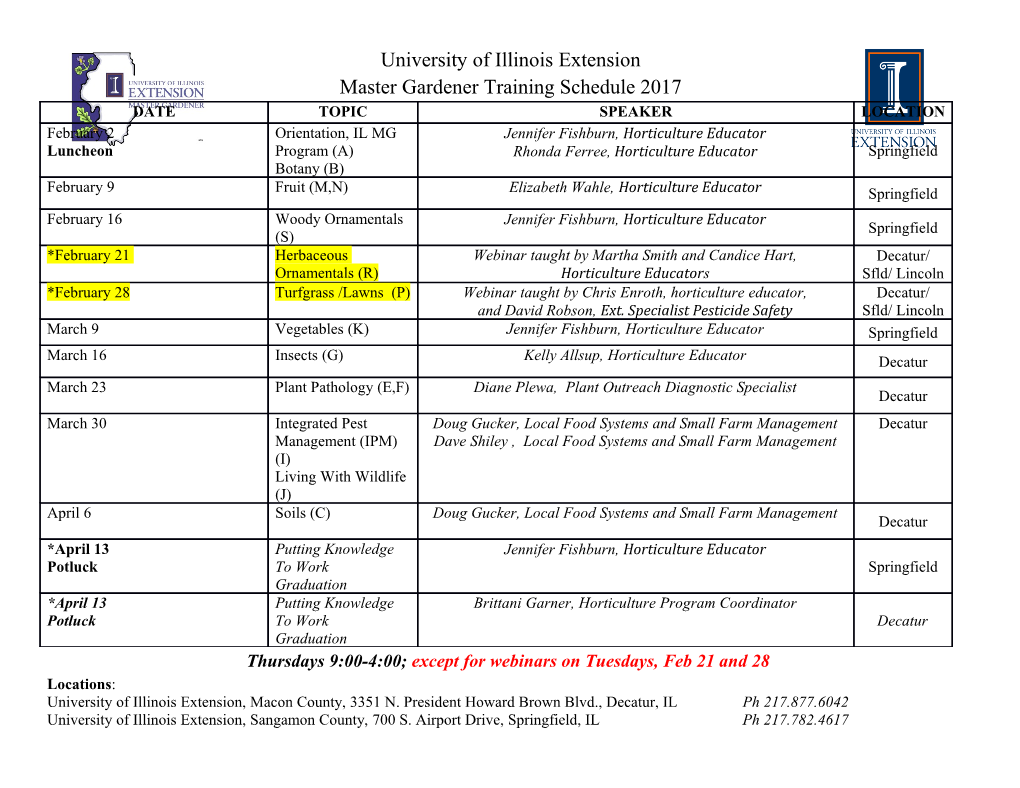
Suffix Trees Outline for Today ● Review from Last Time ● A quick refresher on tries. ● Suffix Tries ● A simple data structure for string searching. ● Suffix Trees ● A compact, powerful, and flexible data structure for string algorithms. ● Generalized Suffix Trees ● An even more flexible data structure. Review from Last Time A D B C B D A E A I O A R D T N T K U G D N A E D T T E I I A O K T Tries ● A trie is a tree that stores a collection of strings over some alphabet Σ. ● Each node corresponds to a prefix of some string in the set. ● Tries are sometimes called “prefix trees.” ● If |Σ| = O(1), all insertions, deletions, and lookups take time O(|w|), where w is the string in question. ● Can also determine whether a string w is a prefix of some string in the trie in time O(|w|) by walking the trie and returning whether we didn't fall off. Aho-Corasick String Matching ● The Aho-Corasick string matching algorithm is an algorithm for finding all occurrences of a set of strings P₁, …, Pₖ inside a string T. ● Runtime is O(m + n + z), where ● m = |T|, ● n = |P₁| + … + |Pₖ| ● z is the number of matches. Aho-Corasick String Matching ● The runtime of Aho-Corasick can be split apart into two pieces: ● O(n) preprocessing time to build the matcher, and ● O(m + z) time to find all matches. ● Useful in the case where the patterns are fixed, but the text might change. Genomics Databases ● Many string algorithms these days pertain to computational genomics. ● Typically, have a huge database with many very large strings. ● More common problem: given a fixed string T to search and changing patterns P₁, …, Pₖ, find all matches in T. ● Question: Can we instead preprocess T to make it easy to search for variable patterns? Suffix Tries Substrings, Prefixes, and Suffixes ● Recall: If x is a substring of w, then x is a suffix of a prefix of w. ● Write w = αxω; then x is a suffix of αx. ● Fact: If x is a substring of w, then x is a prefix of a suffix of w. ● Write w = αxω; then x is a prefix of xω ● This second fact is of use because tries support efficient prefix searching. Suffix Tries e s ● A suffix trie of T is a trie n o of all the suffices of T. n o s n e ● In time O(n), can determine whether P₁, …, s n e s n Pₖ exist in T by searching e s n e s for each one in the trie. e s n e n e s s e e nonsense A Typical Transform $ s n ● Typically, we e o append some new $ n o s n e character $ ∉ Σ to the end of T, then s n e s $ n construct the trie e s $ n e s for T$. $ e s n e ● Leaf nodes correspond to n e s $ suffixes. s $ e ● Internal nodes correspond to e $ prefixes of those suffixes. $ nonsense$ Constructing Suffix Tries ● Once we build a single suffix trie for string T, we can efficiently detect whether patterns match in time O(n). ● Question: How long does it take to construct a suffix trie? ● Problem: There's an Ω(m2) lower bound on the worst-case complexity of any algorithm for building suffix tries. A Degenerate Case $ a b $ a b b … b b … a $ ambm$ b b … b b b … b $ b … b $ ThereThere are are Θ( Θ(mm)) … b $ copiescopies of of nodes nodes chainedchained together together as as b $ m bbm$$.. $ 2 SpaceSpace usage: usage: Ω( Ω(mm2).). Correcting the Problem ● Because suffix tries may have Ω(m2) nodes, all suffix trie algorithms must run in time Ω(m2) in the worst-case. ● Can we reduce the number of nodes in the trie? Patricia Tries $ s n ● A “silly” node in a e o trie is a node that $ n o s n e has exactly one child. s n e s $ n ● A Patricia trie (or e s $ n e s radix trie) is a trie where all “silly” $ e s n e nodes are merged n e s $ with their parents. s $ e e $ $ nonsense$ Patricia Tries ● A “silly” node in a $ e s trie is a node that n o e has exactly one n $ n o s s $ child. e n s n e s ● A Patricia trie (or e s n s e radix trie) is a trie $ e $ n $ n e where all “silly” s $ s e nodes are merged e $ with their parents. $ nonsense$ 012345678 Suffix Trees ● A suffix tree for $ e s a string T is an n o e Patricia trie of T$ n 8 $ n o s s $ where each leaf is e n s n e s labeled with the 7 e s n s 6 e index where the $ e $ n $ n e corresponding s $ 4 s 5 e suffix starts in T$. e $ 3 $ 1 2 0 nonsense$ 012345678 Properties of Suffix Trees ● If |T| = m, the $ e s suffix tree has n o e exactly m + 1 n 8 $ n o s s $ leaf nodes. e n s n e s 7 e s n ● For any T ≠ ε, all s 6 e $ e $ n $ internal nodes in n e s $ the suffix tree 4 s 5 e e $ 3 have at least two $ 1 children. 2 ● Number of nodes 0 in a suffix tree is nonsense$ 012345678 Θ( m). Suffix Tree Representations ● Suffix trees may have Θ(m) nodes, but the labels on the edges can have size ω(1). ● This means that a naïve representation of a suffix tree may take ω(m) space. ● Useful fact: Each edge in a suffix tree is labeled with a consecutive range of characters from w. ● Trick: Represent each edge label α as a pair of integers [start, end] representing where in the string α appears. Suffix Tree Representations $ e s n o e n $ s 8 n o s $ n s n e e n s 7 e s 6 e $ e n o s $ e $ s n e $ start 8 4 0 1 3 n s s $ end 4 5 e 8 4 0 8 4 e $ 3 child $ 1 2 0 nonsense$ 012345678 Building Suffix Trees ● Using this representation, suffix trees can be constructed using space Θ(m). ● Claim: There are Θ(m)-time algorithms for building suffix trees. ● These algorithms are not trivial. We'll discuss one of them next time. An Application: String Matching String Matching ● Given a suffix tree, $ e s can search to see if n o e a pattern P exists in n 8 $ n o s s $ time O(n). e n s n e s ● Gives an O(m + n) 7 e s n s 6 e string-matching $ e $ n $ n e algorithm. s $ s e 4 e 5 3 ● T can be $ $ 1 preprocessed in time O(m) to 2 0 efficiently support binary string nonsense$ matching queries. 012345678 String Matching ● Claim: After $ e s spending O(m) time n o e preprocessing T$, n $ s can find all 8 n o s $ n s e e matches of a string n s 7 e s n P in time O(n + z), s 6 e $ e $ n $ where z is the n e s $ number of matches. 4 s 5 e e $ 3 $ 1 2 0 nonsense$ 012345678 String Matching ● Claim: After $ e s spending O(m) time n o e preprocessing T$, n $ s can find all 8 n o s $ n s e e matches of a string n s 7 e s n P in time O(n + z), s 6 e $ e $ n $ where z is the n e s $ number of matches. 4 s 5 e e $ 3 $ 1 Observation 1: Every Observation 1: Every 2 occurrenceoccurrence of of P P in in T T is is a a 0 prefixprefix of of some some suffix suffix of of T T.. nonsense$ 012345678 String Matching ● Claim: After $ e s spending O(m) time n o e preprocessing T$, n $ s can find all 8 n o s $ n s e e matches of a string n s 7 e s n P in time O(n + z), s 6 e $ e $ n $ where z is the n e s $ number of matches. 4 s 5 e e $ 3 $ 1 Observation 2: Because Observation 2: Because 2 thethe prefix prefix is is the the same same 0 eacheach time time (namely, (namely, P P),), all all thosethose suffixes suffixes will will be be in in nonsense$ thethe same same subtree. subtree. 012345678 String Matching ● Claim: After $ e s spending O(m) time n o e preprocessing T$, n $ s can find all 8 n o s $ n s e e matches of a string n s 7 e s n P in time O(n + z), s 6 e $ e $ n $ where z is the n e s $ number of matches. 4 s 5 e e $ 3 $ 1 2 0 nonsense$ 012345678 String Matching ● Claim: After $ e s spending O(m) time n o e preprocessing T$, n $ s can find all 8 n o s $ n s e e matches of a string n s 7 e s n P in time O(n + z), s 6 e $ e $ n $ where z is the n e s $ number of matches.
Details
-
File Typepdf
-
Upload Time-
-
Content LanguagesEnglish
-
Upload UserAnonymous/Not logged-in
-
File Pages76 Page
-
File Size-