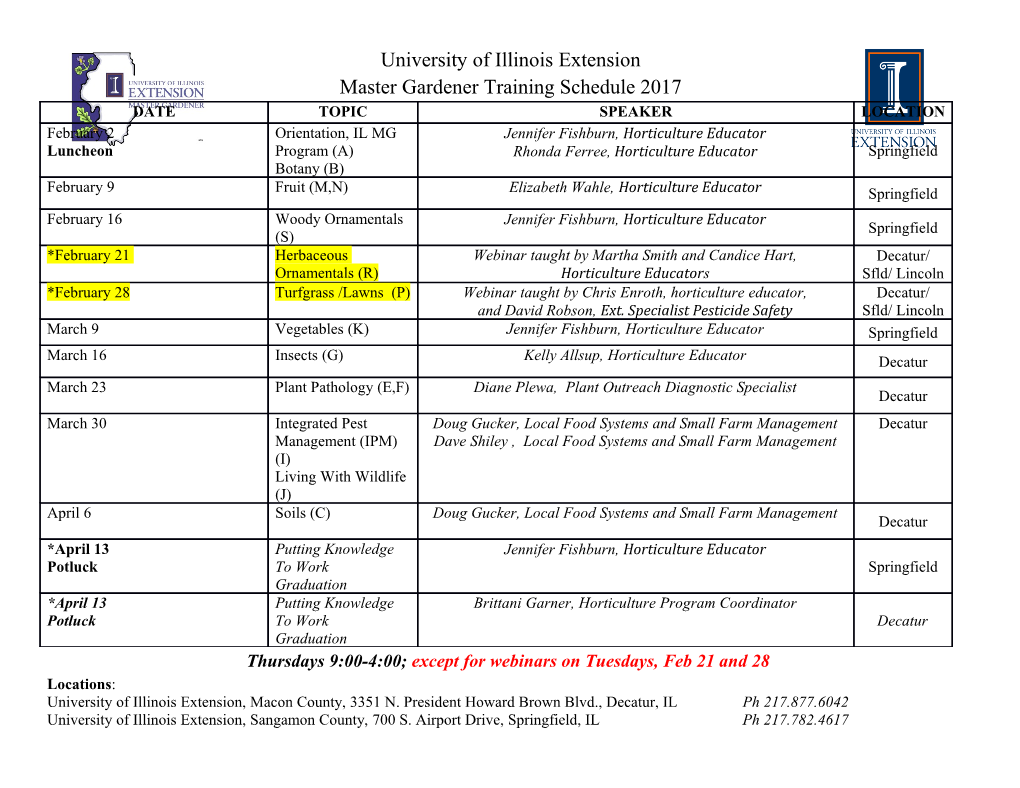
Linear algebra review Dec 10, 2014 1. Solving system of linear equations: Given a system of linear equation Ax = b, where A is an m n matrix. × (a) We first observe the augmented matrix and perform elementary row operations to find the reduced echelon form: [A b] [R c]. | −→ | If there are some rows in [R c] of the form | [0 0 d], ··· where d =0,thenthesystemoflinearequationshasnosolutionandisinconsis- tent; otherwise$ we only need to observe the coefficient matrix A: If rank A = n, then there is a unique solution (no free variables); If rank A<n, then there are infinitely many solutions (still has free variables). Note rank A can never be greater than either m or n. (b) To find the general solutions to the system of linear equations, we still need to use the augmented matrix [R c], the concrete steps are as follows: | Recover a “new” system of linear equations from [R c], solve basic variables using free variables, write them in the parametric vector| form. (c) If A is square matrix (number of the equations equals the number of variables), then Cramer’s rule is an efficient method to determine the existence as well as to calculate the exact solutions of a system of linear equations. There will be formulas involving determinant for the solution. 2. Homogeneous system of linear equations Ax = 0 There are only two possible types of solutions for homogeneous system: If rank A = n (column vectors of A are linearly independent), only trivial solution. If rank A<n, infinitely many solutions. The set of all solutions forms a vector space, called Null space of A. 1 3. Linear dependence and independence (a) Definition: Ax = 0 only has trivial solution or not (b) Criterion: rank A = n (independent) or not (dependent). 4. Subspace, basis, dimension (a) Definitions and examples (e.g. linear combination of a set of vectors) (b) Some distinguished examples: Row(A), Col(A), Null(A), Eigλ(A), W ⊥ (c) Basis and dimension (d) Relations: T Row(A)=Col(A ), Null(A)=Row(A)⊥. 5. Invertible matrix, elementary matrix (a) Definition and Criterion, 2 2example × (b) Calculate the inverse: Elementary row operations, adjoint matrix (c) Correspondence between elementary matrices and elementary row operations; in- verse of the elementary matrices 6. Determinant (a) Cofactor expansions (b) Properties under elementary row operations (c) Applications: Geometry of determinant, Cramer’s rule, adjoint matrix. 7. Linear transformation (a) Definition and examples, T : Rn Rm maps x to Ax. −→ (b) Distinguished examples: Orthogonal projections, differential operators (c) One-to-one (rank A =n)andonto(rankA =m). 8. Change of basis (a) Change of the coordinate from basis to standard basis: B Let = b ,...,b , B { 1 n} and let P =[b1 bn]. B |···| Then x = P [x] . B B 2 (b) Change of the coordinate from basis to basis : B C [x] = P [x] , C C←B B where 1 P = P − P . C←B C B (c) The linear transformation under change of basis The general formula is on Page 289 of the book. A special case, let T : Rn Rn −→ x Ax %−→ and = b1,...,bn , then B { } 1 [T ] = P − AP . B B B 1 This result can be applied to a diagonalizable matrix A = PDP− , where P is invertible and D is diagonal. If we change the standard basis to a basis consisting of columns of P (hence P = P ), then [T ] = D. B B B 9. Eigenvalues and Eigenspaces (a) Definitions (b) Characteristic polynomials 10. Diagonalization (a) Definition (b) Examples: Matrices with mutually distinct eigenvalues. (c) Criterion: For each eigenvalue λ, mult(λ)=dimEig (A)=n rank(A λI ) λ − − n (d) Find invertible matrix P (formed by eigenvectors as its columns) and D (eigen- 1 values as the diagonal entries, correspondingly) such that A = PDP− . 11. Inner product and orthogonality (a) Properties, norm, normalize, some inequalities (b) Orthogonal (orthonormal) sets, orthogonal (orthonormal) basis (c) Decomposition of a vector under orthogonal (orthonormal) basis (d) Orthogonal matrix (e) Orthogonal complement 3 12. Gram-Schmidt process, QR decomposition The formula is on page 355, by applying Gram-Schmidt, a basis is converted to an orthogonal basis. 13. Orthogonal projection Let ProjW y = PW y to denote the orthogonal projection of a vector y to a subspace m W R . Let x1,...,xn be a basis of W and A =[x1 xn]. ⊂ { } |···| T 1 T (a) Use projection matrix to find ProjW y = PW y, where PW =(A A)− A . (b) Apply Gram-Schmidt on the basis x ,...,x to obtain an orthogonal basis { 1 n} u1,...,un , then ProjW y can be write explicitly under the orthogonal (orthonor- mal){ basis: } y u y u Proj y = · 1 u + + · n u . W u u 1 ··· u u n 1 · 1 n · n (c) There is a unique decomposition: for y Rm, ∈ y =ProjW y + z, where z W ⊥. ∈ 14. Inconsistent system of linear equations, least squares (a) Least-squares solution xˆ of Ax = b is a vector such that b Axˆ b Ax . ( − (≤( − ( The solution is actually ProjCol Ab, that is, T 1 T xˆ =(A A)− A b, Note here we require columns of A are linearly independent. (b) Line of least square is a special case of (a), see page 369 for the detail. 4.
Details
-
File Typepdf
-
Upload Time-
-
Content LanguagesEnglish
-
Upload UserAnonymous/Not logged-in
-
File Pages4 Page
-
File Size-