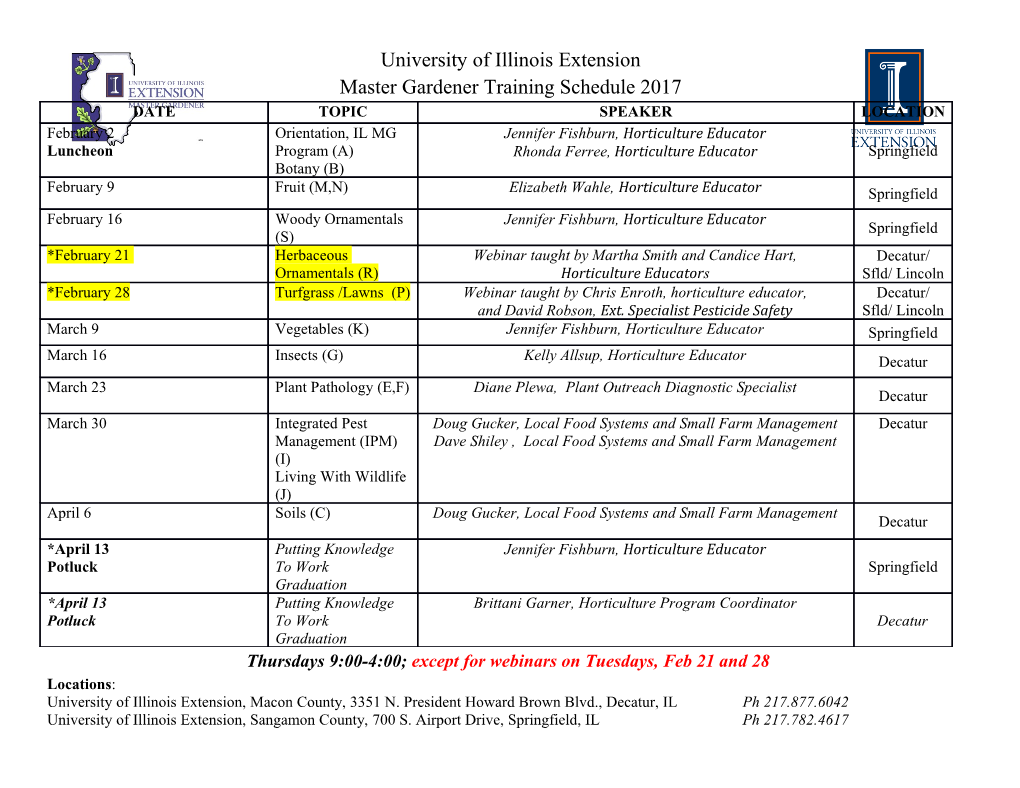
On the Reproducibility of Meta-Analyses 1 1 RUNNING HEAD: ON THE REPRODUCIBILITY OF META-ANALYSES 2 3 4 On the Reproducibility of Meta-Analyses: Six Practical Recommendations 5 6 Daniël Lakens 7 Eindhoven University of Technology 8 Joe Hilgard 9 University of Missouri - Columbia 10 Janneke Staaks 11 University of Amsterdam 12 13 In Press, BMC Psychology 14 15 Word Count: 6371 16 17 Keywords: Meta-Analysis; Reproducibility; Open Science; Reporting Guidelines 18 19 Author Note: Correspondence can be addressed to Daniël Lakens, Human Technology 20 Interaction Group, IPO 1.33, PO Box 513, 5600MB Eindhoven, The Netherlands. E-mail: 21 [email protected]. We would like to thank Bianca Kramer for feedback on a previous draft 22 of this manuscript. 23 24 Competing Interests: The authors declared that they had no competing interest with respect 25 to their authorship or the publication of this article. 26 27 Author Contributions: All authors contributed writing this manuscript. DL drafted the 28 manuscript, DL, JH, and JS revised the manuscript. All authors read and approved the final 29 manuscript. 30 31 Email Addresses: D. Lakens: [email protected] 32 Joe Hilgard: [email protected] 33 Janneke Staaks: [email protected] On the Reproducibility of Meta-Analyses 2 34 Abstract 35 Background 36 Meta-analyses play an important role in cumulative science by combining information 37 across multiple studies and attempting to provide effect size estimates corrected for 38 publication bias. Research on the reproducibility of meta-analyses reveals that errors are 39 common, and the percentage of effect size calculations that cannot be reproduced is much 40 higher than is desirable. Furthermore, the flexibility in inclusion criteria when performing a 41 meta-analysis, combined with the many conflicting conclusions drawn by meta-analyses of 42 the same set of studies performed by different researchers, has led some people to doubt 43 whether meta-analyses can provide objective conclusions. 44 Discussion 45 The present article highlights the need to improve the reproducibility of meta-analyses 46 to facilitate the identification of errors, allow researchers to examine the impact of subjective 47 choices such as inclusion criteria, and update the meta-analysis after several years. 48 Reproducibility can be improved by applying standardized reporting guidelines and sharing 49 all meta-analytic data underlying the meta-analysis, including quotes from articles to specify 50 how effect sizes were calculated. Pre-registration of the research protocol (which can be peer- 51 reviewed using novel ‘registered report’ formats) can be used to distinguish a-priori analysis 52 plans from data-driven choices, and reduce the amount of criticism after the results are 53 known. 54 Summary 55 The recommendations put forward in this article aim to improve the reproducibility of 56 meta-analyses. In addition, they have the benefit of “future-proofing” meta-analyses by 57 allowing the shared data to be re-analyzed as new theoretical viewpoints emerge or as novel 58 statistical techniques are developed. Adoption of these practices will lead to increased 59 credibility of meta-analytic conclusions, and facilitate cumulative scientific knowledge. On the Reproducibility of Meta-Analyses 3 60 On the Reproducibility of Meta-Analyses: Six Practical Recommendations 61 Any single study is just a data-point in a future meta-analysis. Meta-analyses play an 62 important role in cumulative science by combining information across multiple studies. 63 Especially when sample sizes are small, effect size estimates can vary substantially from one 64 study to the next. Statistical inferences are even more complicated due to publication bias. 65 Because statistically significant studies are more likely to be published, it is a challenge to 66 draw quantifiable conclusions about the presence or absence of effects based on the published 67 literature. Meta-analytic statistical tools can provide a partial solution to these problems by 68 drawing conclusions over multiple independent studies and calculating effect size estimates 69 that attempt to take publication bias into account. Although meta-analyses do not provide 70 definitive conclusions, they are typically interpreted as state-of-the-art empirical knowledge 71 about a specific effect or research area. Large-scale meta-analyses often accumulate a 72 massive number of citations and influence future research and theory development. It is 73 therefore essential that published meta-analyses are of the highest possible quality. 74 To guarantee this quality, both pre- and post-publication peer review are important 75 tools. Peers can check the quality of meta-analyses only when the meta-analytic effect size 76 calculations are reproducible. Unfortunately, this is rarely the case. Gøtzsche, Hróbjartsson, 77 Marić, and Tendal (2007) examined whether standardized mean differences in meta-analyses 78 were correctly calculated and reproducible for 27 meta-analyses. In 10 (37%) of these 79 studies, results could not be reproduced within a predefined difference of the effect size 80 estimate of 0.1. Among these ten meta-analyses, one that had yielded a significant meta- 81 analytic effect was no longer significant, three without significant results yielded significant 82 results when reproduced, and one meta-analysis was retracted. While quality control is 83 important, there are other benefits to reproducible meta-analyses. When meta-analyses are 84 well-documented and transparent, it is easier to re-analyze published meta-analyses using 85 different inclusion criteria. Such re-analyses can yield important insights that differ from the 86 conclusions in the initial meta-analysis. For example, Derry, Derry, McQuay, and Moore 87 (2006) found that even though many systematic reviews had concluded acupuncture was 88 beneficial, their meta-analysis using stricter inclusion criteria (such as randomized, double 89 blind trials) revealed strong evidence that acupuncture had no benefit. 90 Beyond re-analyses, making meta-analytic data publically available facilitates 91 continuously cumulating meta-analyses that update effect size estimates as new data are 92 collected (e.g., Braver, Thoemmes, & Rosenthal, 2014) or as new theoretical viewpoints or 93 statistical techniques are developed. Meta-analyses need to be updated regularly to prevent On the Reproducibility of Meta-Analyses 4 94 outdated scientific conclusions from influencing public policy or real-life applications. 95 Cochrane reviews are required to be updated every two years, or else the lack of an update 96 needs to be justified (The Cochrane Collaboration, 2011). Similarly, authors of Campbell 97 reviews are obliged to plan for an update within five years after publication (The Campbell 98 Collaboration, 2014a). If the data underlying meta-analyses were openly accessible and 99 reproducible, such updates may become more common in psychology than they are now, 100 which would facilitate cumulative knowledge. If meta-analytic efforts are to stand the test of 101 time, it is essential that the extracted meta-analytic data can be easily updated and reanalyzed 102 by researchers in the future. 103 The open accessibility of meta-analytic data may also make these analyses more 104 objective and convincing. A lack of openness about the data and choices for inclusion criteria 105 underlying meta-analyses has been raised as one of the problems in resolving debates 106 following the publication of meta-analyses (e.g., Bushman, Rothstein, & Anderson, 2010). As 107 Ferguson (2014a) argues, “meta-analyses have failed in replacing narrative reviews as more 108 objective”. Researchers on different sides of a scientific argument often reach different 109 conclusions in their meta-analyses of the same literature (see Goodyear-Smith, van Driel, 110 Arroll, & Del Mar, 2012, for detailed discussion of different meta-analyses on depression 111 screening with opposite recommendations). We believe this situation can be improved 112 substantially by implementing six straightforward recommendations that increase the 113 openness and reproducibility of meta-analyses. We have summarized these recommendations 114 in Table 1, and will expand upon each in the remainder of this article. 115 116 Table 1: Six Practical Recommendations to Improve the Reproducibility of 117 Meta-Analyses 118 1. Facilitate Cumulative Science by Future-Proofing Meta-Analyses: 119 Disclose all meta-analytic data (effect sizes, sample sizes for each condition, test 120 statistics and degrees of freedom, means, standard deviations, and correlations 121 between dependent observations) for each data point. Quote relevant text from studies 122 that describe the meta-analytic data to prevent confusion, such as when one effect size 123 is selected from a large number of tests reported in a study. When analyzing 124 subgroups, include quotes from the original study that underlie this classification, and 125 specify any subjective decisions. On the Reproducibility of Meta-Analyses 5 126 2. Facilitate quality control: Specify which effect size calculations are used and 127 which assumptions are made for missing data (e.g., assuming equal sample sizes in 128 each condition, imputed values for unreported effect sizes), if necessary for each 129 effect size extracted from the literature. Specify who extracted and coded the data, 130 knowing it is preferable that two researchers independently extract effect sizes from 131 the literature. 132 3. Adhere to
Details
-
File Typepdf
-
Upload Time-
-
Content LanguagesEnglish
-
Upload UserAnonymous/Not logged-in
-
File Pages24 Page
-
File Size-