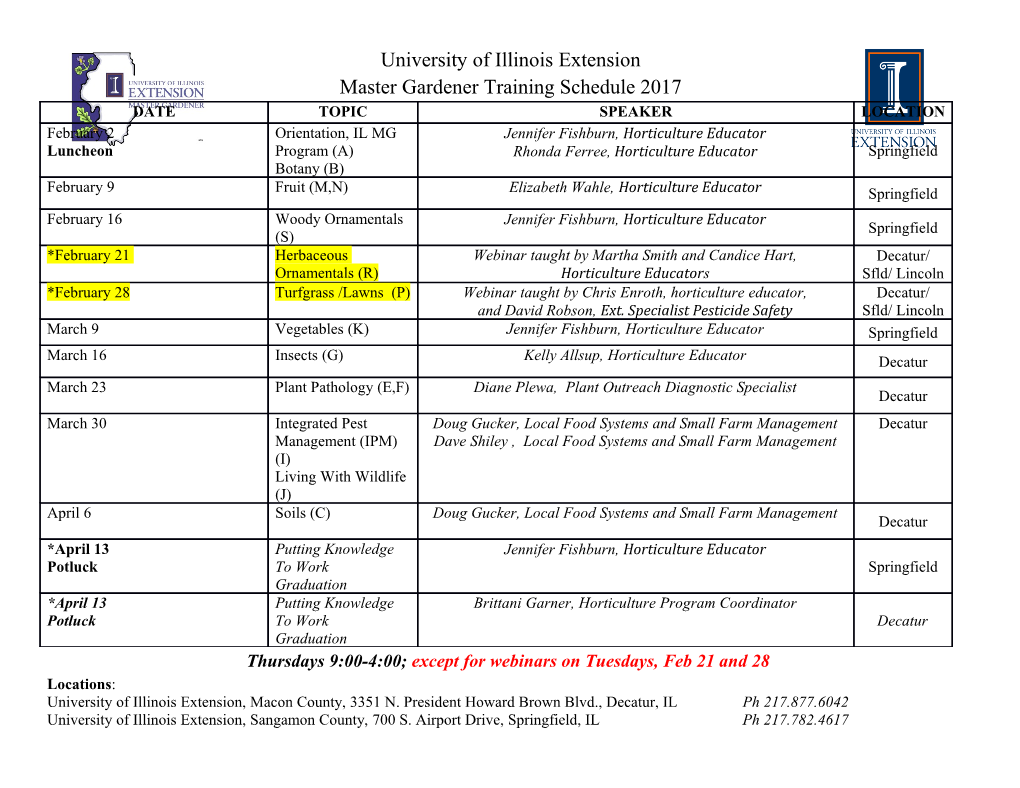
WHITE PAPER Mobile Malware Visual Analytics and Similarities of Attack Toolkits RiskSense® Technical White Paper Series Author: Anand Paturi, Manoj Cherukuri, John Donahue, and Dr. Srinivas Mukkamala Table of Contents Executive Summary 1 Introduction 2 Web Malware 3 Mobile Malware Analysis – Detecting Malcode In Repackaged Applications 3 Methodology 4 Attack Toolkits 5 Similarity Analysis of Attack Toolkits 6 Summary 9 WHITE PAPER • Mobile Malware Visual Analytics and Similarities of Attack Toolkits Executive Summary This paper discusses the use of Normalized Compression Distance (NCD), based on its capabilities to perform similarity measure of unstructured data, to enumerate code similarity between malicious Android applications and visualize their clusters. This document is part of the RiskSense Technical White Paper Series, which provides practical advice from a variety of RiskSense cybersecurity experts to assist security analysts in their day-to- day operations. The illustrated classification methods and visual analytics can help the anti-virus community to ensure that a variant of a known malware can still be detected without the need of creating a signature. This paper also showcases that the proposed methods can be used to understand the similarity / behavior with known malware families when a new malware is released. WHITE PAPER • Mobile Malware Visual Analytics and Similarities of Attack Toolkits WHITE PAPER • Mobile Malware Visual Analytics and Similarities of Attack Toolkits Page 1 Introduction Malicious applications installed on smart phones target them the same way as traditional malware would target personal computers since smart phones provide the same capabilities as handheld personal computers. A recent malware threat report from McAfee1 indicates that malicious applications increase by 100,000 samples a day and target smart phones, employing attack vectors that are the same as x86 and x64 malware. Most of the malicious mobile applications are the result Authors have implemented an application similarity measurement of repacking, which is a very common technique used by called DroidMOSS (using fuzzy hashing technique) to effectively adversaries whereby a legitimate application is modified by detect repackaged applications.4 Authors first perform feature injecting malicious code. Under this scenario, benign mobile extraction, which includes extracting of instructions in the applications are disassembled, embedded with malware, application and author information. They extract opcodes from re-assembled, and then re-packaged to look like benign the Dalvik bytecode for instructions and META-INF subdirectory applications. Finally, the embedded payload is launched when for author information of the application. Next fingerprint the application is activated. generation is performed, in which a sliding window hashing technique is used, whereby a sliding window starts from the very In prior reports, security researchers from the North Carolina beginning of the instruction sequence and moves forward until State University observed that much of Android malware is its rolling hashing value equals a pre-selected reset point, which repackaging other legitimate (popular) applications. After determines the boundary of the current piece. This hashing analyzing more than 1,200 Android malware samples, they technique is implemented on all instructions of the application, found that 86 percent repackaged legitimate applications to starting from the first instruction, a hash value is computed for include malicious payloads.2 In their recent experiments on (i + 1)th instruction to (j, (j +1)th) instruction to nth instruction. Android malware, they have observed that the detection rates of Additionally, a hash value is calculated for the entire instruction well-known anti-virus engines range from 51.02 percent to 100 sequence. The primary reason to calculate window hashes is to percent, while the detection rate of the Google App Verification find changes that could occur in a subset of instructions, which Service in Android 4.2 (JellyBean) is 20.41%.3 may be either adding or deleting instructions. Finally, authors perform similarity scoring to compute the distance between two fingerprints. This approach does not detect the malicious payload at source code level in the repackaged application and suffers from extracting features to perform the whole process. Authors propose a scalable infrastructure for code similarity analysis among Android applications.5 Initially, authors perform application pre-processing to represent it in a common XML- based format and then perform feature extraction using feature hashing. Finally, similarity measurement is performed to enumerate the similarity measure and extract the similar code. This methodology incurs the complexity of feature extraction, which might make it infeasible for quick analysis of repackaged applications over a large dataset. 1 https://threatpost.com/mobile-malware-way-mcafee-q2-threat-report-090412/76972/ 2 Y. Zhou, X, Jiang, “Dissecting Android Malware: Characterization and Evolution,” InProceedings of the 33rd IEEE Symposium on Security and Privacy (Oakland 2012), San Francisco, CA, May 2012 3 http://www.cs.ncsu.edu/faculty/jiang/appverify/ 4 W. Zhou, Y. Zhou, X. Jiang, and P. Ning. “Detecting Repackaged Smartphone Applicationsin Third-party Android Marketplaces,” In Proceedings of the Second ACM Conferenceon Data and Application Security and Privacy, CODASPY’12, pages 317-326, 2012 5 S. Hanna, L. Huang, E. Wu, S. Li, C. Chen, and D. Song. “Juxtapp: A Scalable System forDetecting Code Reuse Among Android Applications,” In Proceedings of the 9th Conferenceon Detection of Intrusions and Malware & Vulnerability Assessment, DIMVA’12 WHITE PAPER • Mobile Malware Visual Analytics and Similarities of Attack Toolkits Page 2 The approach that RiskSense cybersecurity experts propose, In our initial approaches we used Cosine measure to calculate the does not suffer from feature extraction, since they do not similarity between malware samples (Financial Crimeware and implement feature extraction to detect similarity in applications Attack Toolkits: Eleonore is similar to Fragus, IEKit, JustExploit, but rather use NCD for obtaining quick measurement of MyPloySploits, Neon, ExploitPack, and ZeroExploit). Cosine similarity analysis and then detect the degree of code similarity. Similarity is measured as the cosine of the angle between the In RiskSense’s current experiments for Mobile malware analysis two vectors (API sequence of malware samples). (focusing on DroidKungFu family), we calculate NCD between applications to measure their similarity and represent results in Our analysis shows that the shellcodes used as payloads, across a distance matrix. We apply a hierarchical clustering to group different attack kits, were nearly similar with scores over 90%. malware; the clusters are represented using a Pythagoras tree We observe that some of the attack kits released across different fractal. In our fractal visualization, all samples from the distance years had the same shellcodes. matrix are considered as a single square in the first step and from there on, two squares are constructed with each scaled down by a linear factor of 1⁄2 √2. With respect to mobile malware (DroidKungFu family) we can classify all the samples correctly and represent clusters (malware families and benign applications) using a Pythagoras tree fractal. Web Malware With perimeter defenses getting stronger over time, the Based on the results, similarity measures (Cosine Measure attackers have targeted the web for distributing malware. and Normalized Compression Distance) can be an effective Researchers have conducted several studies to understand the static mechanism to detect malware variants, shellcode based 6,7,8 life cycle of Web malware. Google had observed that about drive-by download attacks, attack toolkits, mobile malware, and 9 1.3 percent of the search queries result in a malicious link and repacked mobiles applications with malware. more than 60 percent of the Web attacks happen through the attack kits.10 Detection of shellcode for detecting the drive-by download attacks is one of the more focused approaches for preventing Web-based malware attacks. Previous researchers have published several approaches relying on heuristics and emulation for the detection of shellcodes.11, 12, 13 Mobile Malware Analysis: Detecting Malcode In Repackaged Applications In our methodology, mobile applications collected from multiple output of this method is a distance matrix based upon we application stores are passed through a pre-processing phase, visualize clusters of mobile applications, which shows the in which we convert each mobile application in to a pre-defined similarity between mobile applications based on their source format. Later we use gzip, bzip2, and rsynccompressors to code similarity. Using NCD enables us to perform similarity compress the pre-processed files (by defining a compression analysis without prior domain knowledge. block size) and then calculate the NCD between them. The 6 N. Provos, D. McNamee, P. Mavrommatis, K. Wang, and N. Modadugu. “The Ghost In The Browser Analysis of Web-based Malware,” In First Workshop on Hot Topics in Understanding Botnets, 2007 7 C. Kolbitsch, B. Livshits, B. Zorn, and C. Seifert. “Rozzle: De-cloaking internet malware,” Technical Report MSR-TR-2011- 94, Microsoft Research Technical Report, 2011 8 M. Polychronakis, and N. Provos. “Ghost turns zombie: Exploring the life
Details
-
File Typepdf
-
Upload Time-
-
Content LanguagesEnglish
-
Upload UserAnonymous/Not logged-in
-
File Pages12 Page
-
File Size-