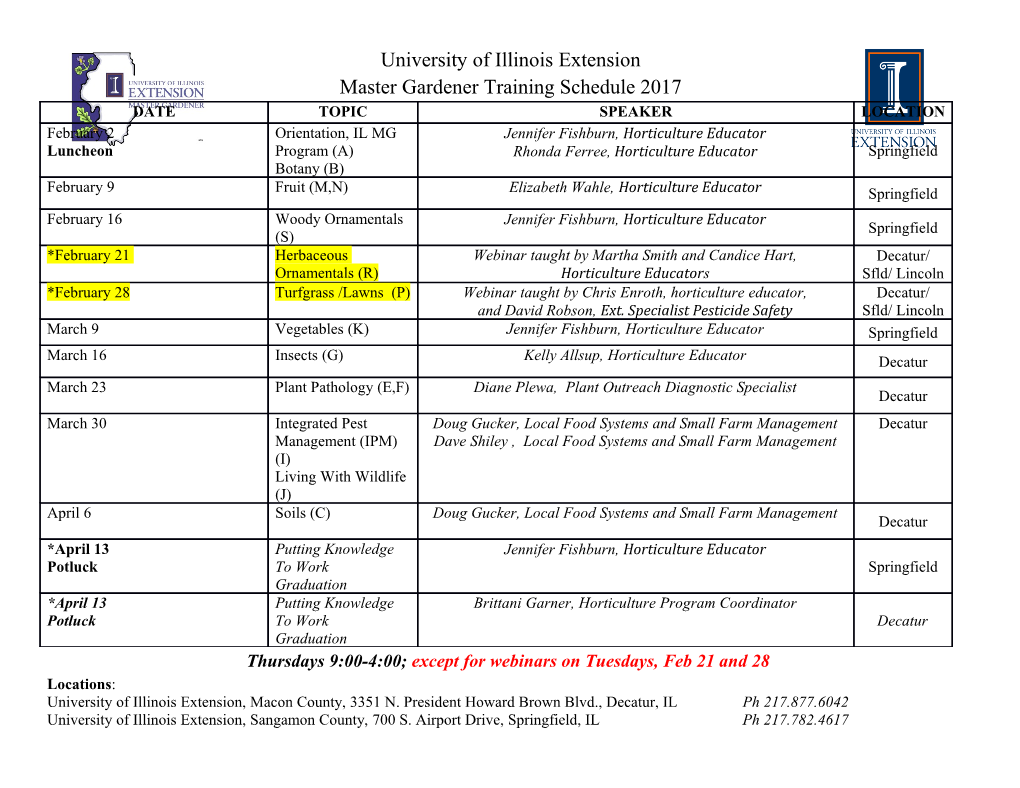
The Pitfalls of Prediction by Greg Ridgeway The criminal justice system should take advantage of the latest scientific developments to make reliable predictions. rediction is common in every- be deployed to prevent future crime, day life. We make predictions and victims wrestle with the uncer- Pabout the length of our morn- tain odds of being revictimized. ing commute, the direction of the stock market, and the outcomes There is a long history of research on of sporting events. Most of these prediction in criminology and criminal common-sense predictions rely justice, and two developments are on cognitive shortcuts — or helping the criminal justice system heuristics — that shape our expec- improve its ability to make reliable, tations of what is likely to occur in scientific predictions. First, more and the future. But these heuristics are more jurisdictions are accumulating not necessarily true; they rely on rich data and are getting better at cognition, memory and sensory linking across their data sources. impressions rather than a balanced Second, a growing set of sophis- analysis of facts. Consequently, they ticated analytic prediction tools is can result in biased predictions. available to help agencies make decisions about future events, The challenge of predicting the future unknown risks and likely outcomes. has always been at the heart of Editor's Note: This article was the criminal justice system. Judges Practitioners can now combine presented to seven law enforcement weigh the risks of releasing offend- expert assessment with data-driven agencies that were developing ers to probation, police agencies try prediction models to discern how predictive policing programs. to anticipate where officers should much risk a probationer poses, 34 NIJ JOURNAL / ISSUE NO. 271 ■ FEBRUARY 2013 determine whether a pair of illicit drug transactions signals the emer- NIJ’s Role in Predictive Policing gence of a drug market, or project whether crime will increase or decrease during the next month. More and more, police departments aw enforcement work is frequently reactive: Officers respond to are using forecasting tools as a basis Lcalls for service, control disturbances and make arrests. But law for formal predictive policing efforts; enforcement work is becoming increasingly proactive: Departments these statistical prediction methods combine data with street intelligence and crime analysis to understand inform their prevention strategies so why a problem arises and predict what might happen next if they take they can anticipate rather than react certain actions. 1 to crime. (See sidebar, “NIJ’s Role in NIJ is supporting predictive policing efforts in a number of ways: Predictive Policing,” on this page.) n Predictive policing symposiums. NIJ convened two symposiums Although the science of prediction at which researchers, practitioners and law enforcement leaders continues to improve, the work developed and discussed the concept of predictive policing and its of making predictions in criminal impact on crime and justice. Read summaries of both sessions at justice is plagued by persistent http://www.nij.gov/topics/law-enforcement/strategies/predictive- shortcomings. Some stem from policing/symposium/welcome.htm. unfamiliarity with scientific strategies n Predictive policing grants. The Chicago and Shreveport police or an over-reliance on timeworn — departments are using grants to explore data-driven policing but unreliable — prediction habits. strategies. In Phase 1, they received funding to identify a problem If prediction in criminal justice is to and develop predictive policing strategies to solve it. In Phase 2, take full advantage of the strength they were awarded additional funding to implement and evaluate of these new tools, practitioners, the strategies. For more on these grants, see http://www.nij.gov/nij/ analysts, researchers and others topics/law-enforcement/strategies/predictive-policing/symposium/ must avoid some commonplace discussion-demonstrations.htm. mistakes and pitfalls in how they make predictions. For more information: Pitfall #1: Trusting Expert n To learn more about predictive policing in general, read the NIJ Prediction Too Much Journal article “Predictive Policing: The Future of Law Enforcement?” Using data and computers to predict at http://www.nij.gov/journals/266/predictive.htm. or help experts predict shows prom- ise, but the pace of adoption has not matched that promise. Why? Perhaps we trust ourselves more than we offices. Some researchers have would vote on the 68 upcoming trust machines. found that physicians have “a high cases on the 2002 docket. Based on regard for their own decision-making their knowledge of the justices and For example, more than 30 years ability and are afraid of any competi- the ins and outs of the court, they ago, Stanford scientists developed tion from computers.”3 correctly predicted how the Supreme a pathbreaking, computer-based Court would vote on 59 percent of medical expert system that could So how do experts and machines the cases. synthesize patient features and compare in their ability to predict in therapeutic options.2 The system, the justice system? Researchers used a computer pro- called MYCIN, outperformed practi- gram to make the same prediction. tioners in selecting the right antibiotic Consider this example: A panel of The computer analyzed 628 previous treatments. Despite MYCIN’s 83 experts — law professors, deans Supreme Court cases and generated demonstrated success and similar of law schools and others who had data-derived rules.4 The researchers kinds of computer-based prediction practiced before or clerked at the created a decision-tree prediction successes, we still do not see these U.S. Supreme Court — set out to model based on a simple set of systems being used in our doctors’ predict how the U.S. Supreme Court these rules. The Pitfalls of Prediction | 35 NIJ JOURNAL / ISSUE NO. 271 ■ FEBRUARY 2013 Pitfall #2: Clinging to What You Figure 1. Decision Tree for Supreme Court Justice Learned in Statistics 101 Sandra Day O'Connor If your knowledge of prediction is Was the lower court decision liberal? limited to what gets covered in intro- ductory statistics courses, you are Yes probably unfamiliar with the predic- tion model used above. Instead, you No Reverse most likely learned how to check model assumptions and carefully test hypotheses. But when it comes Was the case from the 2nd District, to prediction, the rules are different 3rd District, D.C. or Federal Circuit? and rather simple: Are the predic- tions accurate, and can you get them Yes when you need them? You can judge No the quality of a specific prediction Affirm model by considering the following: Performance criteria. Do the Was the respondent an entity other model’s goals and constraints match than the U.S. government? the intended use? Methods that are good at predicting, for example, No whether an injury will result from a Yes mission are not necessarily the same Reverse as those that are good at predicting the number of days an officer will Was the case concerning civil rights, be out with that injury. If you are 1st Amendment, economic or federalism issue? planning a tactical unit’s staffing, it is important for you to know the No Yes expected person-hours that will be lost to injuries. Thus, using a model that can accurately predict only Affirm Reverse whether an injury will occur — and not how long an officer will be out — would be insufficient. Figure 1 shows the decision tree for So what can we take away from Accuracy. Can the model make Justice Sandra Day O’Connor. Based this example? It should lead us to accurate forecasts? More specifically, on a simple set of rules — such as question — but not necessarily the implemented model should be whether the lower court decision dismiss — the predictions of experts, better at prediction than the agency’s was liberal — the model was able to including ourselves. Of course, not current practice. For example, if cops predict how Justice O’Connor would all cases afford us the data to build are allocating resources to neighbor- decide 70 percent of the cases in predictive models. But if we have hoods where they think crime will 2002. Using similar decision trees for data that we can use to construct spike, then going forward we should the other eight justices,5 the model predictive models, then we should test whether the prediction model is correctly predicted the majority build the models and test them even better at selecting those neighbor- opinion in 75 percent of the cases, if our expert detectives, probation hoods. If a probation officer is assign- substantially outperforming the officers and others in the field indi- ing remote monitoring anklets to DUI experts’ 59 percent. The experts cate that they already know how to probationers, then we should test lost out to a machine that had a predict. They may be as surprised as whether the prediction model is few basic facts about the cases. the expert panel was in the Supreme better at picking which DUI proba- Court example. tioners will reoffend in the next six 36 | The Pitfalls of Prediction NIJ JOURNAL / ISSUE NO. 271 ■ FEBRUARY 2013 months. For a prediction model accurate in predicting, say, when and to be useful, it must outperform where a gang retaliation shooting will Prediction can play practice as usual. take place, then a more transparent a major role in model might not be worth the cost. Computation time. Can you apply This issue will be discussed further the criminal justice the prediction model in a reasonable under Pitfall #5. amount of time? Some models can system. Even small be computationally intensive to run Pitfall #3: Assuming One and use. There is little point in using Method Works Best for improvements in where a model that cannot produce predic- All Problems tions in time for them to be useful.
Details
-
File Typepdf
-
Upload Time-
-
Content LanguagesEnglish
-
Upload UserAnonymous/Not logged-in
-
File Pages7 Page
-
File Size-