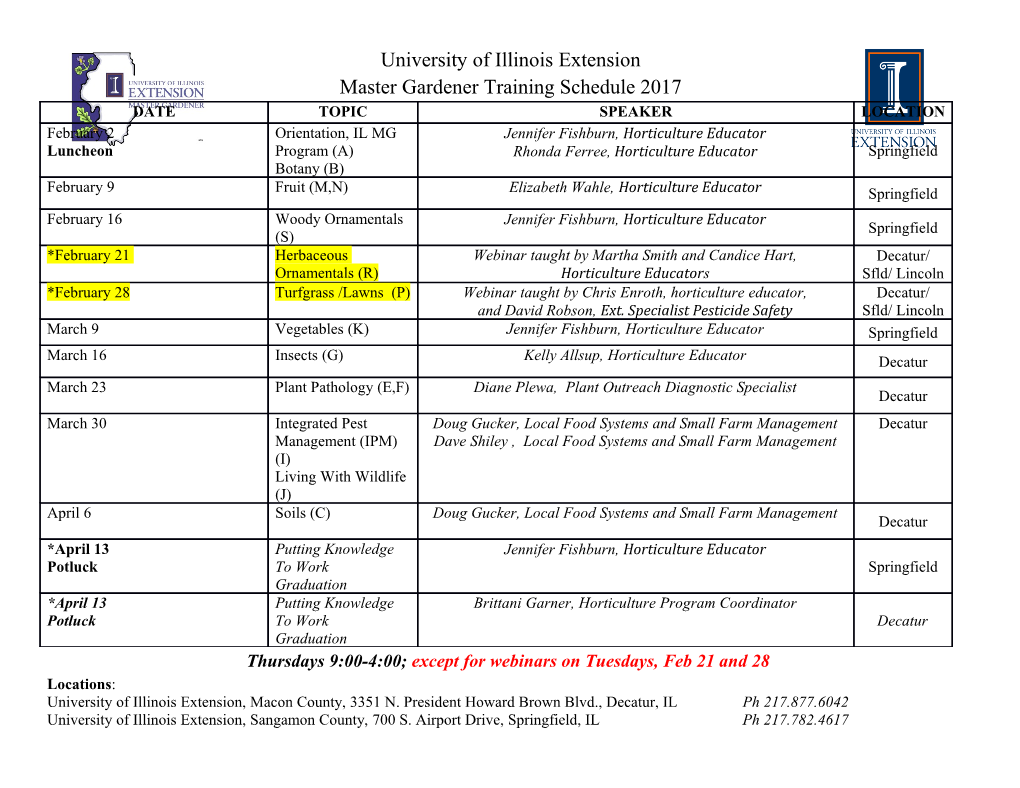
⃝c 2018 Xiang Ren MINING ENTITY AND RELATION STRUCTURES FROM TEXT: AN EFFORT-LIGHT APPROACH BY XIANG REN DISSERTATION Submitted in partial fulfillment of the requirements for the degree of Doctor of Philosophy in Computer Science in the Graduate College of the University of Illinois at Urbana-Champaign, 2018 Urbana, Illinois Doctoral Committee: Professor Jiawei Han, Chair & Director of Research Professor Tarek F. Abdelzaher Professor Heng Ji Professor ChengXiang Zhai ABSTRACT In today's computerized and information-based society, text data is rich but often also \messy". We are inundated with vast amounts of text data, written in different genres (from grammatical news articles and scientific papers to noisy social media posts), covering topics in various domains (e.g., medical records, corporate reports, and legal acts). Can computational systems automatically identify various real-world entities mentioned in a new corpus and use them to summarize recent news events reliably? Can computational systems capture and represent different relations between biomedical entities from massive and rapidly emerging life science literature? How might computational systems represent the factual information contained in a collection of medical reports to support answering detailed queries or running data mining tasks? While people can easily access the documents in a gigantic collection with the help of data management systems, they struggle to gain insights from such a large volume of text data: document understanding calls for in-depth content analysis, content analysis itself may require domain-specific knowledge, and over a large corpus, a complete read and analysis by domain experts will invariably be subjective, time-consuming and relatively costly. To turn such massive, unstructured text corpora into machine-readable knowledge, one of the grand challenges is to gain an understanding of the typed entity and relation structures in the corpus. This thesis focuses on developing principled and scalable methods for extracting typed entities and relationship with light human annotation efforts, to overcome the barriers in dealing with text corpora of various domains, genres and languages. In addition to our effort-light methodologies, we also contribute effective, noise-robust models and real-world applications in two main problems: • Identifying Typed Entities: We show how to perform data-driven text segmen- tation to recognize entities mentioned in text as well as their surrounding relational phrases, and infer types for entity mentions by propagating \distant supervision" (from external knowledge bases) via relational phrases. In order to resolve data sparsity issue during propagation, we complement the type propagation with clustering of function- ally similar relational phrases based on their redundant occurrences in large corpus. Apart from entity recognition and coarse-grained typing, we claim that fine-grained entity typing is beneficial for many downstream applications and very challenging due to the context-agnostic label assignment in distant supervision, and we present prin- cipled, efficient models and algorithms for inferring fine-grained type path for entity ii mention based on the sentence context. • Extracting Typed Entity Relationships: We extend the idea of entity recognition and typing to extract relationships between entity mentions and infer their relation types. We show how to effectively model the noisy distant supervision for relationship extraction, and how to avoid the error propagation usually happened in incremental extraction pipeline by integrating typing of entities and relationships in a principled framework. The proposed approach leverages noisy distant supervision for both entities and relationships, and simultaneously learn to uncover the most confident labels as well as modeling the semantic similarity between true labels and text features. In practice, text data is often highly variable: corpora from different domains, genres or languages have typically required for effective processing a wide range of language resources (e.g., grammars, vocabularies, and gazetteers). The massive and messy nature of text data poses significant challenges to creating tools for automated extraction of entity and relation structures that scale with text volume. State-of-the-art information extraction systems have relied on large amounts of task-specific labeled data (e.g., annotating terrorist attack-related entities in web forum posts written in Arabic), to construct machine-learning models (e.g., deep neural networks). However, even though domain experts can manually create high- quality training data for specific tasks as needed, both the scale and efficiency of such a manual process are limited. This thesis harnesses the power of \big text data" and focuses on creating generic solutions for efficient construction of customized machine-learning models for mining typed entities and relationships, relying on only limited amounts of (or even no) task-specific training data. The approaches developed in the thesis are thus general and applicable to all kinds of text corpora in different natural languages, enabling quick deployment of data mining applications. We provide scalable algorithmic approaches that leverage external knowledge bases as sources of supervision and exploit data redundancy in massive text corpora, and we show how to use them in large-scale, real-world applications, including structured exploration and analysis of life sciences literature, extracting document facets from technical documents, document summarization, entity attribute discovery, and open-domain information extraction. iii To my wonderful parents, Yanhong Wang and Yongkui Ren, for their love and support. iv ACKNOWLEDGMENTS I am greatly indebted to my Ph.D. advisor, Jiawei Han, one of the kindest and nicest people I have ever met. His passion and dedication on research has deeply influence me. Even during weekend nights, he spent hours remotely to give me advice on my research projects and plans, and to teach me how to do research, write scientific papers and manage all sorts of things in my Ph.D. career. He spent his sabbatical on campus, working closely with every student and providing detailed advices. Over the past five years, Jiawei did not just prepare me for every step of the Ph.D. degree, but also for an independent academic career by involving me in a comprehensive set of academic activities including grant proposal writing, paper reviewing, PI meetings, guest lectures, student mentoring, and following up with many constructive and timely feedback and advice. He has also been extremely supportive and open-mined, always encouraging me to explore various new problems, to think from a bigger picture, and to learn from other people's work. Moreover, Jiawei has been always fair, giving credit and generous; he always supported our trips to different conferences (even when we only got short papers or demo papers accepted there). During my last year at UIUC, he spent lots of efforts on my job search, by providing timely feedbacks on my application documents and job talk slides, giving me tips during the whole application process, and connecting me to relevant folks at different places. Even until the final version of this thesis, Jiawei has been providing insightful comments and suggestions. No matter how much I write in this note, it is impossible to express all my gratitude to him. I would also like to say thank you to all the other thesis committee members, Tarek F. Abdelzaher, Heng Ji and Cheng-Xiang Zhai, for giving me valuable feedback and asking insightful questions during my thesis proposal and defense. I would like to particularly thank Cheng for spending a lot of time to give me detailed comments on this thesis, help improve the content, and also provide lots of tips and advices on my job search process. I am grateful to Heng and Tarek for not only sitting on my dissertation committee, but also advising me through my job application process, answering a lot of questions, and suggesting interesting future directions for my work. I have had the awesome opportunity to work with numerous great mentors during summer internships. I went back to Beijing, China in my first summer and spent the summer at Microsoft Research Asia (where I stayed for over a year during my college time). I got the chances to know new and industry-scale problems, and learned different ways of thinking about solution and styles of doing research. My second and third summers were at Microsoft v Research Redmond, working with the data management group and ISRC group respectively. I want to thank Tao Cheng, Bolin Ding, Yuanhua Lv, Kuan-san Wang, Yang Song and Hao Ma, for their mentorship and guidance during my internship. I really appreciate the time working with the amazing peers in the Data Mining group at UIUC. I thank all my friends in the group: Jialu Liu (thank you for all the discussions we had on many research ideas as well as views on future directions of our research), Xiao Yu (I still remember we started collaborating on recommender system projects right after my first project ended, we spent lots of time together doing experiments and writing papers; it turns out working quite well and we got some nice work done before you graduated), Fangbo Tao (it is great to have you in the group that you are actively connecting folks together and bring joy to the group), Hyungsul Kim (thank you for being my very first student mentor and guiding me on how to do research in my first year). I would also like to thank all the master students and junior Ph.D. students that I am honored to work with, including Ellen Wu, Wenqi He, Meng Qu, Qi Zhu, Hongkun Yu, Urvashi Khandelwal, Bradley Sturt and Tobias Lei. All of you did an amazing job on the collaboration projects and I am very grateful to enjoy the research together with you. Grad school was not just about work. I was fortunate to have wonderful friends around me who made the experience more fun, and less daunting: Gong Chen and Annlin Lee, Ray Wang, Qi Wang, De Liao, Wenzhu Tong; my early officemates, Aston Zhang, Hongwei Wang and Huan Gui.
Details
-
File Typepdf
-
Upload Time-
-
Content LanguagesEnglish
-
Upload UserAnonymous/Not logged-in
-
File Pages137 Page
-
File Size-