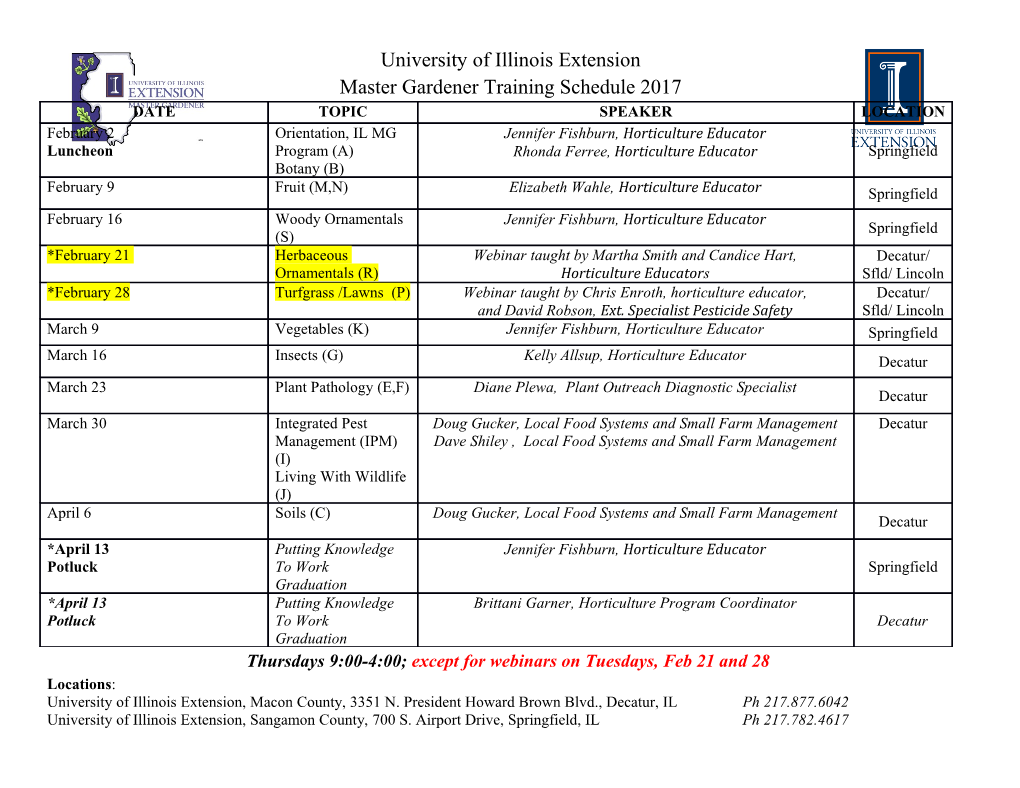
Science Meets Sports Science Meets Sports: When Statistics Are More Than Numbers Edited by Christophe Ley and Yves Dominicy Science Meets Sports: When Statistics Are More Than Numbers Edited by Christophe Ley and Yves Dominicy This book first published 2020 Cambridge Scholars Publishing Lady Stephenson Library, Newcastle upon Tyne, NE6 2PA, UK British Library Cataloguing in Publication Data A catalogue record for this book is available from the British Library Copyright © 2020 by Christophe Ley, Yves Dominicy and contributors All rights for this book reserved. No part of this book may be reproduced, stored in a retrieval system, or transmitted, in any form or by any means, electronic, mechanical, photocopying, recording or otherwise, without the prior permission of the copyright owner. ISBN (10): 1-5275-5856-8 ISBN (13): 978-1-5275-5856-4 We dedicate this book to our families and to the memory of Jacques Dominicy. Christophe Ley especially dedicates this book to his wife Nadine. Contents Preface xiii 1 The Home Run Explosion 1 1.1 Introduction . 2 1.1.1 Game of Baseball . 2 1.1.2 A Plate Appearance . 3 1.1.3 MLB and the Home Run Committee Report . 4 1.1.4 Statcast Data . 6 1.1.5 Plan of the Chapter . 7 1.2 Empirical Perspective . 7 1.2.1 Launch Conditions: The RED Zone . 7 1.2.2 Changes in Rates of Batted Balls in the RED Region . 8 1.2.3 Changes in Home Run Rates for Batted Balls in the RED Region . 10 1.3 Modelling Perspective . 11 1.3.1 Introduction . 11 1.3.2 Generalised Additive Model . 11 1.3.3 Estimating Home Run Probabilities . 12 1.3.4 Predicting Home Run Counts . 13 1.4 Conclusions . 14 2 Advances in Basketball Statistics 19 2.1 Introduction . 20 2.2 Basketball Statistics . 21 2.3 Statistical Analyses . 23 2.3.1 Basic Statistical Analyses . 24 2.3.2 Advanced Statistical Analyses . 25 viii Contents 2.4 Statistics Answers Fans' Questions . 28 2.5 Conclusions . 43 3 Measurement 53 3.1 Introduction|Words and Numbers . 54 3.2 Measurement Best Practices . 54 3.3 Individual Contribution to Team . 58 3.4 Levels of Measurement Redux . 59 3.5 Rating Teams with Disparate Schedules . 61 3.6 Entropy Describes Repertoire . 65 3.7 The Embeddings Approach . 66 3.8 Validity|Words that Matter . 72 3.9 Conclusions . 75 4 Analysing Positional Data 81 4.1 Introduction . 82 4.2 Related Work . 82 4.3 Player Movement . 83 4.3.1 Positions . 83 4.3.2 Velocities and Accelerations . 83 4.3.3 Movement Models . 85 4.4 Zones of Control . 87 4.5 Intelligent Retrieval . 91 4.6 Conclusions . 92 5 Ranking and Prediction Models 95 5.1 Introduction . 96 5.2 Modelling of Football Matches . 97 5.2.1 Poisson Models . 97 5.2.2 Ordinal Models . 99 5.3 Ranking Methods . 100 Contents ix 5.3.1 Point-Winning Systems . 100 5.3.2 Least Squares Models . 101 5.3.3 Maximum Likelihood Methods . 101 5.3.4 Elo Models . 103 5.3.5 Examples . 104 5.4 Prediction Models . 107 5.4.1 Poisson Regression . 107 5.4.2 Ordinal Regression . 110 5.4.3 Prediction Measures . 111 5.4.4 Application to the German Bundesliga . 112 5.4.5 Extensions . 115 5.5 Conclusion . 117 6 Running Shoes and Running Injuries 123 6.1 Introduction . 124 6.2 Part I . 125 6.2.1 It is all in the Study Design . 125 6.2.2 Time to Event Analyses are the Current Gold Standard . 128 6.2.3 Running Shoes do not Cause Running Injuries 130 6.2.4 Accepting Impermanence: Time-Varying Expo- sures, Effect Modifiers and Outcomes . 131 6.3 Part II: The Front-Row View . 133 6.3.1 Strong Beliefs and Competition . 133 6.3.2 Running Shoe Prescription does not Work . 133 6.3.3 Is Cushioning Important? . 135 6.3.4 Foot Morphology, Injury Risk and Shoe Types 136 6.3.5 Other Shoe Features . 137 6.3.6 Throw Your Shoes Away? . 137 6.4 Common Sense Must Prevail . 139 x Contents 7 Markov Chain Modelling 147 7.1 Introduction . 148 7.2 Markov Chain Models . 149 7.2.1 Markov Chains . 149 7.2.2 The Markov Property . 149 7.2.3 Finite Markov Chains . 150 7.3 Net Games as Finite Markov Chains . 153 7.3.1 State-Transition Modelling of Net Games . 153 7.3.2 Performance Analysis Using Markov Chains . 156 7.3.3 Model Validation . 157 7.4 Game Simulations . 159 7.4.1 Simulations Using Changes in Transition Prob- abilities . 159 7.4.2 Simulations Using Numerical Differentiation . 161 7.5 Discussion and Outlook . 165 7.5.1 Sports Applications . 165 7.5.2 Methodological Outlook . 166 8 The Inner Game in Tennis 171 8.1 Introduction . 172 8.2 Experimental Studies . 173 8.3 Qualitative Studies . 174 8.4 Quantitative Studies . 175 8.4.1 Emerging Tools of Quantitative Analysis . 177 8.4.2 Identifying Mentality Profiles . 178 8.4.3 Emotion Measurement with Computer Vision . 180 8.5 Summary . 181 9 Tennis Betting Odds 189 9.1 Introduction . 190 9.2 Literature Review . 191 Contents xi 9.3 Betting Terminology . 193 9.4 Testing the Informational Content . 198 9.4.1 Data . 198 9.4.2 Binned Data . 198 9.4.3 Individual Match Data . 201 9.5 Practical Implications . 206 9.6 Conclusion . 208 10 Sports Timetabling 213 10.1 Introduction . 214 10.2 Preliminaries . 215 10.2.1 Sports Timetabling . 215 10.2.2 Fairness Criteria . 215 10.2.3 Fairness Trade-Offs . 219 10.3 For Academics . 220 10.3.1 Trade-Offs Between Two Fairness Criteria . 220 10.3.2 Trade-Offs Between Efficiency and Equity . 225 10.4 For Practitioners . 233 10.4.1 Trade-Offs Between Two Fairness Criteria . 233 10.4.2 Trade-Offs Between Efficiency and Equity . 236 10.5 Conclusion . 241 Preface Aim of the Book The objective of this book is to present the field of sports statis- tics to two very distinct target audiences. On the one hand the aca- demicians, mainly statisticians, in order to raise their interest in this growing field, and on the other hand sports fans who, even without advanced mathematical knowledge, will be able to understand the data analysis part and gain new insights into their favourite sports. The book thus offers a unique perspective on this attractive topic by combining sports analytics, data visualisation and advanced statistical procedures to extract new findings from sports data such as improved rankings or prediction methods. Football, tennis, basketball, track and field, baseball{every sport aficionado should find his/her interest in this book. Thanks to cutting- edge data analysis tools, the present book will provide the reader with completely new insights into his/her favourite sport and this in an engaging and user-friendly way. Context of the Present Book The world of sports is currently undergoing a fundamental change thanks to the upcoming trend of sports analytics. Recent advances in data collection techniques have enabled the collection of large, some- times even massive, amounts of data in all aspects of sports, such as tactics, technique, health complaints and injuries, spatio-temporal whereabouts (e.g., tracking data from GPS), but also marketing and betting. Data are by now regularly collected in almost every sport, ranging from traditional Olympic disciplines to professional football, basketball, and handball, to name but a few. Moreover, massive data from individual recreational athletes, such as runners or cyclists, is available. It is by far not only professional and commercially success- ful sports clubs that aim to analyse data, even recreational athletes and amateur clubs make use of a variety of sensors to monitor their training and performances. This global rush towards using advanced statistics and machine learning (or, in modern terms, Data Science) methods in sports is due, xiii xiv PREFACE in large parts, to the success of the Oakland Athletics baseball team in the 2002 season. Prior to that season, they had hired new players in a till then atypical way, namely by not relying on scouts' experience but rather on sabermetrics, the technical term for empirical/statistical analysis of baseball. This particular story of general manager Billy Beane relying on the use of analytics to assemble a competitive team despite Oakland's small budget has been written up in the famous book Moneyball: The Art of Winning an Unfair Game by Michael Lewis in 2003, which was released as movie in 2011 under the title Moneyball. The success of the Oakland Athletics team has inspired other teams in baseball and soon after in several other sports. Since then, sports analytics as a field has seen a phenomenal development, having led inter alia to the developments of new journals such as the Journal of Sports Analytics whose first edition appeared in 2015. The present book inscribes itself in this context and aims at further contributing to this stimulating research area thanks to its unique feature of targeting academicians and sports fans. Content of the Present Book Various popular sports will be described in this book from a scien- tific and data-driven perspective. The book covers baseball (Chapter 1 by Albert), basketball (Chapter 2 by Manisera, Sandri and Zuc- colotto), both baseball and basketball from the perspective of mea- surement theory in sports (Chapter 3 by Miller), football (Chapter 4 by Brefeld, Lasek and Mair and Chapter 5 by Groll, Schauberger and Van Eetvelde), running (Chapter 6 by Theisen, Nielsen and Malisoux), net games in general (Chapter 7 by Lames) and tennis in particular (Chapter 8 by Kovalchik and Chapter 9 by Koning and Boot, who fo- cus on betting aspects).
Details
-
File Typepdf
-
Upload Time-
-
Content LanguagesEnglish
-
Upload UserAnonymous/Not logged-in
-
File Pages30 Page
-
File Size-