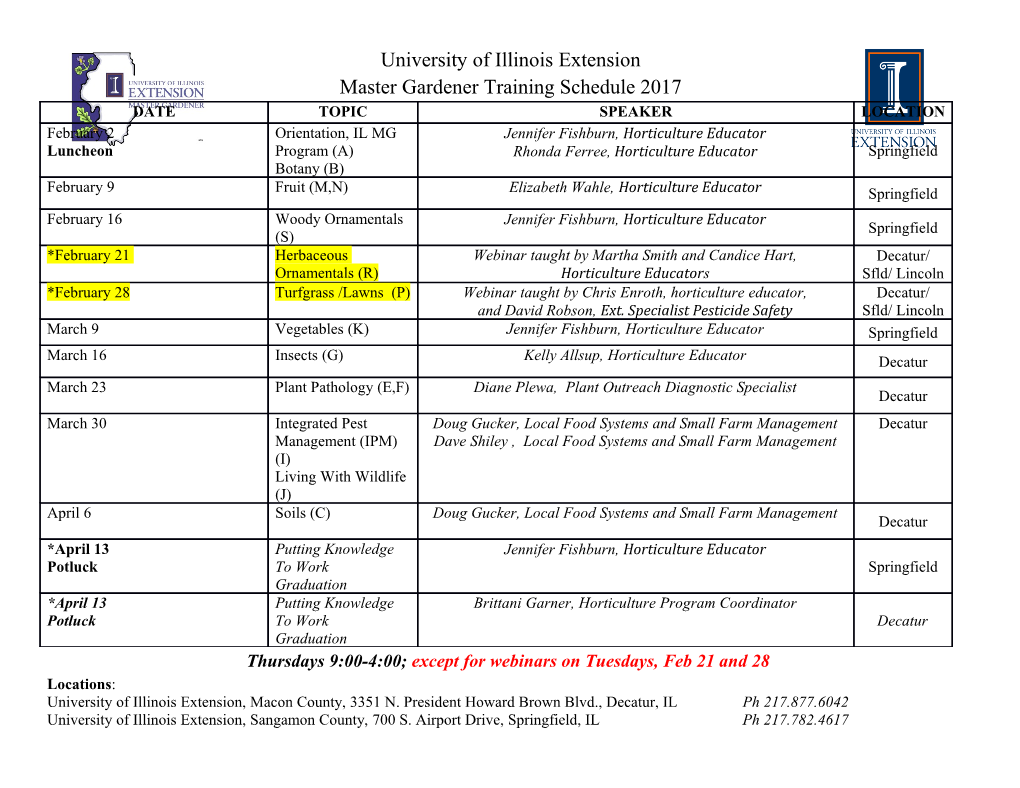
Identification and Recognition of Remote-Controlled Malware Inauguraldissertation zur Erlangung des akademischen Grades eines Doktors der Naturwissenschaften der Universität Mannheim vorgelegt von Christian Jörn Dietrich aus Düsseldorf, Deutschland Mannheim, 2012 Dekan: Prof. Dr. Heinz Jürgen Müller, Universität Mannheim Referent: Prof. Dr. Felix Christoph Freiling, Universität Erlangen-Nürnberg Korreferent: Prof. Dr. Christopher Kruegel, University of California, Santa Barbara Tag der mündlichen Prüfung: 28. März 2013 2 Abstract Remote-controlled malware, organized in so-called botnets, have emerged as one of the most prolific kinds of malicious software. Although numbers vary, in ex- treme cases such as Conficker, Bredolab and Mariposa, one botnet can span up to several million infected computers. This way, attackers draw substantial revenue by monetizing their bot-infected computers. This thesis encapsulates research on the detection of botnets – a required step towards the mitigation of botnets. First, we design and implement Sandnet, an observation and monitoring infrastructure to study the botnet phenomenon. Using the results of Sandnet, we evaluate detection approaches based on traffic analysis and rogue visual monetization. While traditionally, malware authors designed their botnet command and con- trol channels to be based on plaintext protocols such as IRC, nowadays, botnets leverage obfuscation and encryption of their C&C messages. This renders meth- ods which use characteristic recurring payload bytes ineffective. In addition, we observe a trend towards distributed C&C architectures and nomadic behavior of C&C servers in botnets with a centralized C&C architecture, rendering blacklists infeasible. Therefore, we identify and recognize botnet C&C channels by help of traffic analysis. To a large degree, our clustering and classification leverage the sequence of message lengths per flow. As a result, our implementation, called CoCoSpot, proves to reliably detect active C&C communication of a variety of botnet families, even in face of fully encrypted C&C messages. Furthermore, we observe that botmasters design their C&C channels in a more stealthy manner so that the identification of C&C channels becomes even more difficult. Indeed, with Feederbot we found a botnet that uses DNS as carrier protocol for its command and control channel. By help of statistical entropy as well as behavioral features, we design and implement a classifier that detects DNS- based C&C, even in mixed network traffic of benign users. Using our classifier, we even detect another botnet family which uses DNS as carrier protocol for its command and control. Finally, we show that a recent trend of botnets consists in rogue visual mon- etization. Perceptual clustering of Sandnet screenshots enables us to group malware into rogue visual monetization campaigns and study their localization as well as monetization properties. i Zusammenfassung Fernsteuerbare Schadsoftware, zusammengeschaltet in sog. Botnetzen, hat sich mittlerweile zu einer sehr verbreiteten Art an Schadsoftware entwickelt. Obwohl die genauen Zahlen mitunter schwanken, so zeigt sich in Extremfällen wie etwa bei Conficker, Bredolab und Mariposa, dass ein einzelnes Botnetz aus infizierten Computern mit bis zu zweistelliger Millionenanzahl besteht. Die Angreifer er- wirtschaften somit erhebliche Einkommen, indem sie die infizierten Computer monetarisieren. Diese Arbeit umfasst Forschungsarbeiten zur Erkennung von Botnetzen – ein notwendiger Schritt, um Botnetze zu entschärfen. Zunächst entwerfen und imple- mentieren wir die Beobachtungsumgebung Sandnet, um das Botnetz-Phänomen detailliert untersuchen zu können. Mit Hilfe der Ergebnisse des Sandnet ent- werfen und bewerten wir Erkennungsmechanismen, die sowohl auf Verkehrsflus- sanalyse des Netzwerkverkehrs als auch auf dem visuellen Eindruck der bösartigen Benutzerschnittstelle basieren. Während Schadsoftware-Autoren in der Vergangenheit die Steuerkanäle (C&C) ihrer Botnetze häufig unter Verwendung von Klartext-Protokollen wie etwa IRC entworfen haben, so werden neuerdings fast ausschließlich verschleierte oder ver- schlüsselte C&C-Nachrichten verwendet. Dies verhindert Erkennungsmechanis- men, die auf charakteristischen, wiederkehrenden Nutzdaten-Mustern basieren. Darüber hinaus lässt sich ein Trend hin zu verteilten C&C-Architekturen sowie ein nomadisches Umzugsverhalten der C&C-Server im Falle von Botnetzen mit zentralisierter C&C-Architektur erkennen. Auf diese Weise werden Blacklists von C&C-Endpunkten umgangen. Wir entwickeln daher einen Ansatz zur Identifika- tion und Wiedererkennung von Botnetz-C&C-Kanälen mit Hilfe von Verkehrs- flussanalyse. Unser Ansatz basiert dabei in erster Linie auf der Sequenz von Nachrichtenlängen einer Netzwerkverbindung. In der Evaluation beweist un- sere Implementierung CoCoSpot, dass sie auf verlässliche Art und Weise C&C- Kommunikation einer Vielzahl an verschiedenen Botnetz-Familien erkennen kann, selbst wenn die C&C-Nachrichten vollständig verschlüsselt sind. Ferner beobachten wir, dass Botmaster ihre C&C-Kanäle unter erheblicher Berücksichtigung der Tarnung im Netzwerkverkehr entwerfen. Mit Feederbot zeigen wir, dass mittlerweile Botnetze existieren, die DNS als Trägerprotokoll für ihren C&C-Kanal verwenden. Mit Hilfe der statistischen Entropie sowie Ver- iii Zusammenfassung haltenseigenheiten wird ein Klassifizierer entworfen und implementiert, der DNS- basierte C&C-Kanäle erkennen kann – selbst in gemischtem Netzwerkverkehr von legitimen Benutzern. Unter Verwendung unseres Klassifizierers entdecken wir sogar eine weitere Botnet-Familie, die DNS als Trägerprotokoll für ihren C&C benutzt. Schließlich zeigen wir, dass ein aktueller Trend in der sog. rogue visual moneti- zation liegt. Ein wahrnehmungsbasiertes Clustering von Screenshots des Sand- net ermöglicht es uns, Schadsoftware in Kampagnen der rogue visual monetiza- tion zu gruppieren und die Eigenschaften ihrer Lokalisierung und Monetarisierung zu studieren. iv Acknowledgements This thesis would have hardly been possible without the help and support of others. First of all, I would like to thank my supervisor Felix C. Freiling who by his guidance has significantly encouraged me and fostered my research. I have always enjoyed our inspiring discussions and appreciated your kind advice. Your positive outlook and kindness inspired me and gave me confidence. I am deeply grateful to my colleague and friend Christian Rossow. Christian, you are — by far — the most remarkable, sharp-minded, humble and encouraging person I have ever worked with. It has been a great pleasure working with you. Furthermore, I am thankful to Norbert Pohlmann, for supporting me over many years and giving me the opportunity to work in the inspiring environment at his Institute. I appreciate to have learned quite some lessons not limited to academic matters. Moreover, I thank the team at the Institute for Internet Security at the Univer- sity of Applied Sciences Gelsenkirchen, in particular Christian Nordlohne, who supported me with the analysis that influenced the C&C flow fingerprinting in this thesis. Many thanks also to my second supervisor Christopher Kruegel. I really en- joyed to work with you and the people in UCSB’s seclab. I am especially thankful to — alphabetically ordered — Adam Doupé, Yanick Fratantonio, Alexandros Kapravelos, Gianluca Stringhini and Ali Zand. Furthermore, I would like to thank everyone at Lastline. A big thank you to my friend Philipp von Cube who bore with me for many discussions and always supported me in times of decision-making. To conclude, I wish to extend a huge thank you to my parents and my brother for their help and support all the way. You always supported all the decisions I made, without asking for anything in return. Without your care and discipline as well as the ways you have paved, I would simply not be the person I am today. Thank you very much for this. Last but not least I would like to thank my dear Julia. You have always been (and will always be) the one to make my day. I am deeply grateful not only for your inspiration and advice, but also for your love. This dissertation is dedicated to you. v Contents Abstracti Zusammenfassung iii Acknowledgementsv 1 Introduction1 1.1 Motivation...............................1 1.2 Contributions: Countering Deviance.................2 1.2.1 Detection := Identification + Recognition.........4 1.2.2 List of Contributions.....................5 1.3 Thesis Outline.............................7 1.4 List of Publications..........................8 2 Background 11 2.1 Remote-Controlled Malware..................... 11 2.2 Machine Learning for Malware Detection.............. 14 2.2.1 Clustering Evaluation..................... 18 2.2.2 Classification Evaluation................... 20 2.3 Summary............................... 21 3 Malware Analysis using Sandnet 23 3.1 Introduction.............................. 23 3.2 Sandnet Architecture........................ 24 3.3 Segmentation and Dissection of Network Traffic.......... 26 3.4 Visualization............................. 29 3.5 Dataset Overview and Evaluation.................. 31 3.6 Conclusion............................... 38 4 Recognition of Command and Control Flows 41 4.1 Introduction and Problem Statement................ 41 vii Contents 4.2 Related Work............................. 47 4.3 Methodology............................. 49 4.3.1 Traffic Analysis Features of Botnet C&C.......... 50 4.4 Clustering Analysis of Known C&C Flows............. 51 4.4.1 Definition of the Distance
Details
-
File Typepdf
-
Upload Time-
-
Content LanguagesEnglish
-
Upload UserAnonymous/Not logged-in
-
File Pages139 Page
-
File Size-