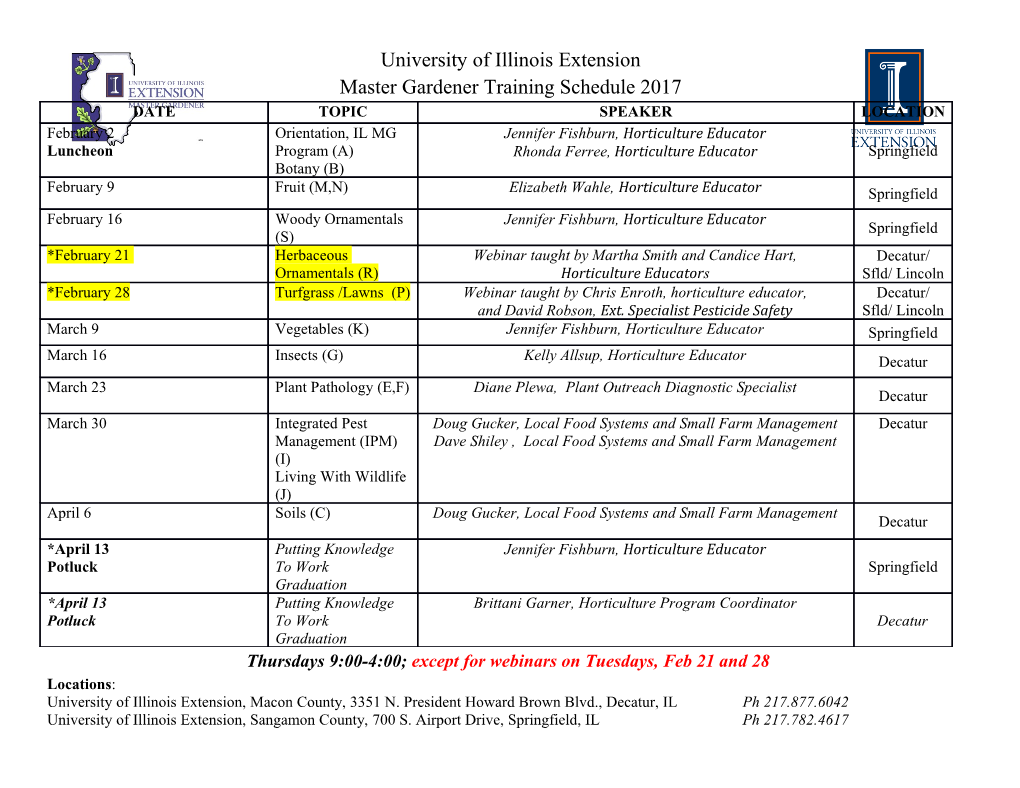
Time series Class Notes Manuel Arellano Revised: February 12, 2018 1 Time series as stochastic outcomes T A time series is a sequence of data points wt observed over time, typically at equally spaced f gt=1 intervals; for example, the quarterly GDP per capita or the daily number of tweets that mention a specific product. We wish to discuss probabilistic models that regard the observed time series as a realization of a probability distribution function f (w1, ..., wT ). In a random sample observations T are independent and identically distributed so that f (w1, ..., wT ) = t=1 f (wt). In a time series, observations near in time tend to be more similar, in which case the independenceQ assumption is not appropriate. Moreover, the level or other features of the series often change over time, and in that case the assumption of identically distributed observations is not appropriate either. Thus, the joint distribution of the data may differ from the product of marginal distributions of each data point f (w1, ..., wT ) = f1 (w1) f2 (w2) ... fT (wT ) 6 and the form of those marginal distributions may change over time. Due to the natural temporal ordering of data, a factorization that will be often useful is T f (w1, ..., wT ) = f1 (w1) ft (wt wt 1, ..., w1) . t=2 j Y If the distributions f1 (.), f2 (.), ... changed arbitrarily there would be no regularities on which to base their statistical analysis, since we only have one observation on each distribution. Similarly, if the joint distributions of consecutive pairs of observations changed arbitrarily, there would be no regularities on which to base the analysis of their dependence. Thus, modeling the dependence among observations and their evolving pattern are central to time series analysis. The basic building block to facilitate statistical analysis of time series is some stationary form of dependence that preserves the assumption of identically distributed observations. First we will introduce the concept of stationary dependence and later on we will discuss ways of introducing nonstationarities. Stochastic process A stochastic process is a collection of random variables that are indexed with respect to the elements in a set of indices. The set may be finite or infinite and contain integer or real numbers. Integer numbers may be equidistant or irregularly spaced. In the case of our time series the set is 1, 2, 3, ..., T , but usually it is convenient to consider a set of indices t covering all f g integers from to + . In such case we are dealing with a double-infinite sequence of the form 1 1 wt t1= = ..., w 1, w0, w1, w2, ..., wT , wT +1, ... , f g 1 f g 1 which is regarded as a single realization of the stochastic process, and the observed time series is a portion of this realization. Hypothetical repeated realizations of the process could be indexed as: (1) (2) (n) 1 wt , wt , ..., wt . t= n o 1 2 Stationarity A process wt is (strictly) stationary if the joint distribution of wt , wt , ..., wt for a given subset of f g f 1 2 k g indices t1, t2, ..., tk is equal to the joint distribution of wt +j, wt +j, ..., wt +j for any j > 0. That is, f 1 2 k g the distribution of a collection of time points only depends on how far apart they are, and not where they start: f (wt1 , wt2 , ..., wtk ) = f (wt1+j, wt2+j, ..., wtk+j) . This implies that marginal distributions are all equal, that the joint distribution of any pair of variables only depends on the time interval between them, and so on: fwt (.) = fws (.) for all t, s fwt,ws (., .) = fwt+j ,ws+j (., .) for all t, s, j. 2 In terms of moments, the implication is that the unconditional mean and variance t, t of the distri- bution fwt (.) are constant: 2 2 E (wt) = µ, V ar (wt) = . t t Moreover, the covariance between wt and ws only depends on t s : t,s j j Cov (wt, ws) t,s = t s . j j 2 Thus, using the notation 0 = , the covariance matrix of w = (w1, ..., wT ) takes the form 0 1 . T 1 0 1 0 1 . T 2 1 . V ar (w) = B . .. C . B 1 0 C B . C B .. C B T 2 1 C B C B T 1 T 2 . 0 C B C @ A Similarly, the correlation between wt and ws only depends on t s : t,s j j t,s t,s = t s ts j j The quantity j is called the autocorrelation of order j and when seen as a function of j it is called the autocorrelation function. 2 A stationary process is also called strictly stationary in contrast with weaker forms of stationarity. For example, we talk of stationarity in mean if t = or of covariance stationarity (or weak station- arity) if the process is stationary in mean, variance and covariances. In a normal process covariance stationarity is equivalent to strict stationarity. Processes that are uncorrelated or independent A sequence of serially uncorrelated random variables with zero mean and constant finite variance is called a “white noise”process; that is, white noise is a covariance stationary process wt such that E (wt) = 0 V ar (wt) = < 0 1 Cov (wt, wt j) = 0 for all j = 0. 6 In this process observations are uncorrelated but not necessarily independent. In an independent white noise process wt is also statistically independent of past observations: f (wt wt 1, wt 2, ...w1) = f (wt) . j Another possibility is a mean independent white noise process that satisfies E (wt wt 1, wt 2, ...w1) = 0. j In this case wt is called a martingale difference sequence. A martingale difference is a stronger form of independence than uncorrelatedness but weaker than statistical independence. For example, a martin- 2 gale difference does not rule out the possibility that E wt wt 1, ...w1 depends on past observations. j Prediction Consider the problem of selecting a predictor of wt given a set of past values wt 1, ..., wt j . The conditional mean E (wt wt 1, ..., wt j) is the best predictor when the loss func- f g j tion is quadratic. Similarly, the linear projection E (wt wt 1, ..., wt j) is the best linear predictor j under quadratic loss. For example, for a stationary process wt E (wt wt 1) = + wt 1 j with = /γ and = (1 ) . We can also write 1 0 wt = + wt 1 + t where t is the prediction error, which by construction is orthogonal to wt 1. For convenience, predictors based on all past history are often considered: Et 1 (wt) = E (wt wt 1, wt 2, ...) j 3 or Et 1 (wt) = E (wt wt 1, wt 2, ...) , j which are defined as the corresponding quadratic-mean limits of predictors given wt 1, ..., wt j as f g j . ! 1 Linear predictor k-period-ahead Let wt be a stationary time series with zero mean and let ut denote the innovation in wt so that wt = Et 1 (wt) + ut. ut is a one-step-ahead forecast error that is orthogonal to all past values of the series. Similarly, wt+1 = Et (wt+1) + ut+1. Moreover, since the spaces spanned by (wt, wt 1, wt 2, ...) and (ut, wt 1, wt 2, ...) are the same, and ut is orthogonal to (wt 1, wt 2, ...) we have: Et (wt+1) = E (wt+1 ut, wt 1, wt 2, ...) = Et 1 (wt+1) + E (wt+1 ut) . j j Thus, E (wt+1 ut)+ut+1 is the two-step-ahead forecast error in wt+1. In a similar way we can obtain j incremental errors for Et 1 (wt+2) , ..., Et 1 (wt+k). Wold decomposition Letting E (wt+1 ut) = ut, we can write j 1 wt+1 = ut+1 + 1ut + Et 1 (wt+1) and repeating the argument we obtain the following representation of the process: wt = (ut + 1ut 1 + 2ut 2 + ...) + t where ut wt Et 1 (wt), ut 1 wt 1 Et 2 (wt 1), etc. and t denotes the linear prediction of wt at the beginning of the process. This representation is called the Wold decomposition, after the work of Herman Wold. It exists for any covariance stationary process with zero mean. The one-step-ahead 2 1 forecast errors ut are white noise and it can be shown that 1 < (with = 1). j=0 j 1 0 The term t is called the linearly deterministic part of wPt because it is perfectly predictable based on past observations of wt. The other part, consisting of j1=0 jut j, is the linearly indeterministic part of the process. The indeterministic part is the linearP projection of wt onto the current and past linear forecast errors, and the deterministic part is the corresponding projection error. If t = 0, wt is a purely non-deterministic process, also called a linearly regular process. 1 See T. Sargent, Macroeconomic Theory, 1979. 4 Ergodicity A stochastic process is ergodic if it has the same behavior averaged over time as averaged over the sample space. Specifically, a covariance stationary process is ergodic in mean if the time series mean converges in probability to the same limit as a (hypothetical) cross-sectional mean (known as the ensemble average), that is, to E (wt) = : 1 T p wT = wt µ. T t=1 ! P Ergodicity requires that the autocovariances j tend to zero suffi ciently fast as j increases. In the next section we check that wt is ergodic in mean if the following absolute summability condition is f g satisfied: 1 < .
Details
-
File Typepdf
-
Upload Time-
-
Content LanguagesEnglish
-
Upload UserAnonymous/Not logged-in
-
File Pages16 Page
-
File Size-