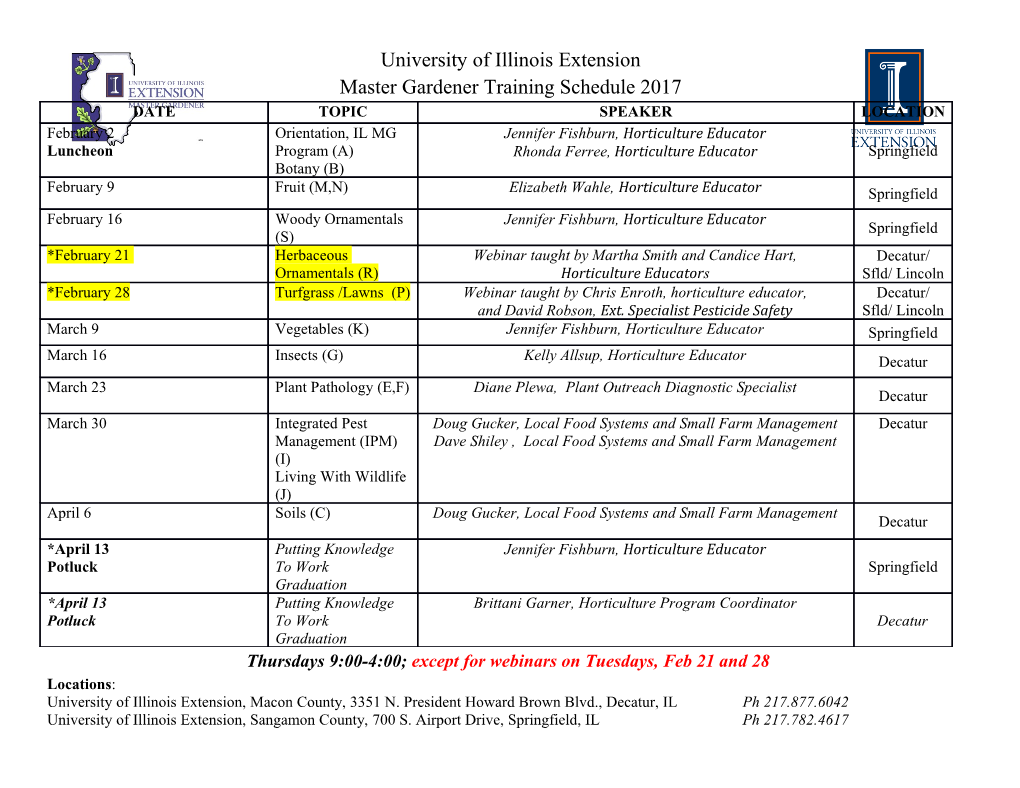
ORIGINAL RESEARCH ARTICLE published: 14 January 2015 doi: 10.3389/fpsyg.2014.01457 Binocular fusion and invariant category learning due to predictive remapping during scanning of a depthful scene with eye movements Stephen Grossberg*, Karthik Srinivasan and Arash Yazdanbakhsh Center for Adaptive Systems, Graduate Program in Cognitive and Neural Systems, Center of Excellence for Learning in Education, Science and Technology, Center for Computational Neuroscience and Neural Technology, and Department of Mathematics, Boston University, Boston, MA, USA Edited by: How does the brain maintain stable fusion of 3D scenes when the eyes move? Every Chris Fields, Independent Scientist, eye movement causes each retinal position to process a different set of scenic features, USA and thus the brain needs to binocularly fuse new combinations of features at each Reviewed by: position after an eye movement. Despite these breaks in retinotopic fusion due to each Greg Francis, Purdue University, USA movement, previously fused representations of a scene in depth often appear stable. The Christopher W. Tyler, 3D ARTSCAN neural model proposes how the brain does this by unifying concepts about Smith-Kettlewell Eye Research how multiple cortical areas in the What and Where cortical streams interact to coordinate Institute, USA processes of 3D boundary and surface perception, spatial attention, invariant object *Correspondence: category learning, predictive remapping, eye movement control, and learned coordinate Stephen Grossberg, Center for Adaptive Systems, Boston transformations. The model explains data from single neuron and psychophysical studies University, 677 Beacon Street, of covert visual attention shifts prior to eye movements. The model further clarifies how Boston, MA 02215, USA perceptual, attentional, and cognitive interactions among multiple brain regions (LGN, V1, e-mail: [email protected] V2, V3A, V4, MT, MST, PPC, LIP, ITp, ITa, SC) may accomplish predictive remapping as part of the process whereby view-invariant object categories are learned. These results build upon earlier neural models of 3D vision and figure-ground separation and the learning of invariant object categories as the eyes freely scan a scene. A key process concerns how an object’s surface representation generates a form-fitting distribution of spatial attention, or attentional shroud, in parietal cortex that helps maintain the stability of multiple perceptual and cognitive processes. Predictive eye movement signals maintain the stability of the shroud, as well as of binocularly fused perceptual boundaries and surface representations. Keywords: depth perception, perceptual stability, predictive remapping, saccadic eye movements, object recognition, spatial attention, gain fields, category learning 1. INTRODUCTION boundaries and surfaces are formed from 3D scenes and 2D pic- 1.1. STABILITY OF 3D PERCEPTS ACROSS EYE MOVEMENTS tures that may include partially occluding objects (Grossberg, Our eyes continually move from place to place as they scan a scene 1994, 1997; Grossberg and McLoughlin, 1997; Grossberg and to fixate different objects with their high resolution foveal rep- Kelly, 1999; Kelly and Grossberg, 2000; Grossberg et al., resentations. Despite the evanescent nature of each fixation, we 2002, 2007, 2008; Grossberg and Swaminathan, 2004; Cao and perceive the world continuously in depth. Such percepts require Grossberg, 2005, 2012; Grossberg and Yazdanbakhsh, 2005; Fang explanation, if only because each eye movement causes the fovea and Grossberg, 2009). The articles that develop FACADE also to process a different set of scenic features, and thus there are summarize and simulate perceptual and neurobiological data breaks in retinotopic fusion due to each movement. Within a supporting the model’s prediction that 3D boundary and surface considerable range of distances and directions of movement, the representations are, indeed, the perceptual units of 3D vision. fused scene appears stable in depth, despite the fact that new aFILM (Anchored Filling-In Lightness Model) simulates psy- retinotopic matches occur after each movement. How does the chophysical data about how the brain generates representations of brain convert such intermittent fusions into a stable 3D percept anchored lightness and color in response to psychophysical dis- that persists across eye movements? plays and natural scenes (Hong and Grossberg, 2004; Grossberg This article develops the 3D ARTSCAN model to explain and and Hong, 2006). simulate how the brain does this, and makes several predictions ARTSCAN (Grossberg, 2007, 2009; Fazl et al., 2009)models to further test model properties. The model builds upon and and simulates perceptual, attentional, and neurobiological data integrates concepts and mechanisms from earlier models: about how the brain can coordinate spatial and object attention FACADE (Form-And-Color-And-DEpth) is a theory of 3D across the Where and What cortical streams to learn and recog- vision and figure-ground separation that proposes how 3D nize view-invariant object category representations as it scans a www.frontiersin.org January 2015 | Volume 5 | Article 1457 | 1 Grossberg et al. Binocular fusion during eye movements 2D scene with eye movements. These category representations The main theoretical goal of the current article is to demon- form in the inferotemporal cortex in response to 2D boundary strate the property of perceptual stability of 3D visual boundaries and surface representations that form across several parts of the and surfaces across saccadic eye movements, which has been clar- visual cortex. In order to learn view-invariant object categories, ified using a variety of experimental paradigms (Irwin, 1991; the model showed how spatial attention maintains its stability in Carlson-Radvansky, 1999; Cavanagh et al., 2001; Fecteau and head-centered coordinates during eye movements as a result of Munoz, 2003; Henderson and Hollingworth, 2003; Beauvillain the action of eye-position-sensitive gain fields. et al., 2005). The article also predicts how this process interacts These earlier models did not, however, consider how 3D with processes of spatial and object attention, invariant object cat- boundary and surface representations that are formed from egory learning, predictive remapping, and eye movement control, binocularly fused information from the two eyes is maintained as notably how they all regulate and/or respond to adaptive coordi- theeyesmovetofixatedifferentsetsofobjectfeatures.Thecur- nate transformations. As explained more fully below, the brain rent article shows how the stability of 3D boundary and surface can prevent a break in binocular fusion after an eye movement representations and of spatial attention are ensured using gain occurs by using predictive gain fields to maintain 3D boundary fields. With this new competence incorporated, the 3D ARTSCAN and surface representations in head-centered coordinates, even model can learn view-invariant object representations as the eyes though these representations are not maintained in retinotopic scan a depthful scene. coordinates. This property is demonstrated by simulations using 3D ARTSCAN is also consistent with the pARTSCAN (posi- 2D geometrical shapes and natural objects that are viewed in tional ARTSCAN) model (Cao et al., 2011), which clarifies how an 3D. In particular, the simulations show that the 3D boundary observer can learn both positionally-invariant and view-invariant and surface representations of these objects are maintained in object categories in a 2D scene; the dARTSCAN (distributed head-centered coordinates as the eyes move. ARTSCAN) model (Foley et al., 2012), which clarifies how These simulation results generalize immediately to 3D objects visual backgrounds do not become dark when spatial attention that have multiple 2D planar surfaces, since the simulations due is focused on a particular object, how Where stream transient not depend upon a particular binocular disparity. Indeed, other attentional components and What stream sustained attentional modeling studies have demonstrated how the same retinotopic components interact, and how prefrontal priming interacts with binocular mechanisms can process object features at multiple dis- parietal attention mechanisms to influence search efficiency; and parities (Grossberg and McLoughlin, 1997; Grossberg and Howe, the ARTSCAN Search model (Chang et al., 2014), which, in 2003; Cao and Grossberg, 2005, 2012), including objects per- addition to supporting view- and positionally-invariant object ceived from viewing stereograms (Fang and Grossberg, 2009)and category learning and recognition using Where-to-What stream natural 3D scenes (Cao and Grossberg, submitted), as well as interactions, can also search a scene for a valued goal object objects that are slanted in depth (Grossberg and Swaminathan, using reinforcement learning, cognitive-emotional interactions, 2004). All these results should be preserved under the action and What-to-Where stream interactions. It thereby proposes a of predictive gain fields to convert their retinotopic boundary neurobiologically-grounded solution of the Where’s Waldo prob- and surface representations into head-centered ones, since the lem. With the capacity of searching objects in depth added, gain fields merely predictively shift the representations that are which the results hereby about 3D perceptual stability permit, created by the retinotopic mechanisms. The key point is thus a 3D ARTSCAN Search model could learn and recognize
Details
-
File Typepdf
-
Upload Time-
-
Content LanguagesEnglish
-
Upload UserAnonymous/Not logged-in
-
File Pages41 Page
-
File Size-