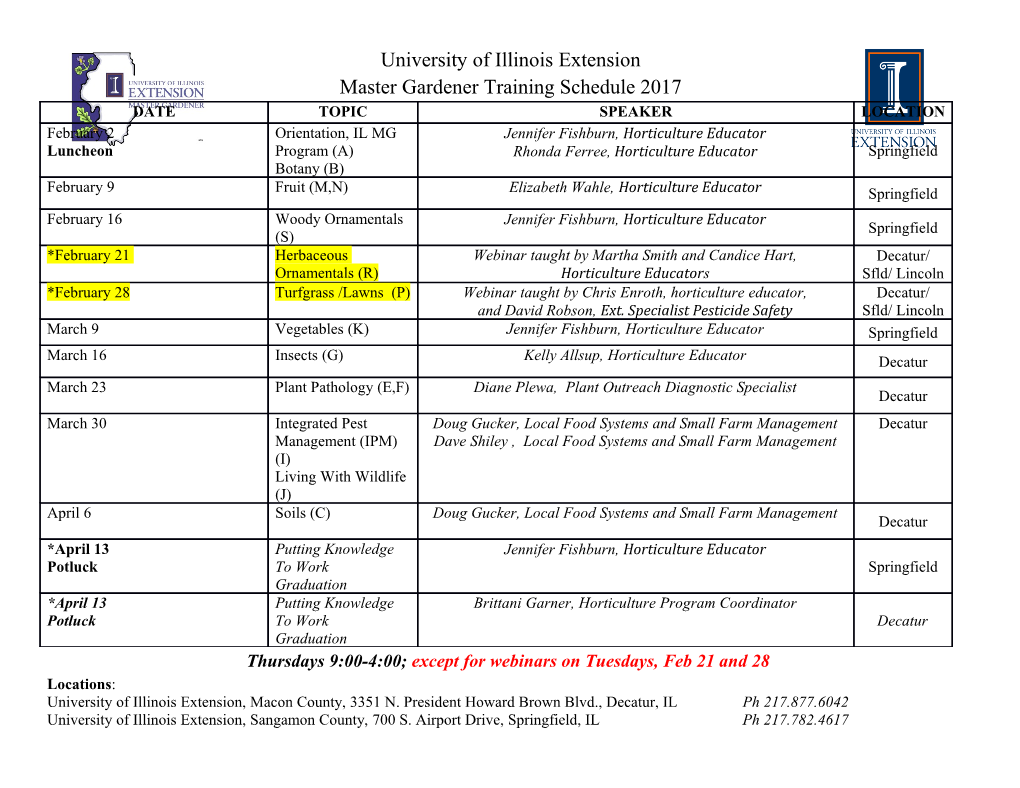
JOURNAL OF MEDICAL INTERNET RESEARCH Himelein-Wachowiak et al Viewpoint Bots and Misinformation Spread on Social Media: Implications for COVID-19 McKenzie Himelein-Wachowiak1, BA; Salvatore Giorgi1,2, MSc; Amanda Devoto1, PhD; Muhammad Rahman1, PhD; Lyle Ungar2, PhD; H Andrew Schwartz3, PhD; David H Epstein1, PhD; Lorenzo Leggio1, MD, PhD; Brenda Curtis1, MSPH, PhD 1Intramural Research Program, National Institute on Drug Abuse, Baltimore, MD, United States 2Department of Computer and Information Science, University of Pennsylvania, Philadelphia, PA, United States 3Department of Computer Science, Stony Brook Unversity, Stony Brook, NY, United States Corresponding Author: Brenda Curtis, MSPH, PhD Intramural Research Program National Institute on Drug Abuse 251 Bayview Blvd Suite 200 Baltimore, MD, 21224 United States Phone: 1 443 740 2126 Email: [email protected] Abstract As of March 2021, the SARS-CoV-2 virus has been responsible for over 115 million cases of COVID-19 worldwide, resulting in over 2.5 million deaths. As the virus spread exponentially, so did its media coverage, resulting in a proliferation of conflicting information on social media platformsÐa so-called ªinfodemic.º In this viewpoint, we survey past literature investigating the role of automated accounts, or ªbots,º in spreading such misinformation, drawing connections to the COVID-19 pandemic. We also review strategies used by bots to spread (mis)information and examine the potential origins of bots. We conclude by conducting and presenting a secondary analysis of data sets of known bots in which we find that up to 66% of bots are discussing COVID-19. The proliferation of COVID-19 (mis)information by bots, coupled with human susceptibility to believing and sharing misinformation, may well impact the course of the pandemic. (J Med Internet Res 2021;23(5):e26933) doi: 10.2196/26933 KEYWORDS COVID-19; coronavirus; social media; bots; infodemiology; infoveillance; social listening; infodemic; spambots; misinformation; disinformation; fake news; online communities; Twitter; public health articles focusing on COVID-19 has also grown exponentially; Introduction more research articles about the disease were published in the Globally, 2020 has been characterized by COVID-19, the first 4 months of the COVID-19 pandemic than throughout the disease caused by the SARS-CoV-2 virus. As of March 2021, entirety of the severe acute respiratory syndrome (SARS) and the COVID-19 pandemic has been responsible for over 115 Middle East respiratory syndrome (MERS) pandemics combined million documented cases, resulting in over 2.5 million deaths. [4]. Unfortunately, this breadth, and the speed with which The United States accounts for 24.9% of the world's COVID-19 information can travel, sets the stage for the rapid transmission cases, more than any other country [1]. of misinformation, conspiracy theories, and ªfake newsº about the pandemic [5]. One study found that 33% of people in the As the virus spread across the United States, media coverage United States report having seen ªa lotº or ªa great dealº of and information from online sources grew along with it [2]. false or misleading information about the virus on social media Among Americans, 72% report using an online news source [3]. Dr Tedros Adhanom Ghebreyesus, the Director-General of for COVID-19 information in the last week, with 47% reporting the World Health Organization, referred to this accelerated flow that the source was social media [3]. The number of research https://www.jmir.org/2021/5/e26933 J Med Internet Res 2021 | vol. 23 | iss. 5 | e26933 | p. 1 (page number not for citation purposes) XSL·FO RenderX JOURNAL OF MEDICAL INTERNET RESEARCH Himelein-Wachowiak et al of information about COVID-19, much of it inaccurate, as an conception that all bots spam or spread malware, but this is not ªinfodemicº [6]. the case. Some bots are benign, like the Twitter account @big_ben_clock, which impersonates the real Big Ben clock Though the pandemic is ongoing, evidence is emerging by tweeting the time every hour [21]. Others have even been regarding COVID-19 misinformation on social media. Rumors used for social good, such as Botivist, which is a Twitter bot have spread about the origin of the virus, potential treatments platform used for recruiting volunteers and donations [22]. or protections, and the severity and prevalence of the disease. Groups of bots can function in coordination with each other, In one sample of tweets related to COVID-19, 24.8% of tweets forming what are called botnets [23]. One botnet of roughly included misinformation and 17.4% included unverifiable 13,000 bot accounts was observed tweeting about Brexit, with information [7]. The authors found no difference in engagement most of these bot accounts disappearing from Twitter shortly patterns with misinformation and verified information, after the vote [24]. Bots of all types are ubiquitous on social suggesting that myths about the virus reach as many people on media and have been studied on Reddit [25,26], Facebook [27], Twitter as truths. A similar study demonstrated that fully false YouTube [28], and Twitter [29], among other platforms. claims about the virus propagated more rapidly and were more frequently liked than partially false claims. Tweets containing Given their large variety, bots are often organized into false claims also had less tentative language than valid claims subclasses, a selection of which we discuss here. Content [8]. polluters are one subclass; these are ªaccounts that disseminate malware and unsolicited content.º Traditional spambots, another This trend of misinformation emerging during times of subclass, are ªdesigned to be recognizable as botsº [30]. Social humanitarian crises and propagating via social media platforms botsÐa newer, more advanced type of bot [31-33]Ðuse ªa is not new. Previous research has documented the spread of computer algorithm that automatically produces content and misinformation, rumors, and conspiracies on social media in interacts with humans on social media, trying to emulate and the aftermath of the 2010 Haiti earthquake [9], the 2012 Sandy possibly alter their behavior.º There are also hybrid human-bot Hook Elementary School shooting [10], Hurricane Sandy in accounts (often called cyborgs) [34], which ªexhibit human-like 2012 [11], the 2013 Boston Marathon bombings [12,13], and behavior and messages through loosely structured, generic, the 2013 Ebola outbreak [14]. automated messages and from borrowed content copied from Misinformation can be spread directly by humans, as well as other sourcesº [35]. It is not always clear which category a bot by automated online accounts, colloquially called ªbots.º Social may fall into (eg, if a given social bot is also a cyborg). bots, which pose as real (human) users on platforms such as Various methods have been used to identify bots ªin the wild,º Twitter, use behaviors like excessive posting, early and frequent so as to build the data sets of known bots used to train retweeting of emerging news, and tagging or mentioning bot-detection algorithms. One method, the ªsocial honeypotº influential figures in the hope they will spread the content to [36], mimics methods traditionally used by researchers to their thousands of followers [15]. Bots have been found to monitor hacker activity [37] and email harvesting [38]. disproportionately contribute to Twitter conversations on Specifically, social honeypots are fake social media profiles set controversial political and public health matters, although there up with characteristics desirable to spammers, such as certain is less evidence they are biased toward one ªsideº of these issues demographics, relationship statuses, and profile pictures [39]. [16-18]. When bots attempt to spam the honeypots (by linking This paper combines a scoping review with an unpublished malware-infested content or pushing product websites), secondary analysis, similar in style to Leggio et al [19] and Zhu researchers can easily identify them. et al [20]. We begin with a high-level survey of the current bot literature: how bots are defined, what technical features Technical Features of Bots distinguish bots, and the detection of bots using machine Overview learning methods. We also examine how bots spread Features that distinguish bots from humans roughly fall into information, including misinformation, and explore the potential three categories: (1) network properties, such as hashtags and consequences with respect to the COVID-19 pandemic. Finally, friend/follower connections, (2) account activity and temporal we analyze and present the extent to which known bots are patterns, and (3) profile and tweet content. These feature publishing COVID-19±related content. categories have the advantage of being applicable across What Are Bots? different social media platforms [27]. Network Properties Before addressing issues surrounding the spread of Networks based on friend/follower connections, hashtag use, misinformation, we provide a definition of bots, describe their retweets, and mentions have been used in a number of studies typical features, and explain how detection algorithms identify that seek to identify social bots [40-43], exploiting network bots. homophily (ie, humans tend to follow other humans and bots Definition and Identification tend to follow other bots). As bots become more sophisticated, Bots, shorthand for ªsoftware robots,º come in a large variety network properties become less indicative of them; studies have of
Details
-
File Typepdf
-
Upload Time-
-
Content LanguagesEnglish
-
Upload UserAnonymous/Not logged-in
-
File Pages11 Page
-
File Size-