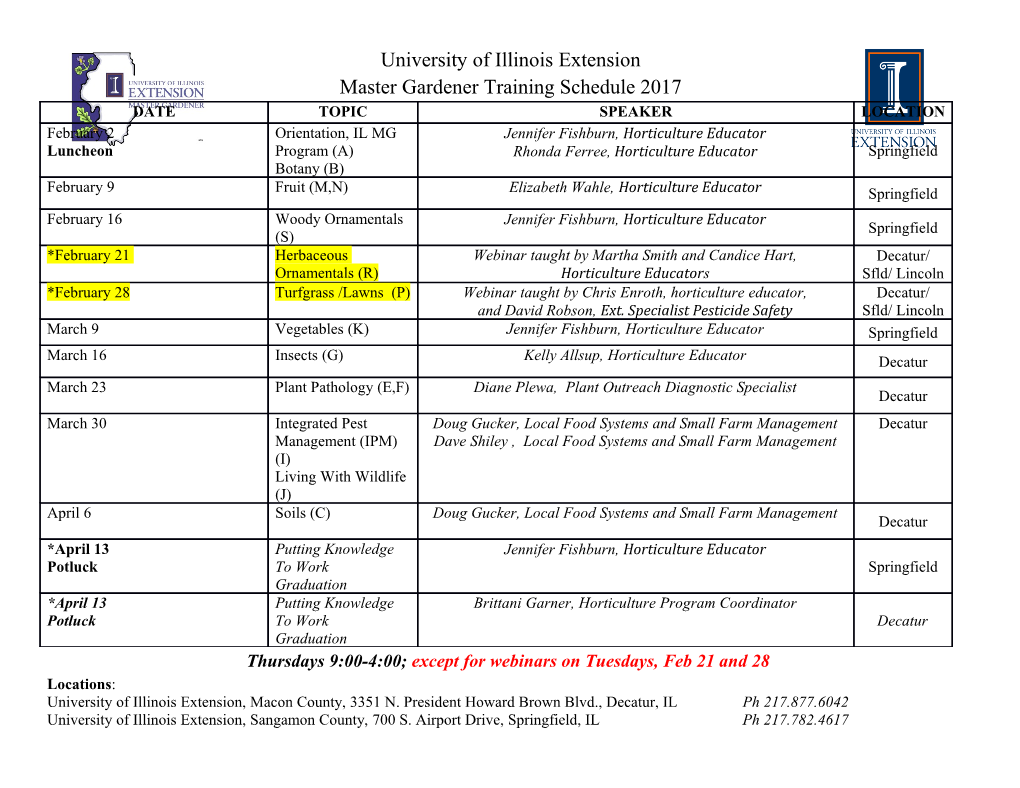
Open Journal of Statistics, 2013, 3, 312-318 http://dx.doi.org/10.4236/ojs.2013.35037 Published Online October 2013 (http://www.scirp.org/journal/ojs) Effect of Correlation Level on the Use of Auxiliary Variable in Double Sampling for Regression Estimation Dawud Adebayo Agunbiade*, Peter I. Ogunyinka Department of Mathematical Sciences, Olabisi Onabanjo University, Ago-Iwoye, Nigeria Email: *[email protected] Received June 21, 2013; revised July 21, 2013; accepted July 28, 2013 Copyright © 2013 Dawud Adebayo Agunbiade, Peter I. Ogunyinka. This is an open access article distributed under the Creative Commons Attribution License, which permits unrestricted use, distribution, and reproduction in any medium, provided the original work is properly cited. ABSTRACT While an auxiliary information in double sampling increases the precision of an estimate and solves the problem of bias caused by non-response in sample survey, the question is that, does the level of correlation between the auxiliary infor- mation x and the study variable y ease in the accomplishment of the objectives of using double sampling? In this re- search, investigation was conducted through empirical study to ascertain the importance of correlation level between the auxiliary variable and the study variable to maximally accomplish the importance of auxiliary variable(s) in double sampling. Based on the Statistics criteria employed, which are minimum variance, coefficient of variation and relative efficiency, it was established that the higher the correlation level between the study and auxiliary variable(s) is, the bet- ter the estimator is. Keywords: Correlation Level; Auxiliary Variable; Regression Estimator; Double Sampling and Relative Efficiency of Estimator 1. Introduction cases of ratio and regression estimations or the use of double sampling in ratio and regression estimations, there In sampling theory, auxiliary information may be utilized must exists positive correlation between the auxiliary va- at any of these three stages or by combining two or all of riable x and study variable y . This article, empirical- the three stages. These stages are: (1) at the pre-selection ly, investigates to ascertain the importance of correlation stage or designing stage of the survey in stratifying the level in the use of auxiliary variable in estimating the po- population; (2) at the sample selection stage; and (3) at pulation parameter using double sampling for regression the post-selection or estimation stage. In whatever case, estimation method. the use of auxiliary information in sample survey is bet- ter than the case where no auxiliary information is util- 2. Methodology ized. Ratio, regression, product and difference estimators take advantage of auxiliary information at the estimation 2.1. The Regression Estimator stage. However, when the population information is not Let y ,1,2,,xi n be the sample values of the known then double sampling method becomes necessary ii main character y and the auxiliary character x re- for estimation. [1] is of the opinion that estimation of re- spectively obtained with simple random sampling with- quired parameters can efficiently be done with ratio and out replacement (SRSWOR) of sample size n from the regression methods of estimation with two-phase sam- population size N . The linear regression estimator of pling or double sampling method. Double sampling for the mean as giving by [6] is: ratio estimation becomes necessary over double sampling ˆ for regression estimation if the data under consideration yyl Xx (1) are well fitted by a straight line through the origin [2]. where Among the authors who have recently contributed to the S use of auxiliary variable(s) to establish various estimators ˆ xy ; (2) S 2 for the population parameters are [3-5]. However, in both x *Corresponding author. ˆ estimated regression coefficient Copyright © 2013 SciRes. OJS D. A. AGUNBIADE, P. I. OGUNYINKA 313 X is the population mean; 2.3. Correlation Coefficient and Coefficient of x mean of the auxiliary information; an Determination y mean of the study variable The simplest method for measuring the relationship exis- The mean square error (MSE) of y is giving as: l tence between two variables (one dependent variable and 1 f 222 one independent variable) is with the tool of correlation Vylyx S S2 Sxy (3) n and regression analysis [8]. Correlation coefficient deter- mines the degree of relationship between variables. It is Similarly, the estimated mean square error (MSE) of linear when all parts x , y on a scattered diagram seem y is giving as: ii l to lie near a straight line or it is nonlinear when all parts 1 f seem to lie near a curve. This work focuses on linear cor- ˆ 222ˆ ˆ Vylyx s s2 sxy (4) n relation. Correlation between variables can be measured with the use of different indices (coefficients). The three expressing Equation (4) in terms of correlation coefficient; most popular of these indices are: Pearson’s Product-mo- s (where ˆ ˆ y ) ment correlation, Spearman’s rank coefficient and kan- sx dall’s tau coefficients. Kendall’s tau established by [9] can be used as an alternative to spearman’s rank correla- 1 f ˆ 22 ˆ Vyly s1 (5) tion coefficient for ranked data. [10] analysed the proper- n ties of kendall’s coefficient and states that “the coeffi- cient we have introduced provides a kind of average 2.2. Double Sampling for Regression Estimator measure of the agreement between pairs of numbers The When double sampling for regression estimation is (“agreement”, that is to say, in respect of order) and thus to be used, then there must exist non-zero interception of has evident recommendation as a measure of the concor- the regression line on the study variable axis of the scat- dance between two rankings” and “In general, is an tered diagram. The double sampling linear regression es- easier coefficient to calculation than . We shall see... timator of population mean is giving as that from most theoretical points of view is prefer- able to )”. It should be noted that Kendall uses to ˆ ydl yxx (6) represent Spearman’s rank correlation coefficient and where as Kendall Tau correlation coefficient. [11] declared that nowadays the calculation of Kendall’s coefficient ˆ estimated simple linear regression coefficient posses no problem. Kendall’s coefficient is equivalent to x sample mean at the first phase Spearman’s rank coefficient in terms of the underlying Reference [7], hence, presented the estimated variance assumptions, but they are not identical in magnitude, of ydl as since their underlying logic and computational formulae Vyˆ are quite different. Similarly, Kendall’s coefficient and dl spearman’s rank correlation coefficient imply different in- 11 11 (7) sss22 ˆˆ222 s terpretations. [12,13] examined the use of Pearson’s pro- yyx xy nN nn duct moment correlation coefficient and Spearman’s rank correlation coefficient for geographical data (on map data Equation (7) can be expressed in terms of ˆ (where that are spatially correlated). s Spearman’s rank correlation coefficient is a nonpara- ˆ y ˆ ), this gives metric (that is distribution free) rank statistic proposed as sx a measure of the strength of the association between two 11 11 variables as compared to Pearson’s product-moment co- ˆ 22ˆ 2 Vydl sy sy 1 (8) efficient, that is a parametric statistic. Similarly, [14] cla- nN nn rified that Spearman’s rank correlation is not a measure Similarly, [7] presented the optimum variance of dou- of the linear relationship between two variables as some ble sampling regression estimator as: statisticians declared. It accesses how well an arbitrary monotonic function can describe the relationship between S 2 2 Vyy C1 2 C2 (9) two variables, without making any assumptions about the dl opt C0 frequency distribution of the variables. Unlike Pearson’s product-moment coefficient, it does not require the as- s2 2 Vˆˆyccy 1 2 ˆ2 (10) sumption that the relationship between the variables is dl opt c0 linear nor does it require the variables to be measured on Copyright © 2013 SciRes. OJS 314 D. A. AGUNBIADE, P. I. OGUNYINKA interval scales. [14] confirmed that Pearson’s product- between variables. The square index is interpreted as pro- moment correlation coefficient (represented with r) was portion of variation in one variable accounted for by dif- the first formal correlation measure and it is still the most ferences in the other variable. According to [16], widely used measure of relationship. 2 The idea of this paper is to use correlation coefficient n xxyy to determine the level of relationship between the auxil- 2 i1 ii r 12 (13) iary and study variables, after which such data will be nn22 ii11xxii yy analysed with double sampling for regression type esti- mator to know which correlation level significantly con- Or tributes to the objective of implementing auxiliary vari- able. However, having considered all the correlation co- 2 2 sxy efficient measures, this paper will use Pearson’s product- r (14) s s moment correlation coefficient. xy where 10r 2 . 2.4. Pearson’s Product—Moment Correlation Coefficient and Its Coefficient of Error and Interpretation in Correlation Coefficient Determination Most common error associated with correlation and re- Pearson first developed the mathematical formula for this gression analysis, as emphasized by [16], is confusing important measure in 1985 when interpreting correlation coefficient result. The most common error in correlation coefficient interpretation is n xxyyii to conclude that changes in one variable causes changes r i1 (11) 12 in the other. Correlation coefficient indicates that char- nnxx22 yy ii11iiacteristics vary together or in opposite direction. How- ever, not interpreting the results of Correlation coeffi- [12] presented correlation in Equation (11) as the “func- cient is another common error. [16] claims that the coef- tion of raw scores and mean”.
Details
-
File Typepdf
-
Upload Time-
-
Content LanguagesEnglish
-
Upload UserAnonymous/Not logged-in
-
File Pages7 Page
-
File Size-