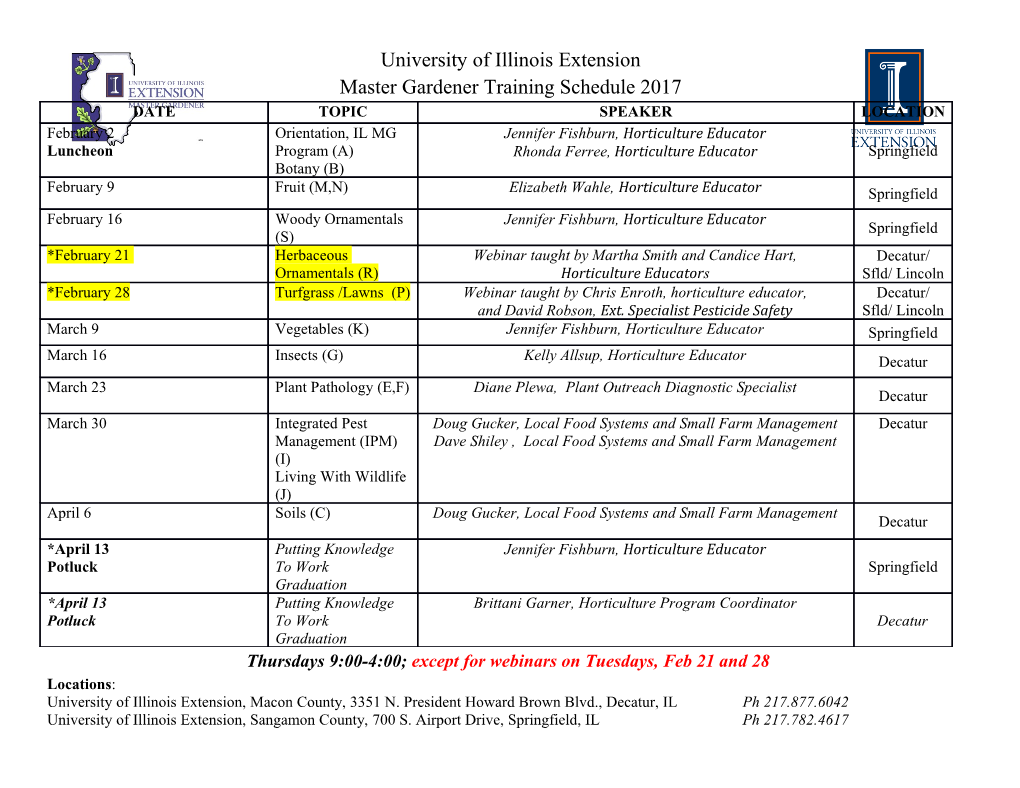
Image-based 3D Reconstruction: Neural Networks vs. Multiview Geometry Julius Schoning¨ and Gunther Heidemann Institute of Cognitive Science, Osnabruck¨ University, Osnabruck,¨ Germany Email: fjuschoening,[email protected] Abstract—Methods using multiple view geometry (MVG), like algorithms, guarantee linear processing time, even in cases Structure from Motion (SfM), are still the dominant approaches where the number and resolution of the input images make for image-based 3D reconstruction. These reconstruction methods MVG-based approaches infeasible. have become quite robust and accurate. However, how robust and accurate can artificial neural networks (ANNs) reconstruct For the research if the underlying mathematical principle a priori unknown 3D objects? Exceed the restriction of object of MVG can be learned by ANNs, datasets like ShapeNet categories this paper evaluates ANNs for reconstructing arbitrary [9] and ModelNet40 [10] cannot be used hence they en- 3D objects. With the use of a synthetic scalable cube dataset for code object categories such as planes, chairs, tables. The training, testing and validating ANNs, it is shown that ANNs are needed dataset must not have shape priors of object cat- capable of learning mathematical principles of 3D reconstruction. As inspiration for the design of the different ANNs architectures, egories, and also, it must be scalable in its complexity the global, hierarchical, and incremental key-point matching for providing a large body of samples with ground truth strategies of SfM approaches were taken into account. Based data, ensuring the learning of even deep ANN. For this on these benchmarks and a review of the used dataset, it is reason, we are using the synthetic scalable cube dataset [11], shown that voxel-based 3D reconstruction cannot be scaled. Thus, [12], which provides 3D objects and 2D images of different voxel-based reconstruction might be misleading for capturing the complexity of real-world images. Also, the benchmark results complexity. Within classical 3D reconstruction pipelines, a show that ANNs have the same limitation by reconstructing global, a hierarchical, or an incremental method for key-point unknown object categories as current MVG approach. matching is used depending on the amount and complexity Index Terms—3D Reconstruction; Artificial Neural Networks; of the data [13]. Assigning these three high-level concepts Image-Based 3D Reconstruction; Structure From Motion to the architecture of ANNs, the networks schematized in Fig. 1(b) – Fig. 1(d), are designed. For ensuring comparable I. INTRODUCTION benchmark results of the global, hierarchical, and incremental In theory, “standard multilayer feedforward networks are network, all of them have by design the same number of capable of approximating any measurable function to any trainable weights. desired degree of accuracy, in a very specific and satisfying sense” [1]. Hence, because the MVG methods consist of II. STATE OF THE ART a concatenation of several algorithms, it must be possible Using ANNs for the image-based 3D reconstructing is to approximate its underlying mathematical principle by an still in an early stage. For known object categories, a few ANN. The accuracy of commercial as well as academic 3D approaches [14], [15] were introduced recently. In contrast, reconstruction software like PhotoScan [2], VisualSfM [3], there are no ANN-based approaches for the 3D reconstruc- and ARC 3D [4] is for the most tasks sufficient [5]. Since tion of unknown or unseen objects and scenes. Therefore this software is based on MVG algorithm, the number, as we provide a brief overview of related approaches such as well as the size of input images, increase the processing time 3D object representation, single-view 3D reconstruction, and exponentially. stereo image generation by ANNs. In the last decade, the computing power of GPU has Using a multilayer feedforward network design, Pengs and improved remarkably due to the use of several thousand Shamsuddins study [16] explores the ability ANNs in learning parallel computing cores. Thus, GPU allows the massive 3D shapes through estimating the object z-coordinate. They parallelization of algorithms, so that the training as well as showed that a 3D object representation by an ANN is more the execution of ANN, even deep configurations with millions accurate than its representation by a 3rd order polynomial. of training weights, become feasible. Up to now, it has been Based on stacks of convolutional neural networks (CNNs), shown that ANN outperforms handcrafted algorithms in many image-based depth prediction is possible [17]. This multi-scale fields of applications such as object recognition tasks [6]– CNN produces a set of features for the entire image at the first [8]. In case ANNs can learn the underlying mathematical scale. The second scale predicts depth information in mid-level principle of MVG, then the trained ANNs can reconstruct resolution and the third scale yields depth information in high any unknown 3D object or scene without seeing it during resolutions. training. Such an image-based reconstruction ANN integrated Roy et al. [18] proposes a convolutional regression forest into 3D reconstruction software might, in contrast to the MVG where each node in the forest is associated with a CNN. The c 2018 IEEE. Personal use of this material is permitted. Permission from IEEE must be obtained for all other uses, in any current or future media, including reprinting/republishing this material for advertising or promotional purposes, creating new collective works, for resale or redistribution to servers or lists, or reuse of any copyrighted component of this work in other works. 3 CNN makes a depth estimation for a window around each r×r×r grid, 2r different 3D objects can be created and saved. pixel and returns a probability value for the depth accuracy. Exporting valid 3D obj files, duplicate vertices are combined In the manner for single view depth estimating, Garg et al. and inner faces, where two subcubes are connected with each [19], introduced an unsupervised framework to train a deep other, are deleted before saving the generated cube. ANN without labeled data. As input data, an image pair is needed showing the same scene but from a slightly different B. Image and Voxel Generator viewpoint. For the generation of the w input images with a pixel resolution x × x as well one voxel cloud with the resolution III. MVG BY ANNS - WHICH OUTPUT REPRESENTATION? of v × v × v voxel, a script is provided which accepts any ANNs have frequently been applied to object recognition 3D obj files as input data. For before running this script, the [6]–[8]. For classification tasks, the output layer usually has number of views w taken from the 3D object and there pixel one output neuron for each category. However, to describe the resolution x can be defined by the user as well as the voxel shape of 3D objects, e.g., in computer graphics and vision, resolution v. In case the randomized cubes are used as input vertices, edges, and faces are required, their numbers are for this generator the voxel resolution v should be set to r. equivalent to the object complexity. The first 3D computer To generate w images of the object from w different view- games use a simplified representation of 3D objects the points, the generator chooses viewpoints uniformly distributed volumetric pixels (voxels). Representing a 3D object or scene around the object to provide as different perspectives as at the output and bottleneck layer of an ANN, thus i) vertices, possible. The viewpoints are evenly distributed on a sphere ii) edges, iii) faces, or iv) voxels can be utilized. Since in using the Fibonacci lattice [20]. From these viewpoints, gray binary classification tasks, ANNs have a high performance, scale images are rendered. For each scene, a light source is the iv) voxel-based representation as ANN output is chosen. added next to the viewpoint. The images, as well as the voxel The voxel space, as an additional advantage, can easily be matrix of the objects, are created after scaling the object to scaled, which therefore affected the 3D reconstruction accu- the v × v × v grid. racy. Next, to the discussion of the output representation, the input representation for MVG by ANN should be, as for SfM C. Cube Datasets approaches, images captured from different viewpoints. In addition to the generators, Schoning¨ et al [11] have released three ready-to-use datasets—one 3×3×3, one 4×4×4 IV. SYNTHETIC SCALABLE CUBE DATASET and one 8 × 8 × 8. This ensures reproducibility to tackle their In the development history of digital cameras, the first baseline. These defined snapshots consist of 100000, 300000, running prototypes had had a low number of pixel, but these and 430000 different cube objects for the respective setup. All prototypes with its low resolution founded the basis for scaling objects care captured with a resolution of 100×100 pixels from up the camera resolution to several tens of megapixels. By 12 different viewpoints. the same argumentation line, we [11] design a synthetic scalable cube dataset for image-based 3D reconstruction of V. GLOBAL,HIERARCHICAL, AND INCREMENTAL ANNS voxel cubes. Obtaining the large numbers of examples required FOR 3D RECONSTRUCTION for training, testing, and validating ANNs with various amount The designed ANNs should be able to acquire 3D voxel of data, this dataset comes with a database generator that can matrices out the ready-to-use dataset, i.e. out of 12 different handle the Wavefront obj file format. Since the dataset should views. The output voxel matrix is defined on a binary voxel cover a variety of unrepeatable 3D obj objects random cube- grid where the values are 1=0 for voxel/non voxel, respectively. based voxel objects as basic geometric shapes are chosen. We design there different feedforward ANNs to emulate These cubes represent both simple and complex object, which the SfM strategies of global, hierarchical, and incremental are difficult to quantify in case real object categories are used.
Details
-
File Typepdf
-
Upload Time-
-
Content LanguagesEnglish
-
Upload UserAnonymous/Not logged-in
-
File Pages7 Page
-
File Size-