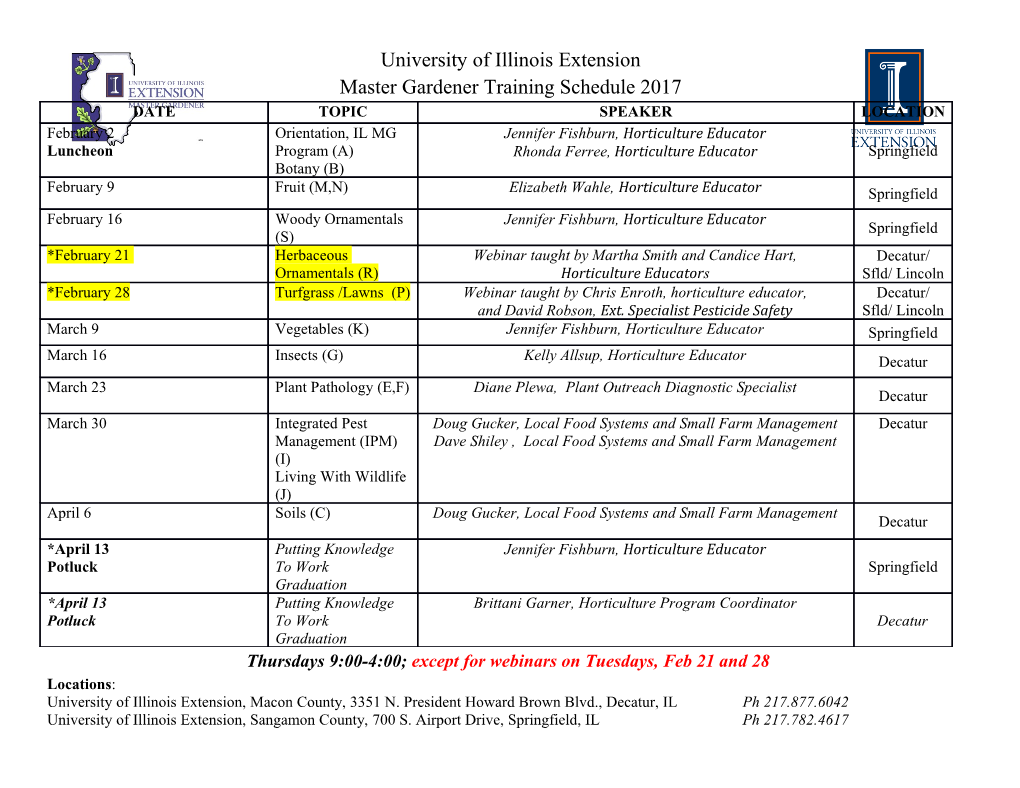
Japanese Journal of Biometrics Vol. 40, No. 2, 69 − 79 (2020) Original Article Reproducibility of statistical test results based on p-value Takashi Yanagawa Biostatistics Center Kurume University e-mail : [email protected] Reproducibility is the essence of a scientific research. Focusing on two-sample problems we discuss in this paper the reproducibility of statistical test results based on p-values. First, demonstrating large variability of p-values it is shown that p-values lack the reproducibility, in particular, if sample sizes are not enough. Second, a sample size formula is developed to assure the reproducibility probability of p-value at given level by assuming normal distributions with known variance. Finally, the sample size formula for the reproducibility in general framework is shown equivalent to the sample size formula that has been developed in the Neyman-Pearson type testing statistical hypothesis by employing the level of significance and size of power. Key words: Interquartile range, Median, Lower and upper quartiles, Neyman-Pearson type statistical test, Reproducibility probability 1.Introduction p-value was introduced by K. Pearson in his chi-square test (1900) and its use promoted by R. A. Fisher as a useful tool for statistical inference from data (Fisher, R. A. 1925). Fisher suggested looked into data to check whether the treatment effect existed if p-value ⩽ 0.05, but not to consider data anymore if p-value>0.05 (Ono, 2018). On the other hand, Neyman-and Pearson (1928) introduced a method of testing statistical hypotheses by establishing a null and alternative hypotheses, as well as introducng the first and second kind of errors, and by solving a mathematical problem of maximizing the power, which is defined as 1-probability of the second kind of error, keeping the probability of the first kind of error less than given level of significance. Fisher emphasized statistical inference, but Neyman-Pearson emphasized statistical decision; philosophical disputes between Fisher and Neyman on the difference of their ideas continued nearly 30 years until the death of Fisher in 1962. Apparently, their ways of testing are equivalently expressed as follows. Reject the null hypothesis if p-value ⩽ α, and hold to reject it if p-value > α, where α is called the level of significance. Neyman-Pearsonʼ s approach of testing statistical hypothesis is exclusively illustrated in mathematical statistics text books. We call it in this paper the Neyman-Pearson type test of Received February 2019. Revised June 2019. Accepted August 2019 70 Yanagawa testing statistical hypothesis. On the other hand p-value and statistical test based on p-value are exclusively illustrated in most biostatistics text books. The author of the present paper tried to bridge these two extremes by introducing the idea of reproducibility of statistical test results based on p-value in his booklet (Yanagawa, 2019), written in Japanese by giving no mathematical proof. The purpose of the present paper is to describe it mathematically with rigorous proof and also in English so that to convey our findings not only to Japanese but also to overseas professionals. Recently, after the publication of the booklet, Valentin Amrhein, Sander Greenland, Blake McShane and more than 800 signatories called for the entire concept of statistical significance to be abandoned (Amrhein, V., Greenland, S., McShane, B., 2019). I appreciate it the calling for give up of Neyman-Pearson type test and coming back to Fisherʼs statistical inference. If this is the case the ʻdetermination of sample sizesʼ, an important topic in designing scientific research that has been undertaken using the concept of statistical power in the framework of Neyman-Pearson type test, could drift on the air. However, it will be demonstrated in the present paper that the concept of reproducibility could replace it. To present our idea simply, we focus the discussion in this paper on two-sample problems consisting of treatment group of sample size n and control group of the same sample size; and letting Δ be the treatment effect, we consider testing statistical hypothesis H0 : Δ=0vs.H1 : Δ=δ (δ>0). p-value is defined in the set up by p=Pr (T ≥ t0 |H0), (1) where T is a test statistic and t0 is the observed value of T . As is seen in (1) p-value is a random variable. The distribution and density functions of p-value have been developed by several authors. We summarize it in section 2. Numerical evaluations of location and scale parameters of the distribution of p-value are given in section 3 to demonstrate the large variability of p-values. Also it is indicated in the section that statistical test results based on p-values lack the reproducibility, in particular, if sample sizes are not enough, because of the large variability of p-values. In section 4, by assuming two-sample normal disributions with known common variance, a sample size formula is developed to assure the reproducibility probability at given level. Finally in the section, the sample size formula for the reproducibility in general framework is shown equivalent to the sample size formula that has been developed in the Neyman-Pearson type test for testing statistical hypothesis by employing the level of significance and size of power. Jpn J Biomet Vol. 40, No. 2, 2020 Reproducibility of statistical test results based on p-value 71 2.Distribution of p-value 2.1 Distribution function and probability density function of p-value Suppose two-sample problems consisting of treatment group of sample size n and control group with the same sample size as the treatment group; and letting Δ be the treatment effect, consider testing statistical hypothesis H0 : Δ=0vs.H1 :Δ=δ (δ>0 ) with test statistic T . Let F0 and F1 be the probability distribution functions of test statistic T under H0 and H1, respectively. Distribution function and probability density function of p-value have been given by Hung, OʼNeil, Bauer and Kohne (1997); Sackrowitz and Samuel-Cahn (1999); and Donahue (1999). We represent them in the following proposition. PROPOSITION 2.1 (1) p-value follows a uniform distribution on (0,1) under H0.(Hung, OʼNeil, Bauer and Kohne, 1997). (2) Denote by Gp (x) and gp (x) the distribution function and probability density function of p- value under H1, respectively, then Gp (x) and gp (x) are given as follows. (Sackrowitz and Samuel- Cahn, 1999; Donahue, 1999). G (x) =1−F F (1−x) (0<x<1) , (2) f F (1−x) g (x) = (0<x<1) . (3) f F (1−x) −1 (3) The distribution function of F0 (1−p) under H1 is F1 (x). COROLLARY 2.1 Let X1, X2,..., Xn be independently and identically distributed (i. i. d) 2 random variables as normal distribution with mean 0 and variance σ ; and let Y1, Y2,..., Yn be i.i. d random variables as normal distribution with mean μ and variance σ2, where σ is assumed known and the treatment effect is expressed by δ=μ/σ.Furthermore, let the test statistic T be given by Y −X T= . (2σ/n) Then, it follows that (1) Gp (x) and gp (x) are given as follows, where Φ is the distribution function of the standard −1 normal distribution, Φ is its inverse, ϕ is its density function, and μn= n/2 δ. G (x) =1−Φ Φ (1−x) −μ (0<x<1) , φ Φ (1−x) −μ g (x) = (0<x<1) . (4) φ Φ (1−x) −1 (2) Φ (1−p) follows normal distribution N(μn, 1) under H1. Jpn J Biomet Vol. 40, No. 2, 2020 72 Yanagawa Figure. 1. Probability density function of p-value given in (4) when n = 25 and μ = 0.176. 2.2 Location and dispersion parameters of the distribution of p-value Probability density function given in (4) is illustrated in Figure 1 when n=25 and μ=0.176, taking ln(p)=loge (p) in the horizontal line. The figure indicates that the distribution of p-value is not symmetric and has long right tail under H1. Thus we shall employ the median (50 % point of the distribution function) as a location parameter and the interquartile range, 75 % point - 25 % point, as a dispersion parameter of the distribution function of p-value, as employed in Box and Whisker plot (Tukey, 1977). Putting q1=0.25,q2=0.50,q3=0.75, the lower quartile (25 % point), median and upper quartile (75 % point) of the distribution of p-value are given by ξi, i=1, 2, 3, respectively, satisfying Gp (ξi)=qi. (5) More precisely, they are given in the following proposition. PROPOSITION 2.2 When qi is given ξi is given as follows. ξ =1−F F (1−q ) COROLLARY 2.2 In the framework given in Corollary 2.1 (1) ξi is given as follows. ξ =1−Φ μ +Φ (1−q ) , (6) i=1, 2, 3, where μn= n/2 δ (2) ξi is a monotone decreasing function of n, i=1, 2, 3. (3) The interquartile range is a monotone decreasing function of n. 3.Numerical evaluation of quartile and interquartile range Assuming the framework given in Corollary 2.1, we numerically evaluate in this section the Jpn J Biomet Vol. 40, No. 2, 2020 Reproducibility of statistical test results based on p-value 73 quartile and interquartile range of the distribution of p-value that are given in the previous section. Figure. 2. Median, lower and upper quartiles of the distribution of p-value when δ = 0.30. 3.1 Medians depend sharply on sample size Figure 2 gives curves of median, lower and upper quartiles of the distribution of p-value when δ =0.30 for n=20〜200, taking n in the horizontal line.
Details
-
File Typepdf
-
Upload Time-
-
Content LanguagesEnglish
-
Upload UserAnonymous/Not logged-in
-
File Pages11 Page
-
File Size-