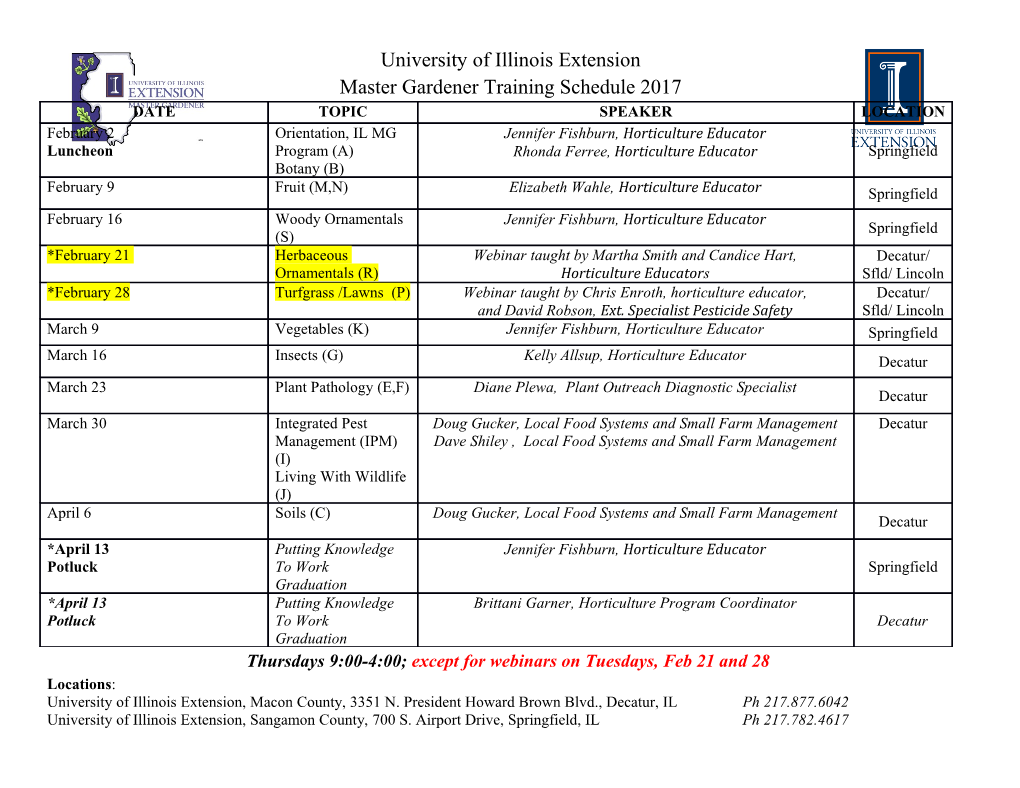
What is naturalness? the fairly biased view of a particle physicist based on P Athron, C Balázs, B Farmer, A Fowlie, D Harries, D Kim JHEP 1710 (2017) 160 A Fowlie, C Balázs, G White, L Marzola, M Raidal JHEP 1608 (2016) 100 D Kim, P Athron, C Balázs, B Farmer, E Hutchison PRD90 (2014) 5 055008 C Balázs, A Buckley, D Carter, B Farmer, M White EPJC73 (2013) 2563 special thanks to Alberto Casas, Jesus Moreno, Bryan Zaldívar C Balázs | 2019 Jun 25 Madrid | page 1 of 40 Outline What is naturalness? How to quantify fine tuning? What is the meaning of a fine tuning measure? C Balázs | 2019 Jun 25 Madrid | page 2 of 40 “Everything is natural: if it weren’t, it wouldn’t be.” Mary Catherine Bateson . M.C. Bateson On the Naturalness of Things “How Things Are: A Science Toolkit for the Mind” C Balázs | 2019 Jun 25 Madrid | page 3 of 40 ed. J. Brockman and K. Matson “Everything is natural: if it weren’t, it wouldn’t be.” Mary Catherine Bateson . Consequently, if a phenomenon appears unnatural then there’s a problem with our understanding of the phenomenon or naturalness. M.C. Bateson On the Naturalness of Things “How Things Are: A Science Toolkit for the Mind” C Balázs | 2019 Jun 25 Madrid | page 4 of 40 ed. J. Brockman and K. Matson Tenish years ago my notion of naturalness was… Naturalness is a theoretical prejudice. Naturalness is a subjective principle. (Compared to e.g. the gauge principle.) Naturalness is qualitative and unquantifiable. Consequently, naturalness shouldn’t be used in physics. C Balázs | 2019 Jun 25 Madrid | page 5 of 40 In particular, I didn’t understand the Barbieri-Ellis-Giudice measure: 휕(푒푙푒푐푡푟표푤푒푎푘 표푏푠푒푟푣푎푏푙푒) 휕(푡ℎ푒표푟푦 푝푎푟푎푚푒푡푒푟) * For the observable people use 푚푍, 푚ℎ, 푣, etc. Why? * For the parameter people use 휇, 푀0, 푀1/2, etc. Why? 휕푚 휕푚2 휕log(푚 ) * The form changes: 푍, 푍, 푍 , etc. Why? 휕휇 휕휇2 휕log(휇) * For more parameters: Max? Quadrature? Another combination? Why? 휕푚 * What does 푍 have to do with the other fine tuning measures? 휕휇 * Beyond MSSM, NMSSM, SUSY and EW fine tuning? * How much fine tuning is too much? C Balázs | 2019 Jun 25 Madrid | page 6 of 40 Since then, I learned a few things... C Balázs | 2019 Jun 25 Madrid | page 7 of 40 Physics is naturally divided across length scales universe 10+30푚 cosmology galaxy 10+20푚 astrophysics star 10+10푚 solar physics rock 100 푚 solid state physics atom 10−10푚 atomic physics top quark 10−20푚 particle physics Planck cell 10−30푚 quantum gravity C Balázs | 2019 Jun 25 Madrid | page 8 of 40 Naturalness Physical phenomena characterized by disparate (energy or length) scales are separated: Their governing laws can be understood largely independently from each other. They obey the naturalness principle. Natural sciences exists as we know them because of naturalness. C Balázs | 2019 Jun 25 Madrid | page 9 of 40 Example 1 The orbit of the moon is not affected by sub-nuclear physics. Newton’s gravity is natural. C Balázs | 2019 Jun 25 Madrid | page 10 of 40 Example 2 A doctor may cure a patient without being an expert in cosmology. Medical science (we may say) is natural. C Balázs | 2019 Jun 25 Madrid | page 11 of 40 Example 3 In the effective description of the Standard Model of particles the Higgs boson mass acquires a dependence on the Planck scale. The Higgs and Planck masses are many orders of magnitudes apart. The Standard Model violates naturalness. C Balázs | 2019 Jun 25 Madrid | page 12 of 40 Example 3 In the effective description of the Standard Model the Higgs boson mass is given by 2 2 2 푚ℎ = −휇 + Λ If Λ is the Planck scale then Λ2 and 휇2 have to be very finely tuned to obtain the observed Higgs mass. Naturalness can be quantified in terms of fine tuning. But how can we quantify fine tuning? C Balázs | 2019 Jun 25 Madrid | page 13 of 40 Many different means exists for quantifying fine tuning. I only concentrate on one of them. I’m convinced that many of them are essentially equivalent. C Balázs | 2019 Jun 25 Madrid | page 14 of 40 Consider model 1 quantified by a single parameter 푝. Assume that this theory predicts a measured observable 표. ℒ(표, 푝) is the likelihood of the model predicting the observable. C Balázs | 2019 Jun 25 Madrid | page 15 of 40 Consider model 1 quantified by a single parameter 푝. Assume that this theory predicts a measured observable 표. ℒ(표, 푝) is the likelihood of the model predicting the observable. C Balázs | 2019 Jun 25 Madrid | page 16 of 40 Consider model 2 quantified by the same parameter 푝. Assume that this theory also predicts the observable 표. ℒ(표, 푝) is the likelihood of the model predicting the observable. C Balázs | 2019 Jun 25 Madrid | page 17 of 40 Which model looks less fine-tuned? If you said ‘the first one’, why? C Balázs | 2019 Jun 25 Madrid | page 18 of 40 Why does the first model look less fine tuned? Particle physicist’s answer: because ℒ(표, 푝) varies milder with 푝. But here I’ll go with the astrophysicist’s answer. C Balázs | 2019 Jun 25 Madrid | page 19 of 40 Why does the first model look less fine tuned? Because it has a higher evidence! ( Bayesians: assume flat prior, or plot the posterior :) What does the evidence have to do with fine tuning? C Balázs | 2019 Jun 25 Madrid | page 20 of 40 Bayesian evidence: ℰ = න ℒ 표, 푝 휋 푝 푑푝 prior info on 푝 before data 푑 data 푑 updating prior info 휋 . C Balázs | 2019 Jun 25 Madrid | page 21 of 40 Bayesian evidence: ℰ = න ℒ 표, 푝 휋 푝 푑푝 probability distribution of 푝 before observation 표 C Balázs | 2019 Jun 25 Madrid | page 22 of 40 Bayesian evidence: ℰ = න ℒ 표, 푝 휋 푝 푑푝 observation 표 updating prior info 휋 . C Balázs | 2019 Jun 25 Madrid | page 23 of 40 the Bayesian evidence is … ℰ = න ℒ 표, 푝 휋 푝 푑푝 … the plausibility that a hypothesis reproduces an observation . … proportional to global fine tuning (will see this quantitatively in a moment) C Balázs | 2019 Jun 25 Madrid | page 24 of 40 deriving a local fine tuning measure assume that 표 푝1, 푝2 is precisely measured: 표푒푥푝 then one model parameter, say 푝1, can be fixed: 휕푝 ℰ = න 훿 표 푝 , 푝 − 표 휋 푝 , 푝 1 푑표 푑푝 1 2 푒푥푝 1 2 휕표 2 C Balázs | 2019 Jun 25 Madrid | page 25 of 40 deriving a local fine tuning measure assume that 표 푝1, 푝2 is precisely measured: 표푒푥푝 then one model parameter, say 푝1, can be fixed: 휕푝 ℰ = න 훿 표 푝 , 푝 − 표 휋 푝 , 푝 1 푑표 푑푝 1 2 푒푥푝 1 2 휕표 2 C Balázs | 2019 Jun 25 Madrid | page 26 of 40 deriving a local fine tuning measure assume that 표 푝1, 푝2 is precisely measured: 표푒푥푝 then one model parameter, say 푝1, can be fixed: 휕푝 ℰ = න 훿 표 푝 , 푝 − 표 휋 푝 , 푝 1 푑표 푑푝 1 2 푒푥푝 1 2 휕표 2 휕푝 ℰ = න 휋 푝 , 푝 1ቤ 푑푝 1,푒푥푝 2 휕표 2 표푒푥푝 휕푝 the prior of 푝 got modified by 1 2 휕표 hence the name naturalness prior C Balázs | 2019 Jun 25 Madrid | page 27 of 40 deriving a local fine tuning measure assume that 표 푝1, 푝2 is precisely measured: 표푒푥푝 then one model parameter, say 푝1, can be fixed: 휕푝 ℰ = න 훿 표 푝 , 푝 − 표 휋 푝 , 푝 1 푑표 푑푝 1 2 푒푥푝 1 2 휕표 2 휕푝 ℰ = න 휋 푝 , 푝 1ቤ 푑푝 1,푒푥푝 2 휕표 2 표푒푥푝 휕푝 1 is the inverse of Barbieri-Ellis-Giudice fine tuning measure ! 휕표 C Balázs | 2019 Jun 25 Madrid | page 28 of 40 deriving a local fine tuning measure assume that 표 푝1, 푝2 is precisely measured: 표푒푥푝 then one model parameter, say 푝1, can be fixed: 휕푝 ℰ = න 훿 표 푝 , 푝 − 표 휋 푝 , 푝 1 푑표 푑푝 1 2 푒푥푝 1 2 휕표 2 휕푝 ℰ = න 휋 푝 , 푝 1ቤ 푑푝 1,푒푥푝 2 휕표 2 표푒푥푝 휕푝 1 measures fine tuning at every value of 푝 휕표 2 휕푝 (표 depends on 푝 , and so does 1) 2 휕표 C Balázs | 2019 Jun 25 Madrid | page 29 of 40 properties of the fine tuning measure in the context of the MSSM: 푝1 = 휇, 표 = 푚푍, 푝2 = rest of para. 휕휇 ℰ = න 휋 휇푒푥푝, 푝2 อ 푑푝2 휕푚푍 푚푍,푒푥푝 휕휇 * when the fine tuning is large, is small, ℰ is suppressed, so 휕푚푍 휕휇 measures the local fine tuning, and 휕푚푍 ℰ measures the global fine tuning C Balázs | 2019 Jun 25 Madrid | page 30 of 40 properties of the fine tuning measure in the context of the MSSM: 푝1 = 휇, 표 = 푚푍, 푝2 = rest of para. 휕휇 ℰ = න 휋 휇푒푥푝, 푝2 อ 푑푝2 휕푚푍 푚푍,푒푥푝 휕휇 * measures fine tuning because 휇 was traded for 푚푍 휕푚푍 휕푚 휕푚 consequently, 푍 , 푍 , etc. are not qunatifying fine tuning 휕푀0 휕푀1/2 휕휇 * depends on 푀0, 푀1/2, … so it measures local fine tuning 휕푚푍 C Balázs | 2019 Jun 25 Madrid | page 31 of 40 Constrained Minimal Supersymmetric Model the set of parameters 푝 = {휇, 푦푡, 퐵0} are traded for observables 표 = {푚푍, 푚푡, 푡푎푛훽} invoking the determinant 휕푚푍 휕푚푡 휕푡푎푛훽 휕휇 휕휇 휕휇 휕표 휕푚 휕푚 휕푡푎푛훽 = 푍 푡 휕푝 휕푦푡 휕푦푡 휕푦푡 휕푚푍 휕푚푡 휕푡푎푛훽 휕퐵0 휕퐵0 휕퐵0 C Balázs | 2019 Jun 25 Madrid | page 32 of 40 What did I learn about the Barbieri-Ellis-Giudice measure? 휕(푒푙푒푐푡푟표푤푒푎푘 표푏푠푒푟푣푎푏푙푒) 휕(푡ℎ푒표푟푦 푝푎푟푎푚푒푡푒푟) 휕표 * Observable: 푚 , 푚 , 푣, etc.
Details
-
File Typepdf
-
Upload Time-
-
Content LanguagesEnglish
-
Upload UserAnonymous/Not logged-in
-
File Pages40 Page
-
File Size-