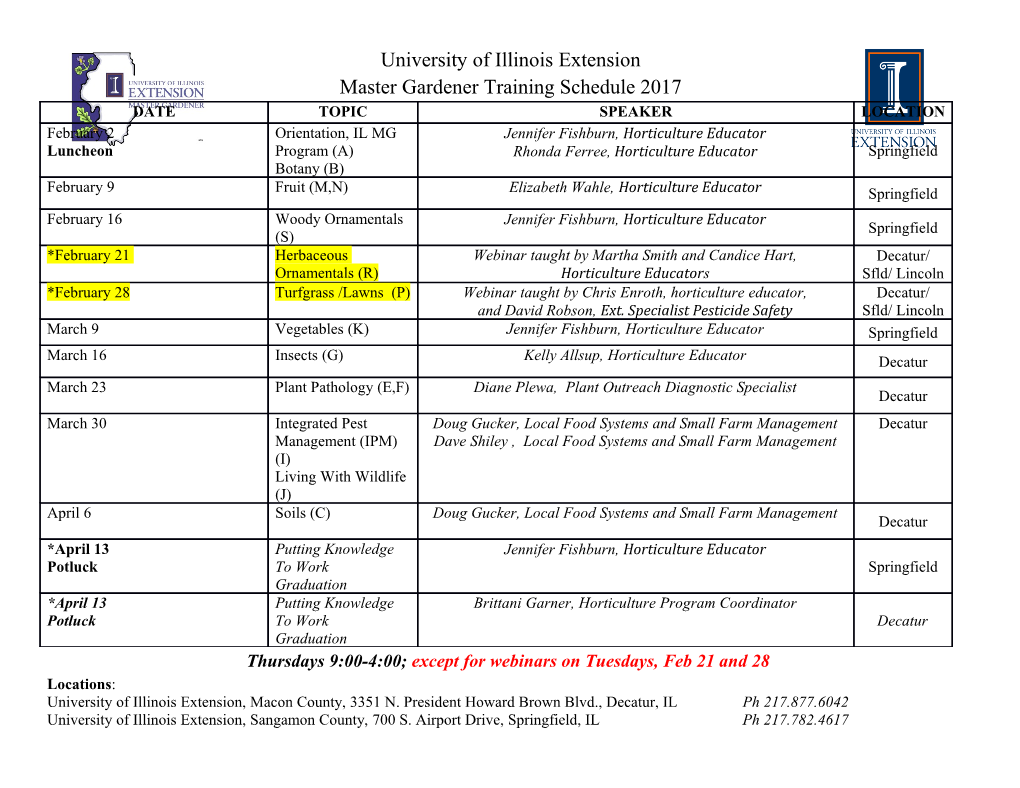
Some functional (H¨olderian)limit theorems and their applications (I) Alfredas Raˇckauskas Vilnius University Outils Statistiques et Probabilistes pour la Finance Universit´ede Rouen June 1{5, Rouen (Rouen 2015) Some functional limit theorems 1 / 94 2 To demonstrate possible applications of the weak invariance principle. This include detection of changed segment in a sample, e.g. epidemic change of a mean, of a variance and some other parameters. Aims of the lectures 1 To introduce the weak invariance principle in a H¨olderian framework (Lamperti's type IP) by considering large family of random structures including independent identically distributed random variables, linear processes, random fields and others. (Rouen 2015) Some functional limit theorems 2 / 94 Aims of the lectures 1 To introduce the weak invariance principle in a H¨olderian framework (Lamperti's type IP) by considering large family of random structures including independent identically distributed random variables, linear processes, random fields and others. 2 To demonstrate possible applications of the weak invariance principle. This include detection of changed segment in a sample, e.g. epidemic change of a mean, of a variance and some other parameters. (Rouen 2015) Some functional limit theorems 2 / 94 Aims of the lectures 1 To introduce the weak invariance principle in a H¨olderian framework (Lamperti's type IP) by considering large family of random structures including independent identically distributed random variables, linear processes, random fields and others. 2 To demonstrate possible applications of the weak invariance principle. This include detection of changed segment in a sample, e.g. epidemic change of a mean, of a variance and some other parameters. (Rouen 2015) Some functional limit theorems 2 / 94 Outline of the lecture Lamperti's invariance principle 1 Introduction 2 H¨olderspaces 3 Weak convergence and tightness 4 Lamperti's invariance principle 5 Adaptive FCLT 6 FCLT for triangular arrays (Rouen 2015) Some functional limit theorems 3 / 94 Motto of the lecture Theory without practice is empty Practice without theory is blind (adapted from Immanuel Kant) (Rouen 2015) Some functional limit theorems 4 / 94 Introduction First example Suppose we have a population (e.g., monthly earnings, pass-rates etc.) whose mean, µ, is unknown. In order to learn something about µ, one takes a large independent sample X1;:::; Xn from the population under consideration, and constructs the sample average 1 X := (X + ··· + X ): n n 1 n By the strong law of large numbers, X n ≈ µ. In order to find a more quantitative estimate one can use the central limit theorem (CLT) which is most rudimentary example from which one could start the story named ,,invariance principle". (Rouen 2015) Some functional limit theorems 5 / 94 CLT [classical] If σ2 2 (0; 1), then 1 D p (Sn − nµ) −−−! N (0; 1); nσ n!1 where −−−!D means convergence in distribution and N (m; σ2) denotes the n!1 normal random variable with mean m and variance σ2. −−−!D n!1 Let X1; X2;::: be independent identically distributed (i.i.d.) random 2 2 variables, EX1 = µ, EX1 = σ . Denote their partial sums by k X S0 = 0; Sk = Xj ; j ≥ 1: j=1 (Rouen 2015) Some functional limit theorems 6 / 94 −−−!D n!1 Let X1; X2;::: be independent identically distributed (i.i.d.) random 2 2 variables, EX1 = µ, EX1 = σ . Denote their partial sums by k X S0 = 0; Sk = Xj ; j ≥ 1: j=1 CLT [classical] If σ2 2 (0; 1), then 1 D p (Sn − nµ) −−−! N (0; 1); nσ n!1 where −−−!D means convergence in distribution and N (m; σ2) denotes the n!1 normal random variable with mean m and variance σ2. (Rouen 2015) Some functional limit theorems 6 / 94 Let X1; X2;::: be independent identically distributed (i.i.d.) random 2 2 variables, EX1 = µ, EX1 = σ . Denote their partial sums by k X S0 = 0; Sk = Xj ; j ≥ 1: j=1 CLT [classical] If σ2 2 (0; 1), then 1 D p (Sn − nµ) −−−! N (0; 1); nσ n!1 where −−−!D means convergence in distribution and N (m; σ2) denotes the n!1 normal random variable with mean m and variance σ2. −−−!D n!1 (Rouen 2015) Some functional limit theorems 6 / 94 By this CLT we have for a < b 2 R Z b p 1 −x2=2 lim P( n(X n − µ)/σ 2 (a; b)) = p e dx: n!1 2π a One can then use this to derive approximate confidence bounds for µ. Since P(N (0; 1) 2 [−1:96; 1:96]) ≈ 0:95, we deduce 1:96 1:96 P(µ 2 (X − p ; X + p ) ≈ 0:95 n σ n n σ n provided the sample size n is ,,large enough". (Rouen 2015) Some functional limit theorems 7 / 94 This was precisely asymptotic analysis of tn that initiated the so-called self-normalized CLT. If σ2 is unknown we can use its estimator n 1 X σ2 = (X − X )2 b n k n k=1 and explore the so called Student's t-statistics p n(X n − µ) tn = : σb (Rouen 2015) Some functional limit theorems 8 / 94 If σ2 is unknown we can use its estimator n 1 X σ2 = (X − X )2 b n k n k=1 and explore the so called Student's t-statistics p n(X n − µ) tn = : σb This was precisely asymptotic analysis of tn that initiated the so-called self-normalized CLT. (Rouen 2015) Some functional limit theorems 8 / 94 Recall that X1 belongs to the domain of attraction of a normal distribution (denoted by X1 2 DAN) if there exists a norming sequence bn " 1 such that −1 D b Sn −−−! N (0; 1): n n!1 Theorem (Selfnormalized CLT [Gin´e,G¨otze,Mason (1997)]) Let X1;:::; Xn be iid random variables. Then the convergence Sn D q −−−! N (0; 1) 2 2 n!1 X1 + ··· + Xn holds if and only if X1 2 DNA and EX1 = 0: (Rouen 2015) Some functional limit theorems 9 / 94 More precisely we have a sample X1; X2;:::; Xn and we wish to know whether there is a k∗, 1 < k∗ < n; such that EX1 = ··· = EXk∗ = µ, 0 EXk∗+1 = ··· = Xn = µ Figure: Annual temperature and µ 6= µ0: 1 Second example Now suppose you are drawing samples as time passes, and wish to know if the mean of the underlying population (e.g., annual temperature) has changed over time. (Rouen 2015) Some functional limit theorems 10 / 94 Second example Now suppose you are drawing samples as time passes, and wish to know if the mean of the underlying population (e.g., annual temperature) has changed over time. More precisely we have a sample X1; X2;:::; Xn and we wish to know whether there is a k∗, 1 < k∗ < n; such that EX1 = ··· = EXk∗ = µ, 0 EXk∗+1 = ··· = Xn = µ Figure: Annual temperature and µ 6= µ0: 1 (Rouen 2015) Some functional limit theorems 10 / 94 The simplest way to construct a required test is to take two parts of the given sample, one X1;:::; Xk and the second one Xk+1;:::; Xn, and to compare their sample averages 1 1 (X + ··· + X ) and (X + ··· + X ): k 1 k n − k k+1 n Calculating their difference gives the quantity k n h X i T (k) := X − kX n k(n − k) j n j=1 which should be large provided the equality of means before and after the moment k∗ = k fails. ∗ As the point of change k is not known one has to look at Tn(k) for all possible k = 1;:::; n. (Rouen 2015) Some functional limit theorems 11 / 94 Thus, it turns out that one needs to investigate the quantity Tn := max (Sk − kX n): 1≤k≤n This is CUSUM test statistics which is one of the most frequently used method to detect change points. Actually it might be easier to start with Mn := max (Sj − jµ); n ≥ 1 1≤j≤n and ask the question whether there is a limit distribution for Mn as n ! 1 under the assumption that X1;:::; Xn all have the same distribution? It turns out that the answer is positive, and involves an invariance principle. (Rouen 2015) Some functional limit theorems 12 / 94 Erd¨osand Kac described their method of proof as follows: ,,The proof of all these theorems follow the same pattern. It is first proved that the limiting distribution exists and is independent of the distribution of the Xi 's; then the distribution of Xi 's is chosen conveniently so that the limiting distribution can be calculated explicitly." Perhaps the story ,,invariance principle" have realy started with their providence. Erd¨os1 and Kac2contribution The limit behavior of Mn = max1≤j≤n(Sj − jµ) was precisely the question that were interested Erd¨osand Kac (1946) in. They found r Z x p 2 −y 2=2 lim P( max (Sj − jµ) ≤ nx) = e dy n!1 1≤j≤n π 0 for all x ≥ 0, provided X1; X2;::: are iid with mean µ and var(X1) = 1. 1Paul Erd¨os(26 March 1913 { 20 September 1996) was a Jewish-Hungarian mathematician 2Mark Kac (3 August 1914 { 26 October 1984) was a Polish American mathematician (Rouen 2015) Some functional limit theorems 13 / 94 Perhaps the story ,,invariance principle" have realy started with their providence. Erd¨os1 and Kac2contribution The limit behavior of Mn = max1≤j≤n(Sj − jµ) was precisely the question that were interested Erd¨osand Kac (1946) in. They found r Z x p 2 −y 2=2 lim P( max (Sj − jµ) ≤ nx) = e dy n!1 1≤j≤n π 0 for all x ≥ 0, provided X1; X2;::: are iid with mean µ and var(X1) = 1.
Details
-
File Typepdf
-
Upload Time-
-
Content LanguagesEnglish
-
Upload UserAnonymous/Not logged-in
-
File Pages144 Page
-
File Size-