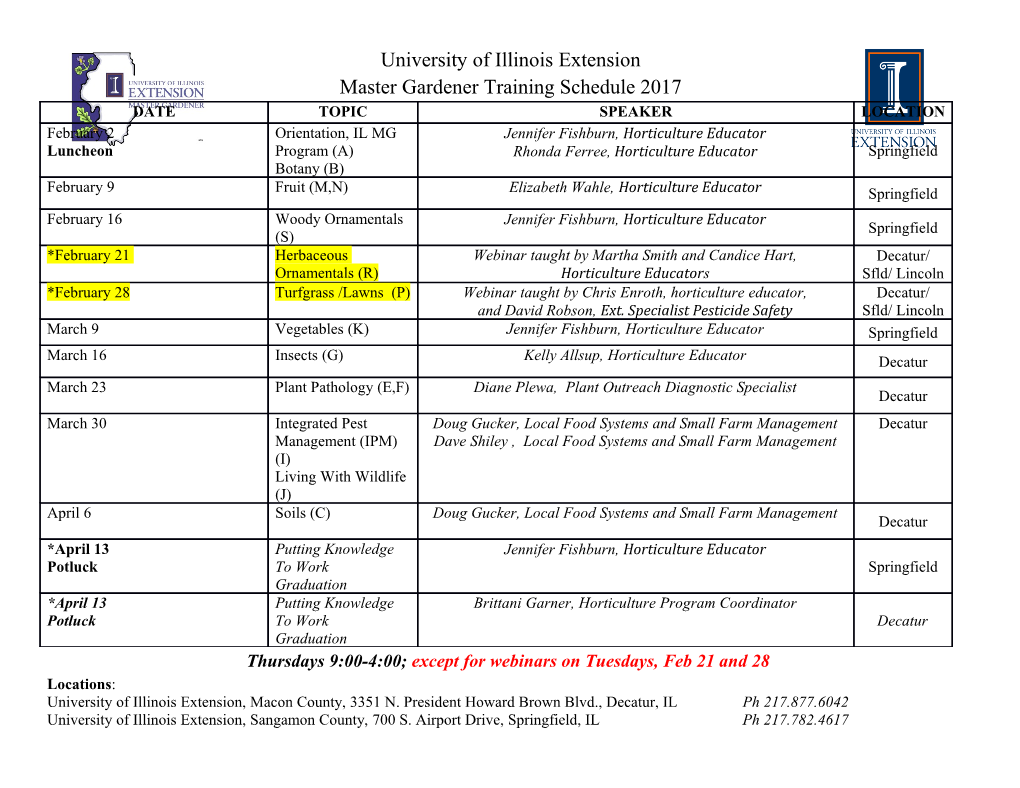
TREND ANALYSIS November 16, 2005 San Francisco, CA Jointly sponsored by California Department of Health Services, Maternal and Child Health Branch, Perinatal Advisory Council: Leadership, Advocacy, and Consultation (PAC/LAC), and Family Health Outcomes Project, UCSF TREND ANALYSIS Family Health Outcomes Project Staff Geraldine Oliva, MD, MPH Linda Remy, PhD Director Senior Statistician Brianna Gass, MPH Nadia Thind, MPH MCAH Coordinator Research Associate Suzanne Ezrre Mary Tran Web Administrator Training and Meeting Coordinator TODAY’S AGENDA Trend Analysis By the end of the training, participants should have introductory skills needed for trend analysis. At the end of the training, the participant will be able to: 1. Describe, analyze and interpret a trend 2. Interpret data from the new FHOP MCAH indicator spreadsheets 3. Identify trend results within and across jurisdictions 9:30 am Coffee and Registration 10:00 am Welcome and Introductions Geraldine Oliva, MD, MPH 10:15 am Session 1. Trend Analysis for Local Public Health Surveillance: Basic Concepts and Added Value Geraldine Oliva, MD, MPH 11:30 pm Session 2. Title V Indicators: The FHOP Databooks Linda Remy, PhD 12:00 pm Lunch 1:00 pm Title V Indicators: The FHOP Databooks (Continued) Linda Remy, PhD 2:00 pm Session 3. Working in Small Groups 3:30 pm Wrap-Up and Conclusions Trend Analysis for Local Public Health Surveillance: Basic Concepts and Added Value Geraldine Oliva M.D., MPH UCSF Family Health Outcomes Project November 16, 2005 Today’s Agenda Overview of current policies and thinking that generate an impetus for trend analysis Review basic analytical and statistical concepts used in trend analysis Review new indicator databook methods and utility Practice interpreting databook spreadsheets from counties and preparing an analysis Today’s Training Objectives By the end of this training, participants should be able to: – Describe why trend analysis is important – Articulate the definitions of terms used in trend analysis – Articulate the basic analytic methods that are used for a variety of situations – Evaluate longitudinal trends in county-level MCAH indicators – Interpret health disparity trends for different age and race/ethnic groups 1 Fiscal and Policy Changes Affecting MCAH National budget deficits are resulting in cuts for basic social and health services for poor families The state Title V Block Grant funds will be reduced as of July 2005 Some of the use of local funds as matching funds for public health nursing functions are being challenged New Expectations from Federal Funders The CDC has begun a major initiative on using evidence based prevention strategies This requires that state and local governments either use an evidence based model or have an evaluation that provides new evidence on the program’s cost and effectiveness Federal agencies are interested in program impact on fewer people rather than typical descriptive epidemiology for monitoring of health indicators in the entire population New Emphases at California DHHS Increasing focus on planning at the local level Increased focus on local solutions Explosion of programs using GIS to examine local health issues 2 Challenges for Local Health Jurisdictions Determine where to allocate reduced resources Monitor results of program cuts Advocate for local resources to sustain threatened programs Advocate at state level for general fund monies to sustain programs Analytic Implications A longitudinal perspective is required at the local level: – To assess the impact of changes in policy and funding among different populations – To assess the impact of new programs as they are implemented among different populations – To assess the impact as more or fewer clients are served over time – To assess the impact of an identified risk or outcome Statistical Implications To observe changes over time at the local level techniques such as time series analysis or forecasting may be used Time series is also used when examining the impact of a risk factor on an outcome e.g. Insurance status and access indicators Trend analysis can also be used to simultaneously control for the overall trends in two measures where the focus is the association of the two factors rather than the trend itself 3 Added Value of Trend Analysis The pattern of change in an indicator over time e.g. gradual change, spikes or curves, can provide information on the impact of exposure, new technology or policy changes Can provide comparison of trends among small geographic areas and regions Can provide comparisons of patterns among different population subgroups Can assist in making future projections Definition of Terms for Regression Analysis for Trends Slope – the average rate of change over time where: zero = flat (+) = increasing trend (-) = downward trend Intercept – the rate at the start of the study period i.e. where the line intercepts the x axis Slope: Fertility Rate per 1,000 Women 15 to 44 – 1991-2000 100 80 STATE: y = 82.8 - 1.5x COUNTY: y = 70.3 - 0.75x 60 40 20 0 1991 1992 1993 1994 1995 1996 1997 1998 1999 2000 4 Change in the Intercept: %LBW newborns (< 2,500 gms. 1991-2000 8 COUNTY: y = 7.5 - 0.13x 6 STATE: y = 5.9 + 0.04x 4 2 0 1991 1992 1993 1994 1995 1996 1997 1998 1999 2000 Definition of Terms for Regression Analysis for Trends P-value- the likelihood that model results are statistically significant: used both for significance of trend of an indicator and differences with comparison groups Standard Error - the Confidence Interval around the slope and intercept Factors Impacting Analysis Sample size and the number of time periods being examined Presence of outliers Availability of numerators and denominators over time Confounding-changes over time in factors related to the indicator of interest 5 Small Numbers and Data Points For large sample sizes at the state, federal and large county level simple linear plots describing changes are treated as error free For the usual county, city or district the problem of unstable rates due to small numbers requires tests of statistical significance and other statistical techniques In looking at the individuals impacted by a particular program or policy the same small numbers problems occur Sample size issues Minimum number for stable rate may require statistical techniques such as aggregating or averaging years Many years of data may be required in order to obtain at least 3 usable data points needed for a trend analysis One size does not fit all: approach differs between one jurisdiction and another and between on indicator and another Sample Size Issues: Raw Data 6 Sample Size Issues: Plot of Data Small #s Methods: Data Aggregation Small #s Methods: Data Averaging 7 Small #s Methods: Regression Techniques Presence of Outliers This can be the result of real events or a statistical problem – When looking at communicable disease there may be years with spikes due to a new flu strain – When small numbers are used to generate rates a spike may not reflect a real change – In an area with a relatively small population, missing or inaccurate data may cause spikes that are the result of problems at the data collection level Trend study 3 8 Availability of Numerators and Denominators For numerators this occurs where data come from surveys only done periodically e.g. CHIS Denominator issues: – Between census years we have to arrive at estimates by vendors using multiple data sets to make projections – Geographic boundaries may change e.g ZIP codes appear and disappear – Intercensile samples for race/ethnic subgroups are often too small to generate reliable rates Changes Over Time in Confounding Factors Policies affecting access to care can impact rates of service utilization e.g. first trimester prenatal care, teen pregnancy Socio-demographic changes in the population of a small area may be a main determinant of changes in an indicator Technological advances may drastically change outcomes e.g. better survival of pre- term infants Monitoring the Impact of Policy Changes Year 1983 1984 1985 1986 1987 1988 1989 1990 1991 1992 1993 1994 1995 1996 1997 1998 1999 2000 19 Healthy Family: 18 100% to 200% FPL 17 = MediCal SCHIP: Lt 19, 200% FPL 16 = CHDP or 50% gt MCaid eligibility 15 = AIM 14 = Healthy Family 13 12 MediCal: A 11 1984 1 year olds at FPL G 10 1985 up to age 5 FPL E 9 1986 up to age 3 with 100% FPL 8 1987 infant up to 185 FPL 7 1989 up to age 6, 133% FPL 6 AIM AI M 5 200% 200% to 300% FPL 4 to 3 CHDP 29 Months 250% 2 AIM FPL 1 100 to 200% FPL 0 CHDP 100% FPL Payor PPS and DRG Implemented MediCal MC legislation but not implemented MediCal MC Expansions Reforms Private Sector MC Reforms Begin Employers drop low wage coverage Employers mandate MC Employers drop insurance Legislative EMTALA EMS for undocumented Recession Residency Prop 187 Assets tests waived then dropped Reforms Medicaid for immigrants Requirements IRCA Welfare Reform 9 Likelihood of Hospital Admission by Race/Ethnicity 1.6 1.4 1.2 1.0 0.8 0.6 0.4 0.2 0.0 1983 1984 1985 1986 1987 1988 1989 1990 1991 1992 1993 1994 1995 1996 1997 1998 White Hispanic Black Asian ACS Rate/10,000 for Payor 50 40 30 20 10 0 1983 1984 1985 1986 1987 1988 1989 1990 1991 1992 1993 1994 1995 1996 1997 1998 Public Private Selecting an Appropriate Statistical Strategy Define the purpose and context of the analysis i.e. comparison groups or dates of specific events or policy changes Are there enough cases to do a trend analysis i.e. should I manipulate data to do a trend or should I use case studies
Details
-
File Typepdf
-
Upload Time-
-
Content LanguagesEnglish
-
Upload UserAnonymous/Not logged-in
-
File Pages68 Page
-
File Size-