulted in complete loss of pete with cotton during the first 9 weeks after emergence, marketable lettuce in field studies in England. Lanini and Le and then the fields were kept weed-free for the remainder Strange (1991) found that season-long weed competition in of the season, yield losses of 60% and 69%, respectively were California lettuce fields reduced lettuce yields by over 50% and observed. Heisel et al. (2002) studied the effect of weed (Sinapis Shrefler et al. (1996) found that competition from spiny ama- arvensis L. or Lolium perenne L.) proximity (2, 4 or 8 cm from the ∗ Corresponding author. Tel.: +1 530 752 5553; fax: +1 530 752 2640. E-mail address: [email protected] (D.C. Slaughter). 0168-1699/$ – see front matter © 2007 Elsevier B.V. All rights reserved. doi:10.1016/j.compag.2007.05.008 64 computers and electronics in agriculture 61 (2008) 63–78 crop) on yield loss in sugar beet. The yield increased an average machine vision), weed detection and identification (machine of 20% (independent of weed species) as the distance between vision, hyperspectral imaging, possibly assisted by RTK GPS), the weed and crop increased from 2 to 8 cm. These factors have precision in-row weed control (micro-spray, cutting, thermal, a direct impact on weed control: the weeds that are most diffi- electrocution), and mapping (GPS & machine vision). cult to remove with a hoe, for example, are also those that are most detrimental to crop yield, weeds that emerge just prior 2. Core technologies for autonomous to or with crop emergence (assuming direct-seeded crops) are robotic weed control in row crops the most critical to remove, and infestations where there are large numbers of weeds relative to the crop (e.g. when crop germination is poor) are most harmful to yield. 2.1. Row guidance systems Currently, for row crops, typical weed control methods include a combination of preemergence herbicide application 2.1.1. Machine vision-based automatic row guidance and/or preemergence tillage, mechanical cultivation, poste- After decades of research and development, row crop guidance mergence herbicide application (if selective herbicides or crop has achieved a high level of automation and some com- resistance is available) and hand hoeing. While herbicide- mercial success. While many guidance-sensing technologies based weed control may be both biologically efficacious and have been attempted (e.g., Jahns, 1983; Tillett, 1991) two types economically effective, in many situations it is not without of sensors have achieved the greatest commercial success: environmental costs. In many locations, increasing pesticide machine vision and global positioning systems. Astrand˚ (2005) use regulations, consumer concerns, and a growing interest lists the following requirements for row guidance systems: in organically produced foods limit the long-term acceptabil- ity of herbicide application. When selective postemergence • Ability to track rows with an accuracy of a few centimeters. herbicides are unavailable or ineffective, hoeing of “in-row” • Ability to control a row cultivator and an autonomous agri- weeds is required. However, in-row hand hoeing is costly, i.e., cultural robot in real-time, which means that both heading over five times more expensive than conventional cultiva- and offset of the row structure must be estimated at a suf- tion (Chandler and Cooke, 1992) and not completely effective. ficient[ly] fast rate. Vargas et al. (1996) observed that hand-hoeing crews mis- • Ability to work on sown crops, not planted, which means take weeds for crop plants or miss weeds, eliminating only that the time of emergence and the size of the crops can about 65–85% of the weeds depending upon the level of field vary in the field. This also means that crops and weeds have supervision and the similarity in appearance between the crop about the same size in early cultivation, i.e. discrimination and the weeds. Weed infestations are often distributed non- between crops and weed cannot be made by size only. uniformly in agricultural fields. The non-uniformity in weed • Ability to work when there is high weed pressure, up to populations has both temporal and spatial aspects that pro- 200 weeds/m2. vide an opportunity for the application of site-specific weed • Ability to work when plants are missing in the row. This is control technology to reduce environmental and economic especially the case in [organic] fields where emergence is costs associated with weed control. Thompson et al. (1991) about 70%, which is less than in conventional fields, where concluded that although the technology for tractor-mounted the emergence usually is about 90%. real-time weed detection and control did not exist, the concept of automated selective spraying of weeds in agricultural fields Vision systems require a guidance directrix (guiding land- had great potential for reducing economic and environmental mark) and typically use a forward-looking view of the crop costs while maintaining a high level of weed control. rows (Fig. 2). One of the most commonly used machine vision meth- 1.2. General system design and architecture for ods for identifying crop rows is based upon the Hough (1962) automatic weed control transform. The Hough transform is a computationally efficient procedure for detecting discontinuous lines or curves in pic- Harrell et al. (1988) observed that an agricultural robot incor- tures, thus it is suited for situations like those listed by Astrand˚ porates three basic components: “(1) a sensing system to (2005) where the crop stand is incomplete with gaps in the measure important physical and biological properties of the crop rows due to poor germination, insect damage or other agricultural system; (2) decision-making capabilities for pro- factors that result in missing crop plants in the row (Duda and cessing information from the sensor system to determine Hart, 1972). This technique was proposed by Reid and Searcy how the agricultural system should be manipulated; and (3) (1986) to facilitate row identification for agricultural guidance actuators to manipulate the agricultural system accordingly.” and was employed by Fujii and Hayashi (1989) in their U.S. Technologies such as machine vision analysis, global position- patent for automatic guidance of a combine harvester. In one ing systems, variable rate application techniques, and robotics of the early implementations, Marchant and Brivot (1995) used provide the technological tools to allow robotic weed con- the Hough transform for row tracking in real-time (10 Hz) and trol to be feasible. This paper will review the current status noted that their technique was tolerant to outliers (i.e. weeds) of core technologies required for successful implementation only when the number of outliers was reasonably small com- of an autonomous mobile agricultural robot for weed control pared to the number of true data points.
Details
-
File Typepdf
-
Upload Time-
-
Content LanguagesEnglish
-
Upload UserAnonymous/Not logged-in
-
File Pages16 Page
-
File Size-