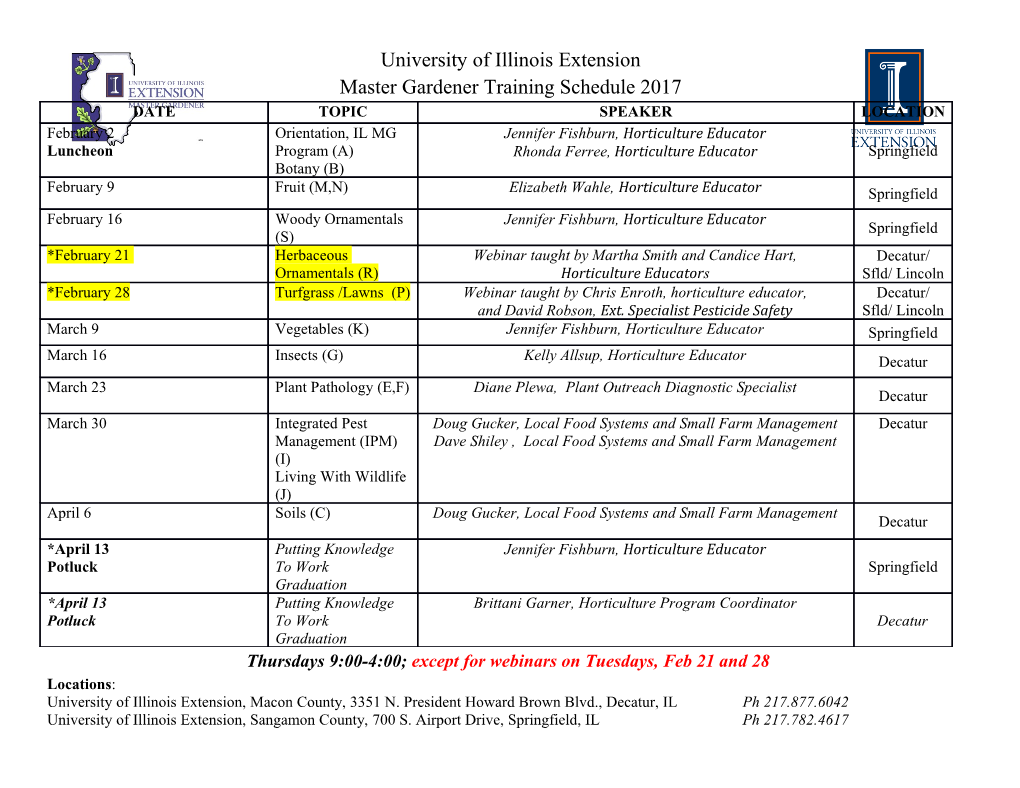
A novel Immune Optimization with Shuffled Frog Leaping Algorithm - A Parallel Approach (P-AISFLA) Suresh Chittineni, ANS Pradeep, Dinesh Godavarthi, Suresh Chandra Satapathy Anil Neerukonda Institute of Technology and Sciences Sangivalasa, Vishakapatnam, Andhra Pradesh, India E-mail: {sureshchittineni, pradeep.6174, dinesh.coolguy222, sureshsatapathy} @gmail.com Abstract: Shuffled frog leaping Algorithm (SFLA) is a new algorithm is used to calculate the global optimal of complex memetic, local search, population based, Parameter free, optimization problems and proven to be one of robust and meta-heuristic algorithm that has emerged as one of the fast efficient algorithms [2]. and robust algorithm with efficient global search capability. On the other side Clonal Selection Algorithm (CSA) is SFLA has the advantage of social behavior through the an Artificial Immune System Technique (AIS) that is inspired by process of shuffling and leaping that helps for the infection of the functioning of Clonal selection theory of acquired immunity. ideas. Clonal Selection Algorithm (CSA) are computational The algorithm provides two mechanisms for searching for the paradigms that belong to the computational intelligence desired final pool of memory antibodies. The first is a local search family and is inspired by the biological immune system of the provided via affinity maturation (hyper mutation) of cloned human body. Cloning and Mutation mechanisms in CSA help antibodies [3]. The second search mechanism provides a global in attaining the global optimal. CSA has the advantages of scope and involves the insertion of randomly generated antibodies Innate and Adaptive Immunity mechanisms to antigenic to be inserted into the population to further increase the diversity stimulus that helps the cells to grow its population by the and provide a means for potentially escaping from local optimal process of cloning whenever required. A hybrid algorithm is [4]. developed by utilizing the benefits of both social and immune Both these algorithms are powerful and efficient in mechanisms. This hybrid algorithm performs the parallel finding the global optimal solution. SFLA achieves this with the computation of social behavior based SFLA and Immune help of social behavior and local search exploitation. On the other behavior based CSA to improve the ability to reach the global hand, CLONALG achieves this by performing the process of optimal solution with a faster and a rapid convergence rate. cloning and Hyper mutation. Both algorithms ensure powerful This paper compared the Conventional CLONALG and search capability in different ways. So, in order to improve the SFLA approaches with the proposed hybrid algorithm and global search capability, to speed up the rate of convergence and tested on several standard benchmark functions. to achieve a stable solution, these two algorithms are combined to Experimental results show that the proposed hybrid approach form a hybri d evolutionary algorithm. A hybrid algorithm is significantly outperforms the existing CLONALG and SFLA developed by making use of the benefits of both social and approaches in terms of Mean optimal Solution, Success rate, immune mechanisms. This hybrid algorithm performs the Convergence Speed and Solution stability. computation of both SFLA and CLONALG in a parallel fashion. This Hybrid evolutionary algorithm preserves the features of both Index Terms: Shuffled Frog Leaping Algorithm (SFLA), SFLA and CLONALG and helps to ensure the global optimal in a CLONALG and P-AISFLA. faster way with a better converged mean solution. This hybrid algorithm is tested on various standard and well known bench I. INTRODUCTION mark optimization functions and found that, the proposed hybrid Swarm intelligence is a research hotspot in the artificial algorithm P-AISFLA outperforms both SFLA and CLONALG. intelligence field. It mainly simulates the population behaviors of The remainder of the paper is organized as follows: the complicate system in nature or society. Shuffled Frog Leaping section II describes the Shuffled Frog Leaping algorithm (SFLA) Algorithm (SFLA), inspired from the food hunting behavior of followed by a brief description of Clonal Selection Algorithm the frog and Clonal Selection Algorithm (CSA), inspired from the (CSA) in section III. The section IV briefs the important features immune system of the human body are such examples of this of both SFLA and CLONALG in terms of Social and immune field. behavior perspectives and also describes our proposed Hybrid SFLA is a population based memetic algorithm that algorithm. Section V gives further explanations, experimental carries the process of memetic evolution in the form of infection analysis, simulation results to several benchmark problems and of ideas from one individual to another in a local search. A comparisons of our proposed algorithms with the conventional shuffling strategy allows for the exchange of information between CLONALG and SFLA. Section VI concludes our paper with local searches to move toward a global optimum [1]. This some remarks and conclusions. II. SHUFFLED FROG LEAPING ALGORITHM (SFLA) Step 5.Local exploration: Repeat the process from step 3 for a specific number of iterations to improve the local search SFLA is a memetic algorithm inspired by the research of capability. food hunting behavior of frogs. It is based on evolution of memes carried by the interactive frogs and by the global exchange of Step 6.Shuffling: Shuffling is the process of combining all the information among themselves. SFLA is a combination of both frogs in all the memeplexes in to a single group. deterministic and random approaches [2]. The SFL algorithm progresses by transforming ‘‘frogs’’ in a Step 7.Convergence check: Return back to Step 2, if the memetic evolution. In this algorithm, frogs are seen as hosts for termination criterion is not met, else stop. memes and described as a memetic vector. Each meme consists of a number of memotypes. The memotypes represent an idea in a manner similar to a gene representing a trait in a chromosome in a III. IMMUNE OPTIMIZATION ALGORITHM genetic algorithm. The frogs can communicate with each other, (CLONALG) and can improve their memes by infecting (passing information) each other [5]. Improvement of memes results in changing an CLONALG is a population based Meta heuristic individual frog’s position by adjusting its leaping step size. algorithm and its main search power relies on cloning operator and mutation operator. The Clonal Selection Algorithm (CSA) A. Steps in SFLA Algorithm: reproduces only those individuals with high affinities and selects their improved and maturates progenies. This strategy suggests The following are the steps involved [6] in the Shuffled Frog that the algorithm performs a greedy search, where single Leaping Algorithm (SFLA): members will be locally optimized and the newcomers yield a Step 1.Random generation of frogs: Initially generate the broader exploration of the search-space [4]. This characteristic population of k frogs randomly within the feasible region. makes the CSA very suitable for solving optimization tasks. The basic steps and working of Immune Optimization algorithm Step 2.Evaluate fitness: The fitness values of each frog are (CLONALG) is described as follows: calculated and then sort all the k frogs in ascending order Step 1. Antibody Pool (AB) Initialization according to the fitness value. Identify the global best frog Initially, an Antibody Pool (AB) is created with N antibodies from the entire population. choosing randomly in the search space. Antibodies are represented by the variables of the problem (ab , ab ,...,ab ) Step 3.Partition to memeplexes: Partition all the k frogs in to p 1 2 N which are potential solutions to the problem. memeplexes, each containing q frogs such that k= p×q. The partition is done in such a way that, the first frog should send to Step 2. Antibody Selection: the first memplex, second one to the second memeplex, similarly, th th th the p frog to the p memeplex and the (p+1) frog back to the For each Antibody (abi), its corresponding affinity is determined. first memplex. These antibodies are then sorted according to the affinity calculated. Select the n highest affinity antibodies. Step 4.Memetic Evolution: In each memeplex, identify the best Step 3. Cloning: frog and the worst frog to perform the process of memetic evolution. In this process, the worst frog that is identified in Cloning is one of the key aspects in AIS. It is the process of each memeplex should be improved as follows: producing similar populations of genetically identical individuals. The selected best n Antibodies will be replicated in proportionate = (. ) × ( − ) (1) to their antigenic affinity. The replicated antibodies i.e., Clones = + (2) are maintained as a separate clone population C. The Number of (− ≤ ≤ ) Clones for each antibody can be calculated by the following Where, rand (.) is a random number between 1 and 0, and equation: B is the maximum allowed change in the frogs position. If this max β. N = ∑ round (3) process produces a better frog, then it should be replaced by the older frog. Otherwise, Xb is replaced by Xg in Equation (3) and the process is once again repeated. If non improvement becomes where Nc is the total number of clones generated, β is possible, in this case a random frog is generated which replaces a multiplying factor, N is the size of Antibody Pool (AB) and the old frog. round(.) is the operator that rounds its argument towards the closest integer. Clone size of each selected antibody is represented by each term of this sum. Higher the affinity is, higher becomes the number of clones generated for the selected If AB (i) is similar with AB (N) antibody [4]. Delete AB (N) in population Else Step 4. Affinity Maturation: j=j-1 End if The Clone Population C is now subjected to Mutation process, i=i+1 inversely proportional to its antigenic affinity measurement Until i= N-1 function. This Maturation helps for low affinity antibodies to Step3: If number of the antibodies< N mutate more in order to improve its affinity.
Details
-
File Typepdf
-
Upload Time-
-
Content LanguagesEnglish
-
Upload UserAnonymous/Not logged-in
-
File Pages8 Page
-
File Size-