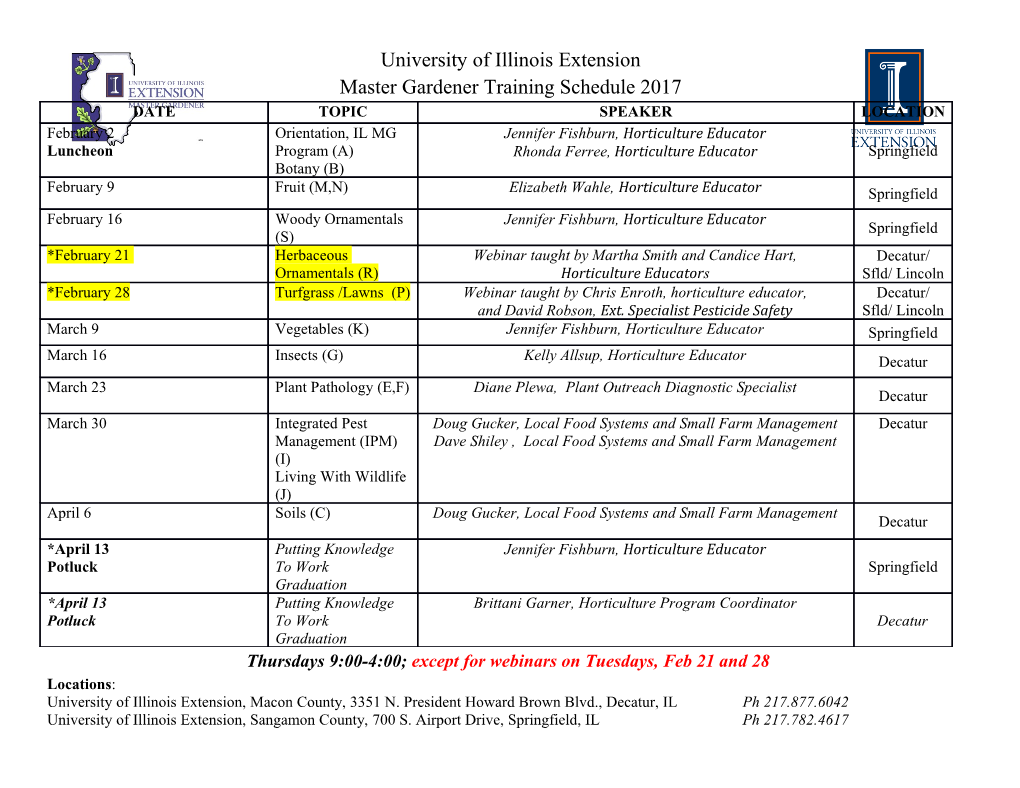
Don’t Just Scratch the Surface: Enhancing Word Representations for Korean with Hanja Kang Min Yoo∗, Taeuk Kim∗ and Sang-goo Lee Department of Computer Science and Engineering Seoul National University, Seoul, Korea fkangminyoo,taeuk,[email protected] Abstract We propose a simple yet effective approach for improving Korean word representations using additional linguistic annotation (i.e. Hanja). We employ cross-lingual transfer learning in training word representations by leveraging the fact that Hanja is closely related to Chi- nese. We evaluate the intrinsic quality of rep- resentations learned through our approach us- ing the word analogy and similarity tests. In Figure 1: An example of a Korean word showing its addition, we demonstrate their effectiveness form and multi-level meanings. The Sino-Korean word KR on several downstream tasks, including a novel consists of Hangul phonograms ( ) and Hanja lo- HJ Korean news headline generation task. gograms ( ). Although annotation of Hanja is op- tional, it offers deeper insight into the word meaning due to its association with the Chinese characters (CN). 1 Introduction There is a strong connection between the Korean Information Skip-Gram), for capturing the seman- and Chinese languages due to cultural and histori- tics of Hanja and subword structures of Korean cal reasons (Lee and Ramsey, 2011). Specifically, and introducing them into the vector space. Note a set of logograms with very similar forms to the that it is also quite intuitive for native Koreans 1 Chinese characters, called Hanja , served in the to resolve the ambiguity of (Sino-)Korean words past as the only medium for written Korean until with the aid of Hanja. We conjecture this heuris- Hangul, the Korean alphabet, and Jamo came into tic is attributed to the fact that Hanja characters existence in 1443 (Sohn, 2001). Considering this are logograms, each of which contains more lex- etymological background, a substantial portion of ical meaning when compared against its counter- Korean words are classified as Sino-Korean, a set part, the Hangul character, which is a phonogram. of Korean words that had originated from Chinese Accordingly, in this work, we focus on exploiting and can be expressed in both Hanja and Hangul the rich extra information from Hanja as a means (Taylor, 1997), with the latter becoming common- of constructing better Korean word embeddings. place in modern Korean. Furthermore, our approach enables us to empir- Based on these facts, we assume the introduc- ically investigate the potential of character-level tion of Hanja-level information will aid in ground- cross-lingual knowledge transfer with a case study ing better representation for the meaning of Ko- of Chinese and Hanja. rean words (e.g. see Fig.1). To validate our To sum up, our contributions are threefold: hypothesis, we propose a simple yet effective ap- proach to training Korean word representations, • We introduce a novel way of training Korean named Hanja-level SISG (Hanja-level Subword word embeddings with an expanded Korean alphabet vocabulary using Hanja in addition ∗Equal contribution. 1Hanja and traditional Chinese characters are very similar to the existing Korean characters, Hangul. but not completely identical. Some differences in strokes and language-exclusive characters exist. • We also explore the possibility of character- 3528 Proceedings of the 2019 Conference on Empirical Methods in Natural Language Processing and the 9th International Joint Conference on Natural Language Processing, pages 3528–3533, Hong Kong, China, November 3–7, 2019. c 2019 Association for Computational Linguistics level knowledge transfer between two lan- However, due to the deconstructible structure guages by initializing Hanja embeddings of Korean characters and the agglutinative na- with Chinese character embeddings before ture of the language, the model proposed by Bo- training skip-gram. janowski et al.(2017) comes short in capturing sub-character and inter-character information spe- • We prove the effectiveness of our method in cific to Korean understanding. As a remedy, the two ways, one of which is intrinsic evaluation model proposed by Park et al.(2018) introduces (the word analogy and similarity tests), and jamo-level n-grams g(j), whose vectors can be as- the other being two downstream tasks includ- sociated with the target context as well. Given that ing a newly proposed Korean news headline a Korean word w can be split into a set of jamo generation task. (j) (j) n (j) (j)o n-grams Gw ⊂ G = g1 ; : : : ; gJ , where 2 Model Description G(j) is the set of all possible jamo n-grams in the corpus, the updated scoring function for jamo- 2.1 Skip-Gram (SG) level skip-gram s(j) is thus Given a corpus as a sequence of words (w1; w2; : : : ; wT ), the goal of a skip-gram model (j) X (Mikolov et al., 2013) is to maximize the log- s (w; c) = s (w; c) + zjvc: (4) (j) probabilities of context words given a target word j2Gw wi: 2.3 Hanja-level SISG2 X X log p (wcjwi); (1) Another semantic level in Korean lies in Hanja i2f1;:::;T g c2C(i) logograms. Hanja characters can be mapped to where C returns a set of context word indices given Hangul phonograms, hence Hanja annotations are a word index. The usual choice for training the sufficient but not necessary in conveying mean- parameterized probability function p (wcjw) is to ings (Fig.1). As each Hanja character is associ- reframe the problem as a set of binary classifica- ated with semantics, their presence could provide tion tasks of the target context word c and other meaningful aid in capturing word-level semantics negative samples chosen from the entire dictionary in the vector space. (negative sampling). The objective is thus We propose incorporating Hanja n-grams in the learning of word representations by allowing the X scoring function to associate each Hanja n-gram L (s (wi; wc)) + L (−s (wi; wj)) ; (2) with the target context word. Concretely, given j2N (i;c) that a Korean word w contains a set of Hanja se- quences H = (h ; : : : ; h ) (e.g. for the where L (x) = log (1 + e−x), the binary logis- w w;1 w;Hw example in Fig.1 h = “>會” and h = “型”) tic loss. N returns a set of negative sample in- w;1 w2 and that I is the set of Hanja n-grams for a Hanja dices given target word and context indices. In the h sequence h (e.g. Ihw;2 = f “<boh>型”, “型”, vanilla SG model, the scoring function s is the dot (h) “型<eoh>”g), all Hanja n-grams Gw for word product between the two word vectors: vw and vc. w are the union of Hanja n-grams of Hanja se- 2.2 Subword Information SG (SISG) and S quences present in w: h2Hw Ih. The length of Jamo-level SISG Hanja n-grams in Ih is a hyperparameter. Then In Bojanowski et al.(2017), the scoring func- the new score function for Hanja-level SISG is tion incorporates subword information. Specifi- cally, given all possible n-gram character sets G = (h) (j) X s (w; c) = s (w; c) + zhvc (5) fg1; : : : ; gGg in the corpus and that the n-gram set h2G(h) of a particular word w is Gw ⊂ G, the scoring w function is thus the sum of dot products between where z is the n-gram vector for a Hanja se- all n-gram vectors z and the context vector v : h c quence h. X 2 s (w; c) = zgvc: (3) The code and models are publicly available online at https://github.com/kaniblu/hanja-sisg g2Gw 3529 Analogy Similarity 2.4 Character-level Knowledge Transfer Method Sem. Syn. All Pr. Sp. Since Hanja characters essentially share deep SISG(cj)y 0.47 0.38 0.43 - 0.67 roots with Chinese characters, many of them can SG 0.42 0.49 0.45 0.60 0.62 SISG(c) 0.45 0.59 0.52 0.62 0.61 be mapped by one-to-one correspondence. By SISG(cj) 0.39 0.48 0.44 0.66 0.67 converting Korean characters into simplified Chi- SISG(cj)z 0.41 0.48 0.45 0.65 0.67 nese characters or vice versa, it is possible to uti- SISG(cjh3) 0.34 0.45 0.39 0.63 0.63 lize pre-trained Chinese embeddings in the pro- SISG(cjh4) 0.34 0.45 0.40 0.62 0.61 SISG(cjhr) 0.35 0.46 0.40 0.65 0.64 cess of training Hanja-level SISG. In our case, we y reported by Park et al.(2018) propose leveraging advances in Chinese word em- z pre-trained embeddings provided by the authors of beddings from the Chinese community by initial- Park et al.(2018) run with our evaluation script izing Hanja n-grams vectors zh with the state-of- the-art Chinese embeddings (Li et al., 2018) and Table 1: Word analogy and similarity evaluation re- training with the score function in Equation5. sults. For word analogy test, the table shows cosine distances averaged over semantic and syntactic word pairs (lower is better). For word similarity test, we 3 Experiments report on Pearson and Spearman correlations between 3.1 Word Representation Learning predictions and ground truth scores. We observe that our approach (SISG(cjh)) shows significant improve- 3.1.1 Korean Corpus ments in the analogy test but some deterioration in the For learning word representations, we utilize the similarity test. Note that the evaluation results reported by the original authors (y) are largely different from corpus prepared by Park et al.(2018) with small our results.
Details
-
File Typepdf
-
Upload Time-
-
Content LanguagesEnglish
-
Upload UserAnonymous/Not logged-in
-
File Pages6 Page
-
File Size-