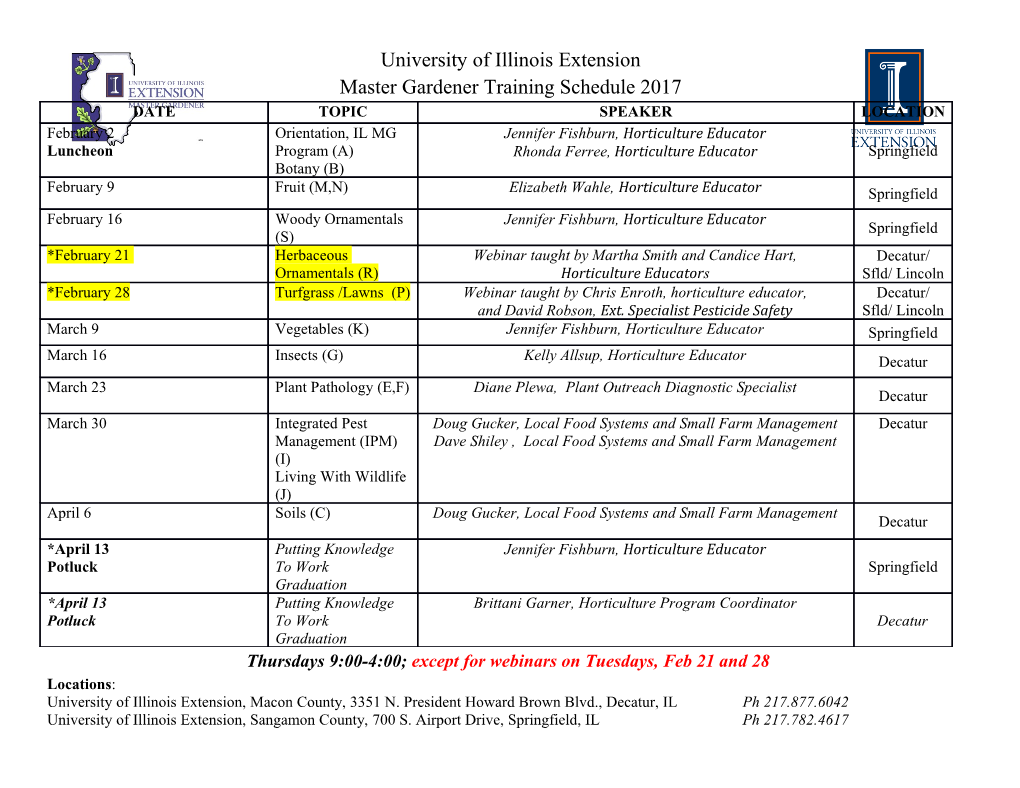
An analysis of pulsation periods of long-period variable stars Jeffrey D. Hart Collabor Department of Statistics, Texas A&M University ators An analysis of pulsation periods of long-period variable stars – p.1 Collaborators Chris Koen – South African Astronomical Observatory, Cape, South Africa Fred Lombard – Rand Afrikaans University, Auckland Park, South Africa An analysis of pulsation periods of long-period variable stars – p.2 Outline • Brief introduction to variable stars • Problem of interest • Statistical model • Investigating assumptions • Characteristics of fitted models • Testing for trend • Conclusions An analysis of pulsation periods of long-period variable stars – p.3 Variable stars • Characterized by brightness changes over time • Long period variables – distinguished by substantial brightness changes • Changes are roughly sinusoidal with typical periods between 100 and 300 days. • The period of a given star is determined by its internal structure. • Period changes important as they reflect changing physical conditions in the stars. An analysis of pulsation periods of long-period variable stars – p.4 Data • Database of 378 long-period variables • Times of maximum brightness recorded by amateur and professional astronomers. • Data processed by American Association of Variable Star Observers (AAVSO). AAVSO website: http://www.aavso.org • For each star in the database, we consider P1; : : : ; Pn, the observed times between successive maxium brightnesses. • n ranges from 32 to 212 with median 74. An analysis of pulsation periods of long-period variable stars – p.5 Light curve and periods 10 8 6 brightness 4 2 0 200 400 600 800 days An analysis of pulsation periods of long-period variable stars – p.6 Typical data plots Omicron Ceti R Aquilae • • • • 350 • • • • • • • • • • • • • • • • • • • • • • • • •• 320 • • •• • 340 • • • • • • • • • • • • • ••• • • • • • • ••• • • •• • • • • • •• • • • • • • • •• •• • • • •• • • • 330 • • • • • •• •• •• period • • period • • • • • • • • 300 • • • • •• • • • • • • • •• • • • • • •••• • • • • • • • • • 320 • • • •• • • • • • • •• • • 280 • • • 310 0.0 0.2 0.4 0.6 0.8 1.0 0.0 0.2 0.4 0.6 0.8 1.0 standardized epoch standardized epoch R Bootis R Camelo • • • • • • • 290 • 235 • • • • • • • • •• • • • • • • • • • • • • • • 230 • • • • •• •• • • 280 ••• • • • • • •• • • • • • • •• ••• • • ••••• • •• •• • • • • • •• • •• • • • • • • • ••• • • 225 • •• • • •• •• • • • • • • • • •• • • • • • • 270 • • • • • • • •• • •••• •• • • • •• • • • • • period • • • •••• • • • • period •• • • •• • • • 220 • • •• • •• • • • • • • • • • • 260 • • • • • • • • • 215 • • • • • • • • • • • • • 250 • • 210 0.0 0.2 0.4 0.6 0.8 1.0 0.0 0.2 0.4 0.6 0.8 1.0 standardized epoch standardized epoch An analysis of pulsation periods of long-period variable stars – p.7 Problem of interest • For each star in the database, determine if there is evidence of a trend in the times between consecutive maximum brightnesses. • Formally, we wish to test the null hypothesis H0 : E(P1) = E(P2) = · · · = E(Pn): Will conduct a test of this hypothesis for each of the 378 stars in the database. • Allow for performing multiple tests: Benjamini and Hochberg (1995). An analysis of pulsation periods of long-period variable stars – p.8 Variety of methods and challenges 1. Time series and spectral analysis 2. Data-driven model selection 3. Smoothing 4. Wild failure of asymptotic distribution theory for a likelihood ratio statistic 5. Bootstrap in an unconventional way to deal with 4 and computational issues. An analysis of pulsation periods of long-period variable stars – p.9 Model • P1; : : : ; Pn: observed lengths of time between successive maximum brightnesses for a given star • Model used by astronomers: Pj = P + Tj + Ij + j − j−1; j = 1; : : : ; n ◦ P : long-run average of all Pjs ◦ T1; : : : ; Tn: constants representing trend ◦ I1; : : : ; In: “intrinsic” errors, i.e., random deviations intrinsic to the star ◦ 1; : : : ; n: errors made in determining times of maximum brightness An analysis of pulsation periods of long-period variable stars – p.10 Hypothesis of interest In terms of our statistical model, the hypothesis of interest is H0 : T1 = T2 = · · · = Tn = 0: We wish to test this hypothesis for each star. An analysis of pulsation periods of long-period variable stars – p.11 Error series Usual assumptions in astronomy literature: • 2 I1; : : : ; In are i.i.d. (0; σI ). • 2 0; : : : ; n are i.i.d. (0; σ ). • The two series are independent. An analysis of pulsation periods of long-period variable stars – p.12 Error series Let ξj = Ij + j − j−1, j = 1; : : : ; n. • fξjg has same covariance function as first order moving average process. • First lag correlation of fξjg is always between −1=2 and 0. • Spectral density of fξjg is monotone increasing, or “high frequency.” An analysis of pulsation periods of long-period variable stars – p.13 De-trending of data Each data set is de-trended as follows: • Fit a fifth degree polynomial to ((j − 1=2)=n; Pj), j = 1; : : : ; n. • Compute residuals ej = Pj − P^j; j = 1; : : : ; n: These residuals will be analyzed in various ways to discover properties of the error process. An analysis of pulsation periods of long-period variable stars – p.14 Estimated log-spectra for five stars 5 4 log(spectrum) 3 2 0.0 0.1 0.2 0.3 0.4 0.5 frequency These are the stars whose estimated spectra at 0 frequency were at the 10th, 25th, 50th, 75th, and 90th percentiles among all 378 stars. An analysis of pulsation periods of long-period variable stars – p.15 Investigating assumptions To perform a valid test of H0, the error series Ij + j − j−1; j = 1; : : : ; n; needs to be modeled correctly. Questions to consider: • Is the error series homoscedastic? • Is it reasonable to assume that the two series fIjg and fjg are Gaussian? An analysis of pulsation periods of long-period variable stars – p.16 Homoscedasticity Absolute residuals for six variable stars and local linear smooths · · · 3 · 2.5 · · · · 2.0 · · 2 · · · · · · · · · · 1.5 · · · · · · |residual| |residual| · · · · · · · · · 1.0 · · 1 · · · · · · · · · · · · · · · · · · · · · · · · · · · · · · · · · · · · 0.5 · · · · · · · · · · ·· · · · · · · · · · · · · · · · · · · ·· · · · · · · · · · · · · · · · · · ·· · 0 · · · · · · · · · · · · · 0.0 0.0 0.2 0.4 0.6 0.8 1.0 0.0 0.2 0.4 0.6 0.8 1.0 epoch epoch · · 2.5 4 · · 2.0 · · · · · · 3 · ·· 1.5 · · · · · · · · · · · · 2 · · · · · · · |residual| · · · ·· |residual| · ·· 1.0 · · · · · · · · · ·· · · · · · · · · · · · · · ·· · · · · · · · · · · · · 1 · ·· · · · · · · · · · · · · ·· · · · ·· · · · · · · 0.5 · · · · · · · · · · · ·· · · · · · ·· ·· · · · · · · · · · · · · · · · · · · · · · ·· · · · ···· ·· · · · ·· · · ·· · · · · · · · · · · · · · · ·· · · · · · · 0 · · · · · · · 0.0 0.0 0.2 0.4 0.6 0.8 1.0 0.0 0.2 0.4 0.6 0.8 1.0 epoch epoch 2.5 · · · · 2.5 · · · 2.0 · · · ·· 2.0 · · · · · · · · · 1.5 · · · · · · · · · · · 1.5 · · · · · · · · · · · · · · ·· · 1.0 |residual| · · · · · · |residual| · · · 1.0 · · · · · · · · · · · · · · · · · · · · · · · · · · · · · · · · · · 0.5 · · · ··· · · · ·· · · · ·· 0.5 · · ·· · · · · · · · · · · · · · · · · ·· · · · · · · · · · · · · · · · ·· · · · · · · ·· · · · · 0.0 0.0 0.0 0.2 0.4 0.6 0.8 1.0 0.0 0.2 0.4 0.6 0.8 1.0 epoch epoch An analysis of pulsation periods of long-period variable stars – p.17 Model for heteroscedasticity • We assume heteroscedasticity arises only from increasing precision in determining times of maximum brightness. • We thus assume intrinsic errors are i.i.d. • Will assume measurement errors have the form j − 1=2 = exp (β =2) + (β =2) η ; j = 1; : : : ; n; j 0 1 n j where β0 and β1 are unknown constants and η1; : : : ; ηn are independent and identically distributed with mean 0 and variance 1. An analysis of pulsation periods of long-period variable stars – p.18 Normality • Is it reasonable to assume that the errors are normally distributed? • Standardized residuals: ei Ei = ; i = 1; : : : ; n; σ^i where e1; : : : ; en are the residuals from a fitted fifth degree 2 polynomial and σ^i , i = 1; : : : ; n, is a local linear smooth of 2 2 e1; : : : ; en. • The standardized residuals for all 378 stars were pooled together to investigate whether there are departures from normality. An analysis of pulsation periods of long-period variable stars – p.19 Distribution of pooled residuals Let ij be i.i.d. fi for j = 1; : : : ; m, i = 1; : : : ; n. Kernel estimate of pooled data: n m 1 x − f^ (x) = K ij h nmh h Xi=1 Xj=1 n 1 = f^ (x; i): n h Xi=1 An analysis of pulsation periods of long-period variable stars – p.20 Distribution of pooled residuals Suppose that for a fraction w of the n data sets, fi ≡ φ, the standard normal density. Then f^h(x) estimates wφ(x) + (1 − w)gn(x); where gn is a mixture of nonnormal densities. Nonnormality should be detectable from f^h if • The fraction 1 − w of nonormal densities is fairly substantial, and • the density gn is substantially nonnormal. Presumably, the latter would happen if the departures from normality tended to be similar to each other. An analysis of pulsation periods of long-period variable stars – p.21 Kernel density estimate of pooled residuals 0.4 0.3 0.2 density 0.1 0.0 −4 −2 0 2 4 residuals —– Kernel estimate —– Standard normal An analysis of pulsation periods of long-period variable stars – p.22 Final model 1. Trend modeled as a polynomial of degree m, which is unknown but not larger than 15. 2. The two series fIjg and fjg are independent of each other. 3. Intrinsic errors fIjg follow an AR(1) process such
Details
-
File Typepdf
-
Upload Time-
-
Content LanguagesEnglish
-
Upload UserAnonymous/Not logged-in
-
File Pages42 Page
-
File Size-