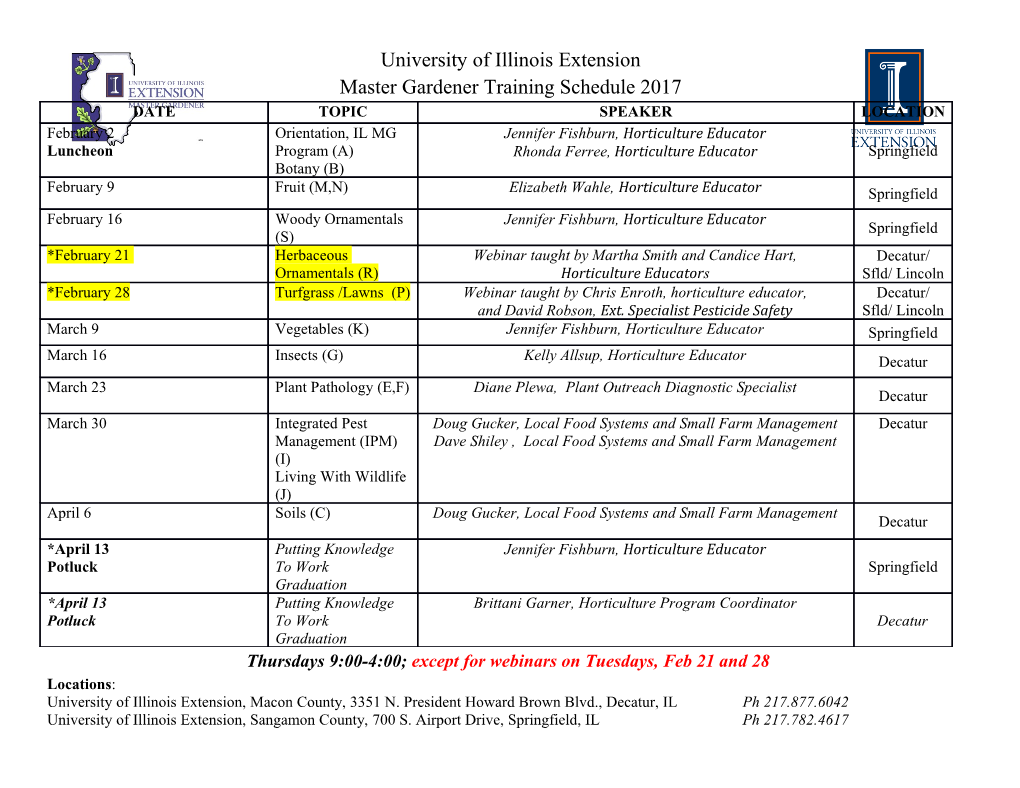
Basic Statistical Concepts Statistical Population • The entire underlying set of observations from which samples are drawn. – Philosophical meaning: all observations that could ever be taken for range of inference • e.g. all barnacle populations that have ever existed, that exist or that will exist – Practical meaning: all observations within a reasonable range of inference • e.g. barnacle populations on that stretch of coast 1 Statistical Sample •A representative subset of a population. – What counts as being representative • Unbiased and hopefully precise Strategies • Define survey objectives: what is the goal of survey or experiment? What are your hypotheses? • Define population parameters to estimate (e.g. number of individuals, growth, color etc). • Implement sampling strategy – measure every individual (think of implications in terms of cost, time, practicality especially if destructive) – measure a representative portion of the population (a sample) 2 Sampling • Goal: – Every unit and combination of units in the population (of interest) has an equal chance of selection. • This is a fundamental assumption in all estimation procedures •How: – Many ways if underlying distribution is not uniform » In the absence of information about underlying distribution the only safe strategy is random sampling » Costs: sometimes difficult, and may lead to its own source of bias (if sample size is low). Much more about this later Sampling Objectives • To obtain an unbiased estimate of a population mean • To assess the precision of the estimate (i.e. calculate the standard error of the mean) • To obtain as precise an estimate of the parameters as possible for time, effort and money spent 3 Measures of location • Population mean () - the average value • Sample mean = y estimates • Population median - the middle value • Sample median estimates population median • In a normal distribution the mean=median (also the mode), this is not ensured in other distributions Y Y Mean & median Median Mean Measures of dispersion • Population variance (2) - average sum of squared deviations from mean • Measured sample variance (s2) estimates population variance 2 (xi - x) n -1 • Standard deviation (s) – square root of variance – same units as original variable 4 Measures (statistics) of Dispersion 2 Population Sum of Squares (xi - ) 2 Sample Sum of Squares SS = (xi - x) (x - )2 2 i Population variance = n • Note, units are squared • Denominator is (n) (x - x)2 2 i Sample variance s = n -1 • Note, units are squared • Denominator is (n-1) Sample 2 (xi - x) standard deviation s = n -1 • Note, units are not squared More Statistics of Dispersion 2 s s = Standard error of the mean sx = • This is also the Standard Deviation n n of the sample means s Coefficient of variation CV = • Measurement of variation x independent of units • Expressed as a percentage of mean (xi -x ) (yi -y ) Covariance sxy = • Measure of how two variables covary n -1 • Range is between -8 and + 8 • Value depends in part on range in data – bigger numbers yield bigger values of covariance 5 Types of estimates • Point estimate – Single value estimate of the parameter, e.g. y is a point estimate of , s is a point estimate of • Interval estimate – Range within which the parameter lies known with some degree of confidence, e.g. 95% confidence interval is an interval estimate of Sampling distribution The frequency (or probability) distribution of a statistic (e.g. sample mean): • Many samples (size n) from population • Calculate all the sample means • Plot frequency distribution of sample means (sampling distribution) 6 P(y) y y Multiple samples - P(y) - multiple sample means y- Sampling distribution of sample means True Mean = 25 Means 21.5 36 22.3 22 27 19 23.0 41 23.9 12 24.9 25 33 23 25.1 25.8 31 26.5 Mean = 21.5 27.8 10 20 30 40 29.9 23 36 24 28 28 25 21 17 16 40 Mean = 25.8 Number of cases Estimate of Mean 7 Sampling distribution of mean • The sampling distribution of the sample mean approaches a normal distribution as n gets larger - Central Limit Theorem. • The mean of this sampling distribution is , the mean of original population. Large number of Samples 16 0.3 Proportion per Bar per Proportion 12 0.2 8 # of cases # 0.1 4 Probability 0 0.0 15 20 25 30 35 Estimate of Mean Estimate of Mean (x) 8 Sampling distribution of mean • The sampling distribution of the sample means approaches a normal distribution as n gets larger - Central Limit Theorem. • The mean of this sampling distribution is , the mean of original population. • The standard deviation of this sampling distribution is approximated by s/n, the standard deviation of any given sample divided by square root of sample size - the standard error of the mean. Standard deviation can be calculated for any distribution The standard deviation of the distribution of sample means can be calculated the same as for a given sample x (x - x)2 sx = i N -1 Where: 1. x = mean of the means and ~ Probability number of 2.5% 2.5% ~2 s ~2 sx means used in x distribution Estimate of Mean (x) 9 Standard deviation can be calculated for any distribution The standard deviation of the distribution of sample means can be calculated the same as for a given sample (x - x)2 sx = i However: N -1 To do so would require an x immense sampling effort, hence an approximation is used: s sx ~ SEM = n Where: s = sample standard deviation Probability 2.5% 2.5% and ~2 SEM ~2 SEM n = number of replicates in the sample Estimate of Mean (x) Standard error of mean • population SD estimated by sample SE: s/n • measures precision of sample mean • how close sample mean is likely to be to true population mean 10 Standard error of mean • If SE is low: – repeated samples would produce similar sample means – therefore, any single sample mean likely to be close to population mean • If SE is high: – repeated samples would produce very different sample means – therefore, any single sample mean may not be close to population mean Effect of Standard error on estimate of (assume df= large) 1 SEM=2 1 SEM=5 0.30 0.30 0.24 0.24 y t y i 0.18 t l i i 0.18 l i b b a a b b o o r 0.12 r P 0.12 P ~2 SEM ~2 SEM 0.06 0.06 2.5% 2.5% ~2 SEM ~2 SEM 0.00 0.00 0 10 20 30 40 0 10 20 30 40 Estimate of Mean Estimate of Mean 16 24 11 Worked example Lovett et al. (2000) measured the 2- concentration of SO4 (sulfate) in 39 North American forested streams (qk2002, Box 2.2) 2- Statistic Value Stream SO4 (mmol.L-1) Sample mean 61.92 Santa Cruz 50.6 Sample median 62.10 Colgate 55.4 Sample variance 27.46 Halsey 56.5 Sample SD 5.24 Batavia Hill 57.5 SE of mean 0.84 Interval estimate • How confident are we in a single sample estimate of , i.e. how close do we think our sample mean is to the unknown population mean. • Remember is a fixed, but unknown, value. • Interval (range of values) within which we are 95% (for example) sure occurs - a confidence interval 12 Distribution of sample means 99% 95% P( y ) y Calculate the proportion of sample means within a range of values. Transform distribution of means to a distribution with mean = 0 and standard deviation = 1 t statistic y s / n 13 0.4 0.3 y t i l i Null distribution b a 0.2 b o r P 0.1 0.0 -5 -4 -3 -2 -1 0 1 2 3 4 5 t = y s / n t statistic – interpretation and units • The deviation between the sample and population mean is expressed in terms of Standard error (i.e. Standard deviations of the y sampling distribution) • Hence the value of t’s are in standard errors • For example t=2 indicates s / n that the deviation (y- ) is equal to 2 x the standard error 14 The t statistic •This t statistic follows a t-distribution, which has a mathematical formula. • Same as normal distribution for n>30 otherwise flatter, more spread than normal distribution. • Different t distributions for different sample sizes < 30 (actually df which is n-1). 0.4 N=30 0.3 Null distributions N=3 y t i l i b a 0.2 b o r P 0.1 0.0 -5 -4 -3 -2 -1 0 1 2 3 4 5 t = y s / n 15 Two tailed t-values Probabilities of t = y occurring outside the range s / n –tdf to + tdf Probability Degrees of Freedom .01 .02 .05 .10 .20 1 63.66 31.82 12.71 6.314 3.078 4 df 2 9.925 6.965 4.303 2.920 1.886 3 5.841 4.541 3.182 2.353 1.638 4 4.604 3.747 2.776 2.132 1.533 95% 5 4.032 3.365 2.571 2.015 1.476 -2.78 +2.78 10 3.169 2.764 2.228 1.812 1.372 -5 -4 -3 -2 -1 0 1 2 3 4 5 15 2.947 2.602 2.132 1.753 1.341 t = y 20 2.845 2.528 2.086 1.725 1.325 s / n 25 2.787 2.485 2.060 1.708 1.316 z 2.575 2.326 1.960 1.645 1.282 One and two tailed t-values (df 4) Degrees of Freedom .005/.01 .01/.02 .025/.05 .05/.10 .10/.20 1 63.66 31.82 12.71 6.314 3.078 2 9.925 6.965 4.303 2.920 1.886 3 5.841 4.541 3.182 2.353 1.638 4 4.604 3.747 2.776 2.132 1.533 5 4.032 3.365 2.571 2.015 1.476 10 3.169 2.764 2.228 1.812 1.372 15 2.947 2.602 2.132 1.753 1.341 20 2.845 2.528 2.086 1.725 1.325 25 2.787 2.485 2.060 1.708 1.316 z 2.575 2.326 1.960 1.645 1.282 2 tailed 1 tailed 1 tailed 95% 95% -2.132 95% -2.78 +2.78 +2.132 -5 -4 -3 -2 -1 0 1 2 3 4 5 -5 -4 -3 -2 -1 0 1 2 3 4 5 -5 -4 -3 -2 -1 0 1 2 3 4 5 t = y s / n 16 The t statistic •This t statistic follows a t-distribution, which has a mathematical formula.
Details
-
File Typepdf
-
Upload Time-
-
Content LanguagesEnglish
-
Upload UserAnonymous/Not logged-in
-
File Pages23 Page
-
File Size-