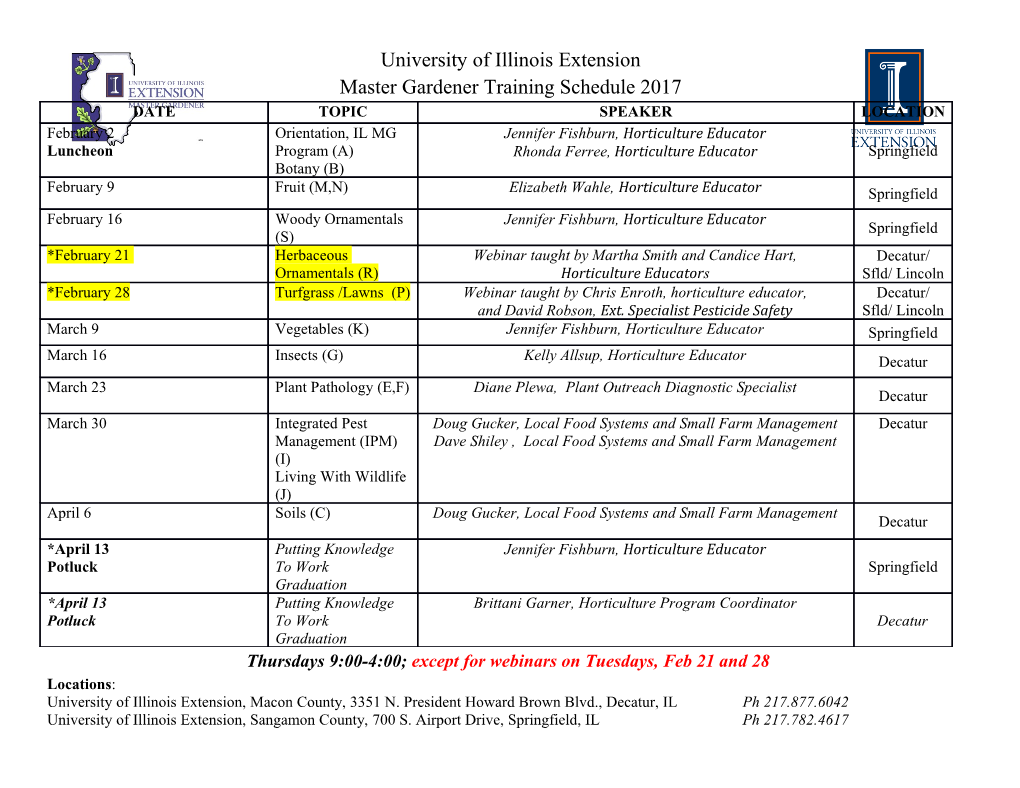
1 Strange Jet Tagging Yuichiro Nakai T. D. Lee Institute & Shanghai Jiao Tong U. Based on YN, D. Shih and S. Thomas, 2003.09517 [hep-ph]. Higgs and Flavor, EF02, Snowmass, August 2020 2 Jets at colliders Jet : collimated bunch of hadrons as the signatures of quarks and gluons produced in high-energy collisions ✓ QCD partons are never observed isolated due to confinement. ✓ They give cascades of radiation (parton shower) by QCD processes. ✓ Hadrons are formed at ∼ ΛQCD Understanding jets is a key ingredient of physics measurements and new physics searches at colliders. What initial parton produces a jet ? 3 Quark and Gluon Tagging Top quark Gluon S. Yang, talk slide More constituents with more uniform Jet mass, N-subjettiness, … energy fragmentation and wider. Bottom/Charm Up-type vs Down-type Look for a displaced pT -weighted jet charge (secondary) vertex. 1 Qi Q (p j )κ κ = jet κ ∑ j T (pT ) j∈jet Wikipedia The last missing piece : Strange quark tagging? 4 Tagging Strategy • Strange vs Gluon We can expect the same thing as quark/gluon discrimination. S. Yang, talk slide More constituents with more uniform • Strange vs Up energy fragmentation and wider. We can expect the same thing as up/down discrimination. pT -weighted jet charge 1 Qi = Q (p j )κ κ (p jet )κ ∑ j T • Strange vs Down T j∈jet Possible ?? Main theme of Both are quarks with the same charge. this talk 5 Tagging Strategy CMS experiment at the LHC Tracker : trajectories of charged particles ECAL : energy of electrons and photons HCAL : energy deposits of hadrons 6 Tagging Strategy After hadronization, strange quarks form Kaons : sd − ds sd + ds K − = su, K + = su, K ≈ , K ≈ No difference L 2 S 2 ± γ cτ ∼ 3 m Detector responses to hadrons : Down jets K L , K ± 0 ± No decay inside detectors K L KS K π π HN ◯ △ γ cτ 3 cm KS ∼ ECAL △ ◯ Decay inside detectors Tracker △ ◯ ◯ + − 0 0 KS → π π (∼ 70%), π π (∼ 30%) Strange jets Hadronic Neutral (HN) = HCAL - Tracker K-long (and K-short) can be used for tagging ! 7 Tagging Strategy K-short behaves very differently in detectors depending on decay length and decay mode. HCAL %±, !± γ cτ 3 cm X KS ∼ 150 cm Decay inside detectors ECAL + − 0 0 %' → 2* KS → π π (∼ 70%), π π (∼ 30%) X X 130 cm ! → 2%' → 4* X $ X X X X X Charged track pairs from Inner , - !$ → % % the secondary vertex. Tracker X Reconstructable X , - !$ → % % X !", !$ 8 Jet Samples Generate strange/down jet samples by PT distribution using MadGraph, PYTHIA and Delphes. 0.07 0.06 Z ss 1M events for each case of : 0.05 Z dd 0.04 0.03 Z → ss (pT > 20 GeV) 0.02 Z → dd (pT > 20 GeV) 0.01 0.00 20 40 60 80 PT [GeV] ss (pT > 200 GeV) PT distribution dd (pT > 200 GeV) 0.012 (η < 0.05) 0.010 ss 0.008 dd Initial parton is required to 0.006 be inside the leading jet : ΔR ≡ (Δη)2 + (Δφ)2 < 0.4 0.004 0.002 0.000 Herwig gives the similar results. 100 200 300 400 500 PT [GeV] 9 Ingredients of strange/down jets Strange jets contain more energetic Kaons than down jets. The pT fraction of a detector-stable particle averaged over jet samples : Z → ss (pT > 20 GeV) Z → dd (pT > 20 GeV) Mostly from Pi0 K± K± KL KS KS KL NSB NSB n n Leptons p CSB Leptons p CSB ± ± NSB: neutral strange baryons, CSB: charged strange baryons 10 Cut-Based Tagging Classify each jet into strange jet (signal) or down jet (background). Put a cut in distribution of HN — E. Measures to estimate efficiency (Correctly classified into signals) ε = and accuracy of taggers S (Total number of signal jets) (Misclassified into signals) ε = B (Total number of backgrounds) Larger εS Smaller εS Larger ε B Smaller ε B 1 1 1 1 0.5 0.5 0.5 0.5 0.1 strange 0.1 0.1 strange 0.1 0.05 down 0.05 0.05 down 0.05 0.01 0.01 0.01 0.01 0.005 pT = 45 GeV 0.005 0.005 pT > 200 GeV 0.005 -1.0 -0.5 0.0 0.5 1.0 -1.0 -0.5 0.0 0.5 1.0 HN -E HN -E 11 Neural Networks ✓ Powerful machine learning-based techniques used to solve many real-world problems Weights ✓ Modeled loosely after the human brain and Weights designed to recognize patterns ✓ Containing weights between neurons that are tuned by learning from data Convolutional Neural Network (CNN) ✓ Show high performance for image recognitions ✓ Maintain the spacial information of images arXiv:1712.01670 Apply a convolution operation to the input, passing the result to the next layer Reduce the image size 12 Jet Images and CNN Classification problem : Strange jet vs Down jet Create jet images with colors (Tracker, HN, ECAL) and feed them into CNN. Tracker HN ECAL Expectation Strange jet contains more energetic neutral Kaons. • HN image : brighter • ECAL image : darker Strangetop quark jet QCDDown quark jet Komiske, Metodiev, Schwartz (2016) 13 Average Images Strange jet (average) image is brighter in HN and darker in ECAL. Strange is darker Strange is brighter Z → ss (pT > 20 GeV) Z → dd (p > 20 GeV) No difference T 14 Average Images We add the 4th color of reconstructable KS pT. The intensity is normalized by the sum of the track pT , ECAL and HN in the whole image. 4th color ! Strange jets contain more energetic K-shorts Z → ss (pT > 20 GeV) than down jets. Z → dd (pT > 20 GeV) ★ The intensity is much small compared to the other colors because the number of images including reconstructable KS is less than 8% (5%) of the total number of images for strange (down) jets. 15 Results ROC curves : 1.0 1.0 pT = 45 GeV pT > 200 GeV 0.8 0.8 0.6 0.6 S S ciency 0.4 Truth BDT3 0.4 Truth BDT3 ffi E CNN4 CNN4 0.2 0.2 Cut1 Cut1 0.0 0.0 0.0 0.2 0.4 0.6 0.8 1.0 0.0 0.2 0.4 0.6 0.8 1.0 MistagD rate D The performance is worse than other classification problems. (quark vs. gluon, …) Can we use such a weak classifier ?? 16 Application CKM mixings The CKM matrix elements are fundamental parameters of the SM and their precise determination is important. However… The values for |Vcs| and |Vcd| are not measured very well. Because the charm quark mass is too heavy to be considered light but not heavy enough to treat in the heavy quark limit. If strange/down jet classification is possible… W boson decay W → cs, cd give the most direct measurement. Measure the ratio of strange to down jets : In principle, any amount of discrimination power will make the measurement possible with enough data. 17 Summary ✓ Strange tagging is the last missing piece of quark/gluon tagging. ✓ Neutral Kaons can be used for strange tagging. ✓ We create jet images with colors (Tracker, Hadronic Neutral, ECAL, KS pT). (= HCAL - Tracker) ✓ Average images of strange jets can be distinguished from down images. ✓ Convolutional Neural Network outperforms cut-based tagger. ✓ Strange jet tagger may be important for measuring CKM mixings. Thank you. Backup Material Applications of Strange Tagging • Top quark reconstruction ✓ All-hadronic channel � Full event reconstruction is possible. Jet combinatorics and large multi-jet � background are problematic. ✓ Semileptonic channel Leptonic top identifies event and � hadronic top can be reconstructed. � Jet combinatorics and multi-jet background are still issues. Which jets are W → cs, us, cd, ud decay products? T. McCarthy, talk slide Identification of strange jet may give some help. Applications of Strange Tagging • Light charged Higgs search Production : tt → W ±bH ∓b Decay : H + → cs The same issue as top quark reconstruction � is applied. � We do not know the charged Higgs mass! CMS • Squark search Identification of strange jet can … ✓ reduce the background Z(→ νν) + jets ✓ identify squark flavor after the discovery CMS Strange tagging may be important ! Ingredients of strange/down jets Strange jets contain more energetic Kaons than down jets. The pT fraction of a detector-stable particle averaged over jet samples : Strange !" > 200 GeV Down !" > 200 GeV K± KL K± KS KL KS NSB NSB n n Leptons p Leptons p ± CSB CSB ± More K-shorts due to boost factor NSB: neutral strange baryons, CSB: charged strange baryons 10 Truth-level classifier Classification problem : Strange jet vs Down jet 1 1 1 1 0.5 0.5 0.5 0.5 0.1 0.1 0.1 0.1 0.05 strange 0.05 0.05 strange 0.05 down down 0.01 0.01 0.01 0.01 0.005 pT = 45 GeV 0.005 0.005 pT > 200 GeV 0.005 -1.0 -0.5 0.0 0.5 1.0 -1.0 -0.5 0.0 0.5 1.0 0 0 (KL + KS + KS+ - - ) pT fraction (KL + KS + KS+ - - ) pT fraction Use Boosted Decision Tree (BDT) for classification. % Inputs have 3 dimensions: !" , !# , $ &' Approximately set the maximal performance we can achieve. Jet Images Create jet images with colors (Tracker, HN = HCAL - Tracker, ECAL). Image pre-processing 1. Shift an image so that the centroid is at the origin 2. Rotate the image so that the major principal axis is vertical 3. Flip the image so that the maximum intensity is in the upper right region 4. Normalize the image to unit total intensity : ˆ track ˆ ˆ ∑(pT + Ehad + Eem ) = 1 jet 5.
Details
-
File Typepdf
-
Upload Time-
-
Content LanguagesEnglish
-
Upload UserAnonymous/Not logged-in
-
File Pages56 Page
-
File Size-