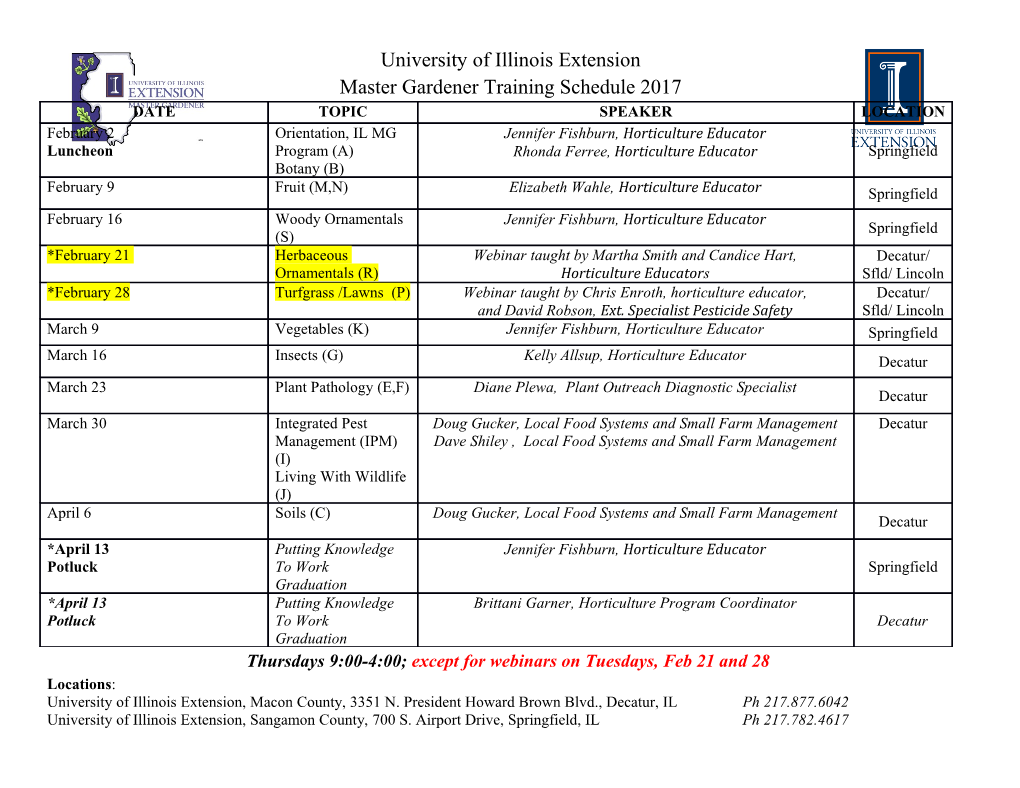
Combining Depth, Color and Position Information for Object Instance Recognition on an Indoor Mobile Robot Louis-Charles Caron To cite this version: Louis-Charles Caron. Combining Depth, Color and Position Information for Object Instance Recog- nition on an Indoor Mobile Robot. Computer Vision and Pattern Recognition [cs.CV]. ENSTA Paris- Tech, 2015. English. tel-01251481 HAL Id: tel-01251481 https://hal.archives-ouvertes.fr/tel-01251481 Submitted on 6 Jan 2016 HAL is a multi-disciplinary open access L’archive ouverte pluridisciplinaire HAL, est archive for the deposit and dissemination of sci- destinée au dépôt et à la diffusion de documents entific research documents, whether they are pub- scientifiques de niveau recherche, publiés ou non, lished or not. The documents may come from émanant des établissements d’enseignement et de teaching and research institutions in France or recherche français ou étrangers, des laboratoires abroad, or from public or private research centers. publics ou privés. Thèse Combining Depth, Color and Position Information for Object Instance Recognition on an Indoor Mobile Robot Présentée à l’Unité d’Informatique et d’Ingénierie des Systèmes pour l’obtention du grade de Docteur de l’ENSTA ParisTech École Nationale Supérieure des Techniques Avancées Spécialité : Informatique par Louis-Charles Caron Thèse soutenue le 4 novembre 2015 devant le jury composé de Président: Youcef Mezouar Professeur — Institut Pascal Clermont-Ferrand Rapporteur: Fabien Moutarde Maître-assistant HdR — Mines ParisTech Rapporteur: Michel Devy Directeur de Recherche — LAAS-CNRS Examinateur: Guy Le Besnerais Maître de recherches HdR — ONERA Superviseur: David Filliat Professeur — ENSTA ParisTech Co-superviseur: Alexander Gepperth Maître de conférence — ENSTA ParisTech 2 Résumé Des robots mobiles ont déjà fait leur apparition dans nos domiciles pour accom- plir des tâches simples. Pour qu’un robot devienne un véritable assistant, il doit reconnaître les objects qui appartiennent à son propriétaire. Dans cette thèse, des algorithmes de reconnaissance d’instances d’objets sont développés pour faire face aux variations (angles de vue, illumination, etc.) qui surviennent dans un contexte de robotique mobile d’intérieur. Différentes manières de tirer profit de ce contexte sont étudiées. Premièrement, un algorithme de segmentation géometrique est proposé pour profiter de la structure inhérente aux scènes d’intérieur et y détecter des objects isolés. Un réseau de neurones pour la reconnaissance d’objets qui fusionne des informations de forme, couleur et texture provenant d’images de couleur et de profondeur est en- suite présenté. Cet algorithme met en évidence l’importance de combiner plusieurs sources d’information et la difficulté à utiliser la couleur en robotique. Enfin,une solution alternative à base de recherche de plus proche voisin, plus facile à entraîner que le réseau de neurones, est détaillée. Cette approche utilise des mesures comme la distance à l’objet pour éliminer certains paramètres dans la recherche de plus proche voisin et le partitionnement de données. L’information provenant de multiples vues d’un même objet sont aussi fusionnés. L’algorithme résultant fonctionne sur un robot mobile et peut reconnaître 52 ob- jects en 500 ms en moyenne (sur un Intel Core i5 avec 3 GB de RAM) avec un taux de succès de 80%. Les expériences menées dans cette thèse montrent qu’il est essentiel d’identifier et d’utiliser la structure et l’information présentes dans le contexte dela robotique d’intérieur pour faire face aux grandes variations qu’il peut y survenir. Abstract Mobile robots have already entered people’s homes to perform simple tasks for them. For robots at home to become real assistants, they have to be able to rec- ognize the objects in their owner’s home. In this thesis, object instance recognition algorithms are designed to cope with the variations (viewing angle, lighting condi- tions, etc.) that occur in the context of mobile robotics experiments. Several ways to take advantage of this context are studied. First, a geometric segmentation algorithm that benefits from the structure of indoor scenes to find isolated objects isdesigned. Then, a neural network based object recognition process that fuses shape, color and texture information provided by color and depth cameras is presented. This step highlights the importance of carefully combining multiple features and the difficulty to use color information in robotics. Finally, an alternative approach using a nearest neighbor classifier, which is easier to train than the neural network, is detailed. It relies on physical measures available to the robot to eliminate some parameters in clustering and nearest neighbor search procedures. Also, it uses information gathered through multiple sightings of the objects to reduce the negative impact of occlusions. The algorithm can recognize 52 objects with a success rate of 80% and runs in 500 ms on average (on an Intel Core i5 CPU with 3 GB of RAM) on a mobile robot. This work shows that identifying and taking advantage of the structure and information available is essential to handle the variations that happen in indoor mobile robot experiments. 3 4 Remerciments Merci à tous ceux qui m’ont aidé, accompagné, entretenu, supporté, enduré, encour- agé, hébergé et inspiré tout au long de ces 3 ans (et demie (et un peu plus)). Cette thèse a été financée par une bourse d’études supérieures du Conseil de recherches en sciences naturelles et en génie du Canada (CRSNG). This thesis was funded by a Postgraduate Scholarship from the Natural Sciences and Engineering Research Council of Canada (NSERC). 5 6 Contents 1 Introduction 15 1.1 Autonomous mobile robots........................ 15 1.2 Software aspects of a robot at home................... 16 1.3 Computer vision on a mobile robot................... 17 1.4 A scenario for a mobile robot at home................. 21 1.5 Contributions............................... 23 1.6 Structure of the thesis.......................... 26 2 State of the art 27 2.1 Image segmentation............................ 27 2.1.1 Over-segmentation........................ 28 2.1.2 Foreground-background separation............... 30 2.2 Feature-based image comparison..................... 32 2.2.1 From pixels to features...................... 32 2.2.2 Detector: from dense to sparse.................. 34 2.2.3 From an array to a set...................... 35 2.2.4 Vocabularies: from values to representatives.......... 37 2.3 Color in computer vision......................... 39 2.3.1 Models of photometric changes................. 39 2.3.2 Color representations....................... 40 2.3.3 Transformed color representation................ 42 2.3.4 Color moment invariants..................... 43 2.4 Using histograms for density estimation................. 44 2.4.1 Choosing parameters for histograms............... 44 2.4.2 Aliasing in histograms...................... 45 2.4.3 Comparing histograms...................... 46 2.5 Trends in object instance recognition algorithms for mobile robots.. 49 3 Methods 53 3.1 Hardware................................. 53 3.2 Software.................................. 54 3.3 Algorithms................................. 54 3.3.1 Parametric model fitting using RANSAC............ 54 3.3.2 Nearest neighbor search with kd-trees.............. 55 3.3.3 Adaptive occupancy representation using octrees........ 56 7 3.3.4 Computing point normals in a point cloud........... 57 3.3.5 Simultaneous localization and mapping............. 58 3.3.6 Scale invariant feature transform for texture description.... 58 3.3.7 Surflet-pair relation for shape description............ 59 3.3.8 Function approximation with feed-forward neural network.. 61 3.4 Data.................................... 63 3.4.1 Segmentation dataset....................... 63 3.4.2 ENSTA offline object dataset.................. 63 3.4.3 ENSTA online object dataset.................. 66 3.5 Multi-class classification performance measures............. 66 4 Geometrical indoor scene segmentation on a mobile robot 71 4.1 Introduction................................ 71 4.2 Design choices............................... 71 4.3 Implementation details.......................... 73 4.3.1 Offline calibration......................... 73 4.3.2 Pre-processing........................... 73 4.3.3 Floor removal........................... 73 4.3.4 Walls removal........................... 75 4.3.5 Object candidates over-segmentation.............. 77 4.3.6 Object candidates rejection................... 78 4.3.7 Parameters............................ 78 4.3.8 Process overview......................... 78 4.4 Experiments................................ 78 4.4.1 Segmentation performance.................... 78 4.4.2 Run time............................. 79 4.4.3 Use cases............................. 80 4.5 Discussion................................. 85 4.6 Conclusion................................. 85 5 Global object recognition by fusion of shape and texture information with a feed-forward neural network 87 5.1 Introduction................................ 87 5.2 Design choices............................... 87 5.3 Description of the features........................ 89 5.3.1 Histogram of occurrence of SIFT words............. 89 5.3.2 Saturation weighted hue histogram............... 91 5.3.3 Transformed RGB histogram................... 91 5.3.4 Surflet-pair-relation histogram.................. 91 5.4 Data fusion with a feed-forward neural network............ 92 5.4.1
Details
-
File Typepdf
-
Upload Time-
-
Content LanguagesEnglish
-
Upload UserAnonymous/Not logged-in
-
File Pages162 Page
-
File Size-