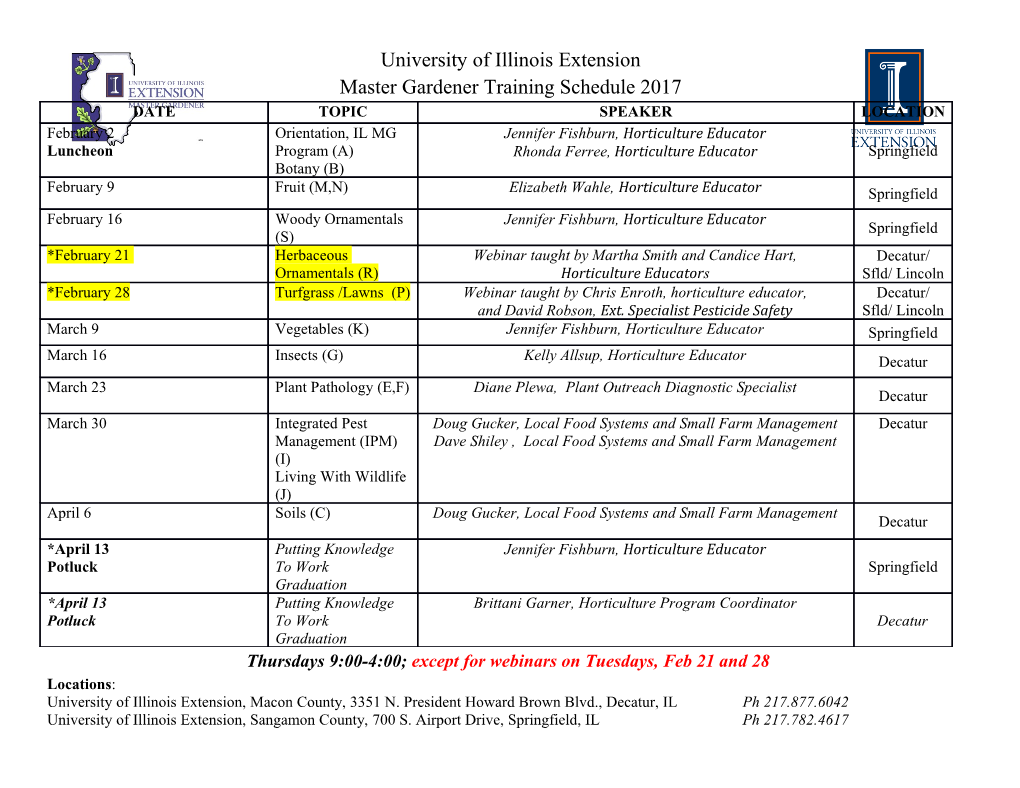
Workshop: Getting Started with R. UTM 14 Oct 2018 .© Dr. Norhaiza Ahmad Getting Started with for newbies STATISTICAL ANALYSIS ON IRIS DATA 14 October 2018 Dr. Norhaiza Ahmad Department of Mathematical Sciences Faculty of Science Universiti Teknologi Malaysia http://science.utm.my/norhaiza/ Workshop: Getting Started with R. UTM 14 Oct 2018 .© Dr. Norhaiza Ahmad IRIS DATASET Iris flower data set is a collection of data to quantifythe morphologicvariation of Iris flowers. The flowers were collected in the Gaspé Peninsula from the same pasture, and picked on the same dayand measured at the same time by the same person with the same apparatus. The data set consists of 50 samples from each of three species of Iris setosa (far left), Iris versicolor (centre) and Iris virginica). Four components of the flowers’ features were measured from each sample: the length and the width of the sepals and petals, in centimetres. Workshop: Getting Started with R. UTM 14 Oct 2018 .© Dr. Norhaiza Ahmad Iris dataset •The iris data is included in the R base package as a dataframe. > iris Sepal.Length Sepal.Width Petal.Length Petal.Width Species 1 5.1 3.5 1.4 0.2 setosa 2 4.9 3.0 1.4 0.2 setosa . 50 5.0 3.3 1.4 0.2 setosa 51 7.0 3.2 4.7 1.4 versicolor 52 6.4 3.2 4.5 1.5 versicolor . 100 5.7 2.8 4.1 1.3 versicolor 101 6.3 3.3 6.0 2.5 virginica 102 5.8 2.7 5.1 1.9 virginica . 149 6.2 3.4 5.4 2.3 virginica 150 5.9 3.0 5.1 1.8 virginica Workshop: Getting Started with R. UTM 14 Oct 2018 .© Dr. Norhaiza Ahmad TASK Call up IRIS dataset on R and analyze the dataset using the codes given to you. Workshop: Getting Started with R. UTM 14 Oct 2018 .© Dr. Norhaiza Ahmad Iris dataset iris #multivariate data on flower measurements head(iris) > head(iris) Sepal.Length Sepal.Width Petal.Length Petal.Width Species 1 5.1 3.5 1.4 0.2 setosa 2 4.9 3.0 1.4 0.2 setosa 3 4.7 3.2 1.3 0.2 setosa 4 4.6 3.1 1.5 0.2 setosa 5 5.0 3.6 1.4 0.2 setosa 6 5.4 3.9 1.7 0.4 setosa tail(iris) Workshop: Getting Started with R. UTM 14 Oct 2018 .© Dr. Norhaiza Ahmad Iris dataset summary(iris) Sepal.Length Sepal.Width Petal.Length Petal.Width Species Min. :4.300 Min. :2.000 Min. :1.000 Min. :0.100 setosa :50 1st Qu.:5.100 1st Qu.:2.800 1st Qu.:1.600 1st Qu.:0.300 versicolor:50 Median :5.800 Median :3.000 Median :4.350 Median :1.300 virginica :50 Mean :5.843 Mean :3.057 Mean :3.758 Mean :1.199 3rd Qu.:6.400 3rd Qu.:3.300 3rd Qu.:5.100 3rd Qu.:1.800 Max. :7.900 Max. :4.400 Max. :6.900 Max. :2.500 #mean and median appear close- indication data symmetric Workshop: Getting Started with R. UTM 14 Oct 2018 .© Dr. Norhaiza Ahmad Iris dataset #Say we want to analyse species versicolor only #Create subset of Species versicolor iris.vs = iris[51:100,1:4] #or iris.vs =iris[iris$Species=="versicolor",1:4] #mean and median appear close- indication data symmetric names(iris.vs) [1] "Sepal.Length" "Sepal.Width" "Petal.Length" "Petal.Width" Workshop: Getting Started with R. UTM 14 Oct 2018 .© Dr. Norhaiza Ahmad Iris dataset #DISPLAY DATA or hist(iris.vs[,1]) #tidy hist(iris.vs[,1],main=names(iris)[1],xlab=NULL) #change layout of graphs par(mfrow=c(1,2)) #1 row, 2 col layout hist(iris.vs[,1]) hist(iris.vs[,1],main=names(iris)[1],xlab=NULL) Workshop: Getting Started with R. UTM 14 Oct 2018 .© Dr. Norhaiza Ahmad Iris dataset #DISPLAY DATA or #Graphs for all measurements of iris versicolor par(mfrow=c(2,2)) hist(iris.vs[,1],main=names(iris)[1],xlab=NULL) hist(iris.vs[,2],main=names(iris)[2],xlab=NULL) hist(iris.vs[,3],main=names(iris)[3],xlab=NULL) hist(iris.vs[,4],main=names(iris)[4],xlab=NULL) #multi scatter-plots between variables > pairs(iris.vs) Workshop: Getting Started with R. UTM 14 Oct 2018 .© Dr. Norhaiza Ahmad Iris dataset #Correlation between variables or cor(iris.vs) Sepal.Length Sepal.Width Petal.Length Petal.Width Sepal.Length 1.0000000 0.5259107 0.7540490 0.5464611 Sepal.Width 0.5259107 1.0000000 0.5605221 0.6639987 Petal.Length 0.7540490 0.5605221 1.0000000 0.7866681 Petal.Width 0.5464611 0.6639987 0.7866681 1.0000000 #pairs Sepal.Length vs Petal.Length, and Petal.Length vs Petal Width #are most strongly correlated with respective correlations of #0.7540 and 0.7867 #Correlation test between Petal.Length vs Petal Width cor.test(iris.vs[,3],iris.vs[,4]) Pearson's product-moment correlation data: iris.vs[, 3] and iris.vs[, 4] t = 8.828, df = 48, p-value = 1.272e-11 alternative hypothesis: true correlation is not equal to 0 95 percent confidence interval: 0.6508311 0.8737034 sample estimates: cor #Significant linear correlation 0.7866681 Workshop: Getting Started with R. UTM 14 Oct 2018 .© Dr. Norhaiza Ahmad Iris dataset or #how to build a statistical model to predict a new Petal width given a new petal length? Use simple linear regression model (irisVS.lm=lm(iris.vs[,3]~iris.vs[,4])) Call: lm(formula = iris.vs[, 3] ~ iris.vs[, 4]) Coefficients: (Intercept) iris.vs[, 4] 1.781 1.869 #Petal width= 1.781+1.869*Petal Length Workshop: Getting Started with R. UTM 14 Oct 2018 .© Dr. Norhaiza Ahmad Iris dataset #Is there a difference between the average Petal Length of species Setosa and Versicolor? #assume the data are normally distributed iris.s =iris[iris$Species==”setosa",1:4] t.test(iris.s[,3],iris.vs[,3]) > t.test(iris.s[,3],iris.vs[,3]) Welch Two Sample t-test data: iris.s[, 3] and iris.vs[, 3] t = -39.4927, df = 62.14, p-value < 2.2e-16 alternative hypothesis: true difference in means is not equal to 0 95 percent confidence interval: -2.939618 -2.656382 sample estimates: mean of x mean of y 1.462 4.260 #Significant evidence to Reject the null hypothesis that there is no difference between the average petal length of species iris Setosa & Versicolor Workshop: Getting Started with R. UTM 14 Oct 2018 .© Dr. Norhaiza Ahmad Use package ggplot2 : IRIS #------------# Advanced- Use package ggplot2 : IRIS library(ggplot2) p1 = ggplot(data = iris, aes(x = Petal.Length, y = Petal.Width)); p1 #setgraph paper p2 = p1 + geom_point(aes(color = Species));p2 #use geom to specify what to plot p3 = p2 + geom_smooth(method='lm');p3 #add a linear regression model to fit the data p4 = p3 + xlab("Petal Length (cm)") + ylab("Petal Width (cm)") +ggtitle("PetalLgth vs Petal Width"); p4 #create/modify title Workshop: Getting Started with R. UTM 14 Oct 2018 .© Dr. Norhaiza Ahmad Other Example Workshop: Getting Started with R. UTM 14 Oct 2018 .© Dr. Norhaiza Ahmad Example: Student Admissions data > UCBdt Admit Gender Dept Freq 1 Admitted Male A 512 Aggregate data on applicants to 2 Rejected Male A 313 3 Admitted Female A 89 postgraduate school at Berkeley for the six 4 Rejected Female A 19 largest departments classified by admission 5 Admitted Male B 353 6 Rejected Male B 207 and gender. 7 Admitted Female B 17 8 Rejected Female B 8 9 Admitted Male C 120 10 Rejected Male C 205 11 Admitted Female C 202 Admission Levels: Admitted/Rejected 12 Rejected Female C 391 Gender: Male/Female 13 Admitted Male D 138 Department: A-F 14 Rejected Male D 279 15 Admitted Female D 131 16 Rejected Female D 244 17 Admitted Male E 53 18 Rejected Male E 138 19 Admitted Female E 94 20 Rejected Female E 299 21 Admitted Male F 22 22 Rejected Male F 351 23 Admitted Female F 24 24 Rejected Female F 317 1 5 Workshop: Getting Started with R. UTM 14 Oct 2018 .© Dr. Norhaiza Ahmad Simple Visual: Student Admissions -package plyr Highest admission for More males than Highest admission for department A compared females admitted to department A compared to the rest. Lowest the university to the rest. Lowest admission for department admission for department F F Dept. A & B discriminate Dept. A & B discriminate gender for admission. gender for admission. 1 6 Workshop: Getting Started with R. UTM 14 Oct 2018 .© Dr. Norhaiza Ahmad #------------- # Advanced- Use package plyr : Students Admission library(plyr) library(datasets) UCBdt <- as.data.frame(UCBAdmissions) overall <- ddply(UCBdt, .(Gender), function(gender) { temp <- c(sum(gender[gender$Admit == "Admitted", "Freq"]), sum(gender[gender$Admit == "Rejected", "Freq"])) / sum(gender$Freq) names(temp) <- c("Admitted", "Rejected") temp }) departmentwise <- ddply(UCBdt, .(Gender,Dept), function(gender) { temp <- gender$Freq / sum(gender$Freq) names(temp) <- c("Admitted", "Rejected") temp }) # A barplot for overall admission percentage for each gender. p1 <- ggplot(data = overall, aes(x = Gender, y = Admitted, width = 0.2)) p1 <- p1 + geom_bar(stat = "identity") + ggtitle("Overall admission percentage") + ylim(0,1) ;p1 # A 1x6 panel of barplots, each of which represents the # admission percentage for a department p2 <- ggplot(data = UCBdt[UCBdt$Admit == "Admitted", ], aes(x = Gender, y = Freq)) p2 <- p2 + geom_bar(stat = "identity") + facet_grid(. ~ Dept) + ggtitle("Number of admitted students\nfor each department") + theme(axis.text.x = element_text(angle = 90, hjust = 1)) ;p2 # A 1x6 panel of barplots, each of which represents the # number of admitted students for a department p3 <- ggplot(data = departmentwise, aes(x = Gender, y = Admitted)) p3 <- p3 + geom_bar(stat = "identity") + facet_grid(.
Details
-
File Typepdf
-
Upload Time-
-
Content LanguagesEnglish
-
Upload UserAnonymous/Not logged-in
-
File Pages17 Page
-
File Size-