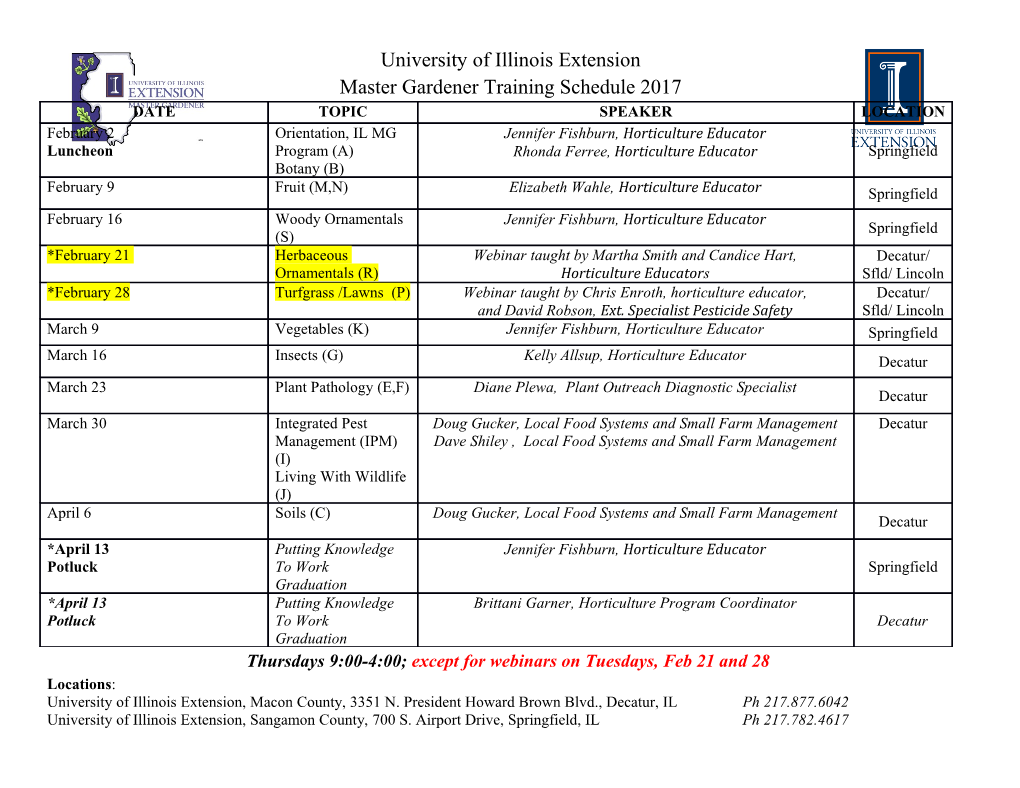
Large-Scale Empirical Analyses of the Abstract/Concrete Distinction Felix Hill ([email protected])1, Anna Korhonen ([email protected])1, Christian Bentz ([email protected])2 1 Computer Laboratory, University of Cambridge 2 Department of Theoretical and Applied Linguistics, University of Cambridge Abstract predicts association for concrete concepts to a greater extent than for abstract concepts. In Analysis 3, we show that free- We present original evidence that abstract and concrete concepts are organized and represented differently, based on association is a more symmetric relation for abstract statistical analyses of thousands of concepts in publicly concepts than for concrete concepts. The findings together available datasets. First, we show that abstract and concrete suggest contrasts in both the organization and representation concepts have differing patterns of association with other of abstract and concrete concepts. We conclude by concepts. Second, we test recent hypotheses that abstract discussing the implications of the findings for existing concepts are organized according to association, whereas theories and models of conceptual representation. concrete concepts are organized according to (semantic) similarity. Third, we present evidence suggesting that concrete representations are more strongly feature-based than Data abstract representations. We argue that degree of feature- Our analyses exploit three publicly available resources based structure may fundamentally determine concreteness, compiled to assist psychological modeling and analysis. and discuss implications for cognitive and computational models of meaning. USF Norms All three experimental analyses use the Keywords: Concreteness; concepts; similarity; association. University of South Florida (USF) Free-association Norms (Nelson & McEvoy, 2012). The USF data consists of over Introduction 5,000 words and their associates. In compiling the data, A large body of empirical evidence indicates important more than 6,000 participants were presented with cue words cognitive differences between abstract concepts, such as and asked to “write the first word that comes to mind that is guilt or obesity, and concrete concepts, such as chocolate or meaningfully related or strongly associated to the presented cheeseburger. It has been shown that concrete concepts are word”. For a cue word c and an associate a, the Forward more easily learned and remembered than abstract concepts, Association Probability (FAP) from c to a is the proportion and that language referring to concrete concepts is more of participants who produced a when presented with c. FAP easily processed (Schwanenflugel, 1991). Moreover, there is thus a measure of the strength of an associate relative to are cases of brain damage in which either abstract or other associates of that cue. concrete concepts appear to be specifically impaired Many of the cues and associates in the USF data have a (Warrington, 1975). In addition, functional magnetic concreteness score, derived from either the norms of Paivio, resonance imaging (fMRI) studies implicate overlapping but Yuille and Madigan (1968) or Toglia and Battig (1978). In partly distinct neural systems in the processing of the two both cases contributors were asked to rate words based on a concept types (Binder et al., 2005). Despite these widely scale of 1 (very abstract) to 7 (very concrete).2 known findings, however, there is little consensus on the cognitive basis of the observed differences WordNet WordNet is a tree-based lexical ontology (Schwanenflugel, 1991). Indeed, while many studies of containing over 155,000 words produced manually by conceptual representation and organization focus on researchers at Princeton University (Felbaum, 1998). The concrete domains, comparatively little has been established present work used WordNet version 3.0. empirically about abstract concepts.1 In this paper we test various theoretical claims concerning Brown Corpus Word frequencies were extracted from the the abstract/concrete distinction by exploiting large one million-word Brown Corpus (Kucera & Francis, 1967), publicly-available experimental datasets and computational chosen because it is an American corpus compiled at a resources. By analyzing thousands of abstract and concrete similar time to the USF data. Word tokens in the Brown concepts, our approach marginalizes potential confounds Corpus are tagged for their part of speech (POS). For a more robustly than in smaller-scale behavioral studies. In word type it is then possible to extract the majority POS (the Analysis 1 we show that abstract concepts are associated in POS with which the type is most frequently tagged). the mind to a wider range of other concepts, although the degree of this association is typically weaker than for concrete concepts. In Analysis 2 we explore the basis of 2Although concreteness is well understood intuitively, it lacks a these associations by testing the hypothesis that similarity universally accepted definition. It is often described in terms of reference to sensory experience (Paivio et al., 1968), but also 1 Notwithstanding a body of theoretical work (see e.g. Markman connected to specificity; rose is often considered more concrete and Stilwell, 2001). than flora. The present work does not address this ambiguity. 579 Analyses predictors even when controlling for frequency as an independent predictor. Each of our analyses is motivated by characteristics of the abstract/concrete distinction proposed in theoretical and behavioral studies. We have shown that abstract words have more associates than concrete words and lower variance in FAP Analysis 1: Patterns of Association distributions. This is consistent with the idea that the Motivation Schwanenflugel‟s Context Availability Model strength of their associates is on average weaker than for (1991) offers a theoretical basis for the aforementioned concrete words. Fig. 1 represents the strength of this effect empirical abstract/concrete differences. Her exposition of visually. Whilst this confirmation of H1 is consistent with the model relies on the following hypothesis:3 Schwanenflugel‟s Context Availability model, it is also consistent with other theoretical characterizations of the (H1) Abstract concepts have more (but weaker) abstract/concrete distinction (Paivio, 1986; Markman and connections (to other concepts) than concrete concepts. Stilwell, 2001). We thus investigate the distinction in more Schwanenflugel presents only small-scale behavioral detail in Analyses 2 and 3. experiments (64 words, 40 participants) in support of H1. All words Nouns only In Analysis 1 we test H1 on a far larger data set. Coeff. (β) t Coeff. (β) t Method We extracted those 3,255 pairs in the USF data for # Assocs -0.04*** -16.70 -0.04*** -15.97 which the concreteness of the cue-word was known. Since Variance -18.01*** -5.85 -15.64*** -4.41 cue words are connected to a finite set of associates by FAP log(Freq) -0.18*** -14.21 -0.12*** -7.87 values, we can isolate a probability distribution over 2 2 associates for each cue. Since our measure of association R = .17, R = .17, strength (FAP) is relative, it is not possible to compare these F(3, 3196) = 211.82*** F(3, 2319) =157.51*** strengths directly across cue words. Nonetheless, we can *p < 0.05; ** p < 0.01; *** p < 0.001 make inferences about absolute cue associate strength from properties of the FAP distributions. If a cue has many Table 1: Multiple regression analysis of Concreteness associates with little variance in the FAP distribution, each FAP value must necessarily be low (and absolute association strength intuitively weak). In contrast, for a 0.36 given number of associates, higher variance implies that some FAP values are notably higher than the mean, and 0.32 thus likely to be strong absolutely. Therefore, to address 0.28 H1 we considered both the dimension (number of associates) and the variance of the FAP distribution for 0.24 Concrete cues Abstract cues each cue word. 0.2 In an initial analysis of the data, we noted a moderate yrange 0.16 but significant negative correlation between concreteness FAP Average and frequency, r(3255) = -.16, p < .001. Therefore, a 0.12 multiple regression analysis was conducted with 0.08 log(Frequency), Number of Associates and Variance of FAP as predictors, and Concreteness as dependent 0.04 variable. Because the Concreteness/Frequency 0 multicolinearity was exacerbated by high frequency 0 10 20 30 40 50 60 70 80 90 1000 10 20 30 40 50 60 70 80 90 100 abstract prepositions and verbs, a second analysis was Associate Rank xrange conducted solely over cue words with majority POS „noun‟ (n = 2,320). Figure 1: Average FAP mass at each associate rank over the 500 most abstract and concrete cue words in the USF data. Results and Discussion In both cases the regression model Note the stronger initial associates in the concrete case and explained 17% of the variance of Concreteness and was the longer tail of weak associates in the abstract case. statistically significant. The beta coefficients in Table 1 indicate that concreteness correlates negatively with both Analysis 2: Distinct Conceptual Organization? #Associates and FAP Variance. Both are highly significant Motivation Based on recent behavioral studies of healthy and brain-damaged subjects, (see e.g. Crutch et al., 2009), Crutch and colleagues argue that abstract and concrete 3 E.g. she states “What is important to this view is not how concepts differ “qualitatively” in how they relate to other abstract
Details
-
File Typepdf
-
Upload Time-
-
Content LanguagesEnglish
-
Upload UserAnonymous/Not logged-in
-
File Pages6 Page
-
File Size-