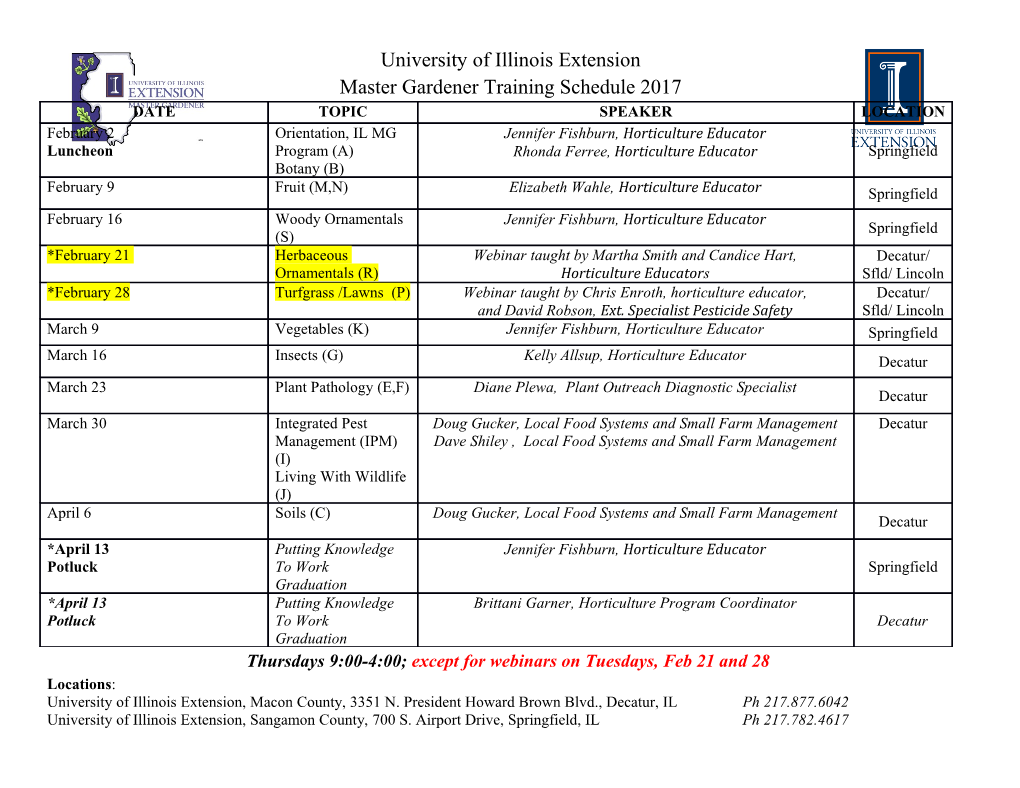
Machine Translation of Arabic Dialects Wael Salloum Submitted in partial fulfillment of the requirements for the degree of Doctor of Philosophy in the Graduate School of Arts and Sciences COLUMBIA UNIVERSITY 2018 © 2018 Wael Salloum All rights reserved ABSTRACT Machine Translation of Arabic Dialects Wael Salloum This thesis discusses different approaches to machine translation (MT) from Dialectal Arabic (DA) to English. These approaches handle the varying stages of Arabic dialects in terms of types of available resources and amounts of training data. The overall theme of this work revolves around building dialectal resources and MT systems or enriching existing ones using the currently available resources (dialectal or standard) in order to quickly and cheaply scale to more dialects without the need to spend years and millions of dollars to create such resources for every dialect. Unlike Modern Standard Arabic (MSA), DA-English parallel corpora is scarcely avail- able for few dialects only. Dialects differ from each other and from MSA in orthography, morphology, phonology, and to some lesser degree syntax. This means that combining all available parallel data, from dialects and MSA, to train DA-to-English statistical machine translation (SMT) systems might not provide the desired results. Similarly, translating di- alectal sentences with an SMT system trained on that dialect only is also challenging due to different factors that affect the sentence word choices against that of the SMT training data. Such factors include the level of dialectness (e.g., code switching to MSA versus dialectal training data), topic (sports versus politics), genre (tweets versus newspaper), script (Ara- bizi versus Arabic), and timespan of test against training. The work we present utilizes any available Arabic resource such as a preprocessing tool or a parallel corpus, whether MSA or DA, to improve DA-to-English translation and expand to more dialects and sub-dialects. The majority of Arabic dialects have no parallel data to English or to any other foreign language. They also have no preprocessing tools such as normalizers, morphological ana- lyzers, or tokenizers. For such dialects, we present an MSA-pivoting approach where DA sentences are translated to MSA first, then the MSA output is translated to English using the wealth of MSA-English parallel data. Since there is virtually no DA-MSA parallel data to train an SMT system, we build a rule-based DA-to-MSA MT system, ELISSA, that uses morpho-syntactic translation rules along with dialect identification and language modeling components. We also present a rule-based approach to quickly and cheaply build a dialectal morphological analyzer, ADAM, which provides ELISSA with dialectal word analyses. Other Arabic dialects have a relatively small-sized DA-English parallel data amount- ing to a few million words on the DA side. Some of these dialects have dialect-dependent preprocessing tools that can be used to prepare the DA data for SMT systems. We present techniques to generate synthetic parallel data from the available DA-English and MSA- English data. We use this synthetic data to build statistical and hybrid versions of ELISSA as well as improve our rule-based ELISSA-based MSA-pivoting approach. We evaluate our best MSA-pivoting MT pipeline against three direct SMT baselines trained on these three parallel corpora: DA-English data only, MSA-English data only, and the combination of DA-English and MSA-English data. Furthermore, we leverage the use of these four MT systems (the three baselines along with our MSA-pivoting system) in two system combi- nation approaches that benefit from their strengths while avoiding their weaknesses. Finally, we propose an approach to model dialects from monolingual data and limited DA-English parallel data without the need for any language-dependent preprocessing tools. We learn DA preprocessing rules using word embedding and expectation maximization. We test this approach by building a morphological segmentation system and we evaluate its performance on MT against the state-of-the-art dialectal tokenization tool. Contents List of Figures vii List of Tables xi 1 Introduction 1 1.1 Introduction to Machine Translation . 2 1.2 Introduction to Arabic and its Challenges for NLP . 4 1.2.1 Arabic as a Prototypical Diglossic Language . 4 1.2.2 Modern Standard Arabic Challenges . 5 1.2.3 Dialectal Arabic Challenges . 5 1.2.4 Dialectness, Domain, Genre, and Timespan . 7 1.2.5 Overview and Challenges of Dialect-Foreign Parallel Data . 7 1.3 Contributions . 8 1.3.1 Thesis Contributions . 9 1.3.2 Released Tools . 19 1.3.3 Note on Data Sets . 19 1.4 Thesis Outline . 20 2 Related Work 21 2.1 Introduction to Machine Translation . 21 2.1.1 Rule-Based versus Statistical Machine Translation . 21 i 2.1.2 Neural Machine Translation (NMT) . 22 2.2 Introduction to Arabic and its Challenges for NLP . 23 2.2.1 A History Review of Arabic and its Dialects . 23 2.2.2 Arabic as a Prototypical Diglossic Language . 26 2.2.3 Modern Standard Arabic Challenges . 26 2.2.4 Dialectal Arabic Challenges . 28 2.2.5 Dialectness, Domain, Genre, and Timespan . 29 2.2.6 Overview and Challenges of Dialect-Foreign Parallel Data . 30 2.3 Dialectal Arabic Natural Language Processing . 31 2.3.1 Extending Modern Standard Arabic Resources . 31 2.3.2 Dialectal Arabic Morphological Analysis . 32 2.3.3 Dialect Identification . 34 2.4 Machine Translation of Dialects . 34 2.4.1 Machine Translation for Closely Related Languages. 35 2.4.2 DA-to-English Machine Translation . 35 2.5 Machine Translation System Combination . 37 2.6 Morphological Segmentation . 38 2.6.1 Supervised Learning Approaches to Morphological Segmentation . 38 2.6.2 Unsupervised Learning Approaches to Morphological Segmentation 38 I Translating Dialects with No Dialectal Resources 41 3 Analyzer for Dialectal Arabic Morphology (ADAM) 43 3.1 Introduction . 43 3.2 Motivation . 44 3.3 Approach . 45 3.3.1 Databases . 45 ii 3.3.2 SADA Rules . 46 3.4 Intrinsic Evaluation . 49 3.4.1 Evaluation of Coverage . 50 3.4.2 Evaluation of In-context Part-of-Speech Recall . 51 3.5 Extrinsic Evaluation . 52 3.5.1 Experimental Setup . 53 3.5.2 The Dev and Test Sets . 53 3.5.3 Machine Translation Results . 54 3.6 Conclusion and Future Work . 55 4 Pivoting with Rule-Based DA-to-MSA Machine Translation System (ELISSA) 59 4.1 Introduction . 59 4.2 Motivation . 60 4.3 The ELISSA Approach . 62 4.4 Selection . 64 4.4.1 Word-based selection . 64 4.4.2 Phrase-based selection . 65 4.5 Translation . 66 4.5.1 Word-based translation . 66 4.5.2 Phrase-based translation . 68 4.6 Language Modeling . 70 4.7 Intrinsic Evaluation: DA-to-MSA Translation Quality . 71 4.7.1 Revisiting our Motivating Example . 71 4.7.2 Manual Error Analysis . 72 4.8 Extrinsic Evaluation: DA-English MT . 73 4.8.1 The MSA-Pivoting Approach . 73 4.8.2 Experimental Setup . 74 iii 4.8.3 Machine Translation Results . 76 4.8.4 A Case Study . 78 4.9 Conclusion and Future Work . 79 II Translating Dialects with Dialectal Resources 81 5 Pivoting with Statistical and Hybrid DA-to-MSA Machine Translation 83 5.1 Introduction . 83 5.2 Dialectal Data and Preprocessing Tools . 84 5.3 Synthesizing Parallel Corpora . 84 5.4 The MSA-Pivoting Approach . 85 5.4.1 Improving MSA-Pivoting with Rule-Based ELISSA . 88 5.4.2 MSA-Pivoting with Statistical DA-to-MSA MT . 89 5.4.3 MSA-Pivoting with Hybrid DA-to-MSA MT . 89 5.5 Evaluation . 90 5.5.1 Experimental Setup . 90 5.5.2 Experiments . 91 5.6 Conclusion and Future Work . 93 6 System Combination 95 6.1 Introduction . 95 6.2 Related Work . 96 6.3 Baseline Experiments and Motivation . 96 6.3.1 Experimental Settings . 97 6.3.2 Baseline MT Systems . 99 6.3.3 Oracle System Combination . 100 6.4 Machine Translation System Combination . 100 iv 6.4.1 Dialect ID Binary Classification . 102 6.4.2 Feature-based Four-Class Classification . 102 6.4.3 System Combination Evaluation . 104 6.5 Discussion of Dev Set Subsets . 106 6.5.1 DA versus MSA Performance . 107 6.5.2 Analysis of Different Dialects . 108 6.6 Error Analysis . 110 6.6.1 Manual Error Analysis . 110 6.6.2 Example . 111 6.7 Conclusion and Future Work . 111 III Scaling to More Dialects 113 7 Unsupervised Morphological Segmentation for Machine Translation 115 7.1 Introduction . 115 7.2 Related Work . 116 7.2.1 Supervised Learning Approaches to Morphological Segmentation . 117 7.2.2 Unsupervised Learning Approaches to Morphological Segmentation 117 7.3 Approach . 118 7.4 Monolingual Identification of Segmentation Rules . 122 7.4.1 Clustering based on Word Embeddings . 122 7.4.2 Rule Extraction and Expansion . 123 7.4.3 Learning Rule Scores . 127 7.4.4 Experiments . 129 7.5 Alignment Guided Segmentation Choice . 130 7.5.1 Approach . 130 7.5.2 The Alignment Model . ..
Details
-
File Typepdf
-
Upload Time-
-
Content LanguagesEnglish
-
Upload UserAnonymous/Not logged-in
-
File Pages201 Page
-
File Size-