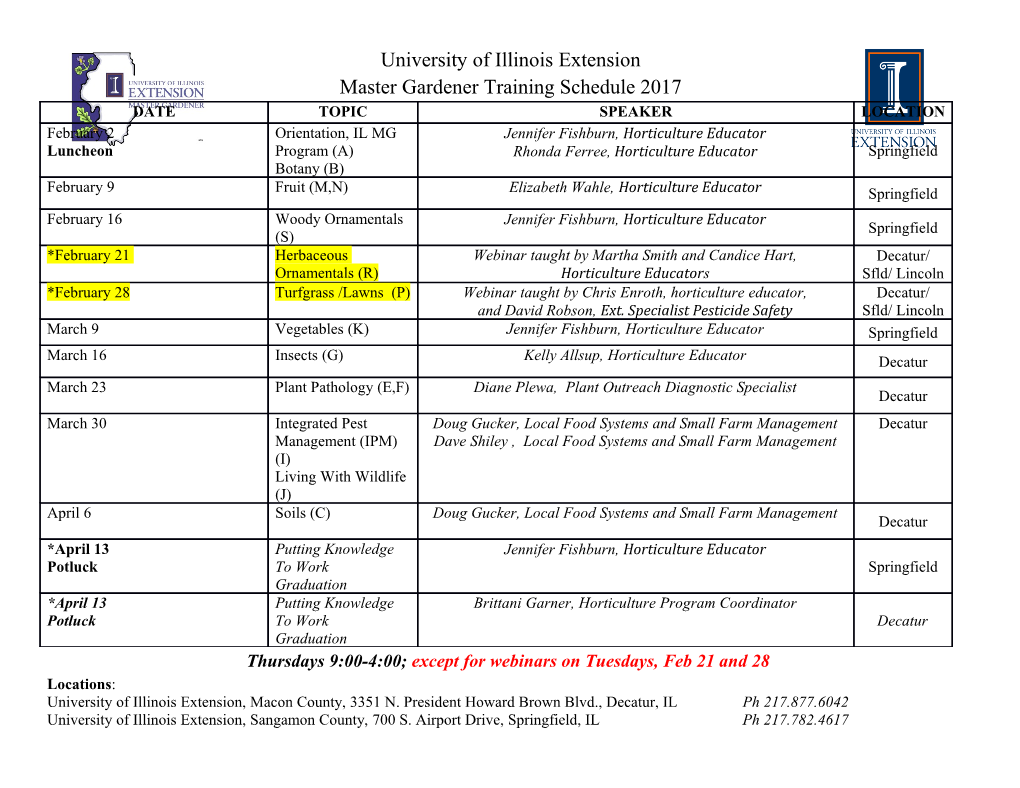
Forschungsgemeinschaft through through Forschungsgemeinschaft SFB 649DiscussionPaper2006-001 * CASE - Center for Applied Statistics and Economics, Statisticsand Center forApplied - * CASE Calibration Riskfor This research was supported by the Deutsche the Deutsche by was supported This research Wolfgang K.Härdle** Humboldt-Universität zuBerlin,Germany SFB 649, Humboldt-Universität zu Berlin zu SFB 649,Humboldt-Universität Exotic Options Spandauer Straße 1,D-10178 Berlin Spandauer http://sfb649.wiwi.hu-berlin.de http://sfb649.wiwi.hu-berlin.de Kai Detlefsen* ISSN 1860-5664 the SFB 649 "Economic Risk". "Economic the SFB649 SFB 6 4 9 E C O N O M I C R I S K B E R L I N Calibration Risk for Exotic Options K. Detlefsen and W. K. H¨ardle CASE - Center for Applied Statistics and Economics Humboldt-Universit¨atzu Berlin Wirtschaftswissenschaftliche Fakult¨at Spandauer Strasse 1, 10178 Berlin, Germany Abstract Option pricing models are calibrated to market data of plain vanil- las by minimization of an error functional. From the economic view- point, there are several possibilities to measure the error between the market and the model. These different specifications of the error give rise to different sets of calibrated model parameters and the resulting prices of exotic options vary significantly. These price differences often exceed the usual profit margin of exotic options. We provide evidence for this calibration risk in a time series of DAX implied volatility surfaces from April 2003 to March 2004. We analyze in the Heston and in the Bates model factors influencing these price differences of exotic options and finally recommend an error func- tional. Moreover, we determine the model risk of these two stochastic volatility models for the time series and consider its relation to cali- bration risk. Key Words: calibration risk, calibration, model risk, Heston model, Bates model, barrier option, cliquet option JEL Classification: C13, G12 Acknowledgement: This research was supported by Deutsche Forschungsgemeinschaft through the SFB 649 ”Economic Risk” and by Bankhaus Sal. Oppenheim. The authors would like to thank Bernd Engelmann and Peter Schwendner for helpful discussions. 1 Introduction Recently, there has been a considerable interest, both from a practical and a theoretical point of view, in the risks involved in option pric- ing. Schoutens et al. (2004) have analyzed model risk in an empirical study and Cont (2004) has put this risk into a theoretical framework. Another source of risk is hidden in the calibration of models to market data. This calibration risk exceeds often the profit margin for exotic options and hence is also of fundamental interest for the banking in- dustry. Moreover, calibration risk exists even if an appropriate model has been chosen and model risk does not exist anymore. Calibration risk arises from the different possibilities to measure the error between the observations on the market and the correspond- ing quantities in the model world. A natural approach to specify this error is to consider the absolute price (AP) differences, see e.g. Schoutens et al. (2004). But the importance of absolute price differ- ences depends on the magnitude of these price. Hence, another useful way for measuring the error are relative price (RP) differences, see e.g. Mikhailov et al. (2003). As models are often judged by their capability to reproduce implied volatility surfaces other measures can be defined in terms of implied volatilities. There are again the two possibilities of absolute implied volatilities (AI) and relative implied volatilities (RI). We consider these four ways to measure the difference between model and market data and explore the implications for the pricing of exotic options. To this end, we focus on the popular stochastic volatility model of Heston. In order to analyze the influence of the goodness of fit on calibration risk we consider in addition the Bates model which is an extension of the Heston model with similar qualitative features. These two models are calibrated to plain vanillas on the DAX. In order to get reliable results we use a time series of implied volatility surfaces from April 2003 to March 2004. Because of the computationally in- tense Monte Carlo simulations for the pricing of the exotic options we consider only one trading day in each week of this period. As exotic options we consider down and out puts, up and out calls and cliquet options for 1, 2 or 3 years to maturity. In this framework we determine the size of calibration risk and analyze factors influencing it. Besides calibration risk there is also model risk which represents wrong prices because a wrong parametric model has been chosen. We consider also model risk between the Heston and the Bates model and 1 analyze the relation between the two forms of risk in pricing exotic options. Section 2 introduces the models and describes their risk neutral dynamics that we use for option pricing. Moreover, this section con- tains information about the data used for the calibration. Section 3 describes the calibration method and defines the error functionals an- alyzed in this work. The goodness of fit is shown by representative surfaces and statistics on the errors. In Section 4, we present the exotic options that we consider for calibration risk and price these products by simulation. In Section 5, we analyze the model risk for the two stochastic volatility models under the four error functionals. In the last Section 6, we summarize the results and draw our conclusions. 2 Models and Data In this section, we describe briefly the Heston model and the Bates model for which we are going to analyze calibration risk. Moreover, we provide some descriptive statistics of the implied volatility surfaces that we use as input data for the calibration. 2.1 Heston model We consider the popular stochastic volatility model of Heston (1993): dSt p 1 = µdt + VtdWt St where the volatility process is modelled by a square-root process: p 2 dVt = ξ(η − Vt)dt + θ VtdWt and W 1 and W 2 are Wiener processes with correlation ρ. The volatility process remains positive if its volatility θ is small enough with respect to the product of the mean reversion speed ξ and the average volatility level η: θ2 ξη > . (1) 2 2 The dynamics of the price process are analyzed under a martingale measure under which the characteristic function of log(St) is given by: −(z2 + iz)V φH (z) = exp{ 0 } t γ(z)t γ(z) coth 2 + ξ − iρθz ξηt(ξ−iρθz) exp{ 2 + iztr + iz log(S0)} × θ γ(z)t ξ−iρθz γ(z)t 2ξη θ2 (cosh 2 + γ(z) sinh 2 ) where γ(z) def= pθ2(z2 + iz) + (ξ − iρθz)2, see e.g. Cont et al. (2004). 2.2 Bates model Bates (1996) extended the Heston model by considering jumps in the stock price process: dSt p 1 = µdt + VtdWt + dZt St p 2 dVt = ξ(η − Vt)dt + θ VtdWt where Z is a compound Poisson process with intensity λ and jumps k that have a lognormal distribution: δ2 log(1 + k) N(log(1 + k) − , δ2). ∼ 2 We analyze the dynamics of this model under a martingale measure under which the characteristic function of log(St) is given by: B −δ2z2/2+i{log(1+k)− 1 δ2}z φt (z) = exp{tλ(e 2 − 1)} −(z2 + iz)V × exp{ 0 } γ(z)t γ(z) coth 2 + ξ − iρθz ξηt(ξ−iρθz) exp{ 2 + izt(r − k) + iz log(S0)} × θ γ(z)t ξ−iρθz γ(z)t 2ξη θ2 (cosh 2 + γ(z) sinh 2 ) where γ(z) def= pθ2(z2 + iz) + (ξ − iρθz)2, see e.g. Cont et al. (2004). The Bates model has eight parameters while the Heston model has only five parameters. Because of these three additional parameters the Bates model can better fit observed surface but parameter stability is more difficult to achieve. 3 mean number mean number mean money- of maturities of obervations ness range short maturities 3.06 64 0.553 (0.25 ≤ T < 1.0) long maturities 5.98 76 0.699 (1.0 ≤ T ) total 9.04 140 0.649 Table 1: Description of the implied volatility surfaces. 2.3 Data Our data consists of EUREX-settlement volatilities of European op- tions on the DAX. We consider the time period from April 2003 to March 2004. Since March 2003 the EUREX trades plain vanillas with maturities up to 5 years. Until March 2004 it has not changed its range of products. Hence, the data is homogeneous in sense that the implied volatility surfaces are derived from similar products. From this time period we analyze the surfaces from all the Wednes- days when trading has taken place. Thus, we consider 51 implied volatility surfaces. We exclude observations that are deep out of the money because of illiquidity of these products. More precisely, we con- sider only options with moneyness m = K/S0 ∈ [0.75, 1.35] for small times to maturity T ≤ 1. As we analyze exotic options with maturity in 1, 2 or 3 years we exclude also plain vanillas with time to maturity less than 3 months. Some information about the resulting implied volatility surfaces are summarized in table 1. The surfaces contain in the mean 140 transformed prices and nine maturities with a mean moneyness range of 65%. The values of the underlying in the considered period are shown in figure 1. This figure contains also the (interpolated) implied volatilities for 1 year to maturity with strike at spot level. Hence, the market of the DAX went up in this period while the implied volatilities went down as figure 1 shows. We approximate the risk free interest rates by the EURIBOR.
Details
-
File Typepdf
-
Upload Time-
-
Content LanguagesEnglish
-
Upload UserAnonymous/Not logged-in
-
File Pages30 Page
-
File Size-