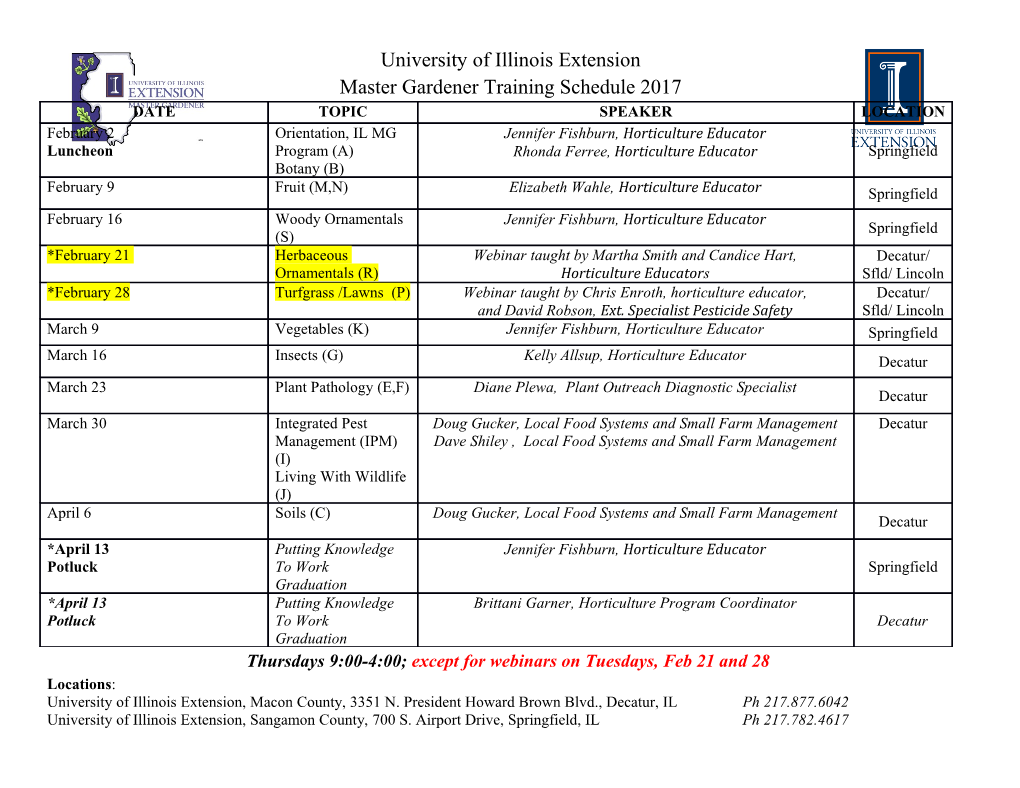
International Journal of Electrical and Electronics Engineering Research (IJEEER) ISSN 2250-155X Vol. 3, Issue 4, Oct 2013, 117-128 © TJPRC Pvt. Ltd. DESIGN & IMPLEMENTATION OF SYSTOLIC ARRAY ARCHITECTURE SWETA SINGH1 & N B SINGH2 1Worked on M.Tech (VLSI Design), CSIR-CEERI, Banasthali Vidyapeeth University, Vanasthali, Rajasthan, India 2Chief Scientist, MEMS MS & RF ICs Design, CSIR-Central Electronics Engineering Research Institute (CEERI), Pilani, Rajasthan, India ABSTRACT The paper describes the implementation of 2-D systolic array matrix multiplier architecture in RTL using one dimensional array to target the design on a appropriate FPGA/PROM/CPLD devices. It also discusses the digital realisation of a binary multiplier. The system development started with top-down planning approach and the blocks were designed using bottom-up implementation. The programs were written, simulated and synthesized using Mentor Graphics tools, ModelSim and Leonardo Spectrum. Results are presented in the paper. The design presented in the paper is an integral part of the higher level efficient systolic architecture. KEYWORDS: Systolic Array, DSP, Verilog and HDL INTRODUCTION A Parallel Algorithm [5], is an algorithm which can be executed a piece at a time on many different processing devices, and then put back together again at the end to get the correct result. Parallel algorithms[8], are valuable because of substantial improvements in multiprocessing systems and the rise of multi-core processors. In general, it is easier to construct a computer with a single fast processor than one with many slow processors with the same throughput. But processor speed is increased primarily by shrinking the circuitry, and modern processors are pushing physical size and heat limits. These twin barriers have flipped the equation, making multiprocessing practical even for small systems. Modelling parallel algorithm is more complicated than modelling sequential algorithm because in practice parallel computers tend to vary more in organization than do sequential computers. As a consequence, a large portion of the research on parallel algorithms has gone into the question of modelling, Although there has been no consensus on the right model, this research has yielded a better understanding of the relationship between the models. Any discussion of parallel algorithms requires some understanding of the various models and the relationship among them. In many situations, hardware description languages (HDL) such as VHDL, Verilog or SystemC is used to develop the functionality of the digital system, while the timing and control signal generation is either neglected or ignored. I have used a methodology wherein a hardware structure was conceptually laid out of the digital system under consideration. The system development started with top-down planning approach and the blocks were designed using bottom-up implementation. The programs were written, simulated and synthesized using Electronic Data Automation (EDA) tools such as ModelSim and Leonardo Spectrum. Instruction set such as transfer, arithmetic, logic, input, output and control instructions were implemented.. Flynn's taxonomy is a classification of computer architectures, proposed by Michael J. Flynn in 1966. According to Flynn’s taxonomy parallelism can be established by using one of these models, SISD has no parallelism. It is used in sequential computing system 118 Sweta Singh & N B Singh SIMD establishes data parallelism. MISD establishes instructions parallelism. MIMD establishes both data and instructions parallelism. Flynn’s taxonomy distinguishes multi-processor computer architectures according to how they can be classified along the two independent dimensions of Instruction and Data. Each of these dimensions can have only one of two possible states: Single or Multiple. The matrix below defines the 4 possible classifications according to Flynn: Figure 1: Flynn’s Taxonomy Classifications From scalar to superscalar, the simplest processors are scalar processors. Each instruction executed by a scalar processor typically manipulates one or two data items at a time. By contrast, each instruction executed by a vector processor operates simultaneously on many data items. An analogy is the difference between scalar and vector arithmetic. A superscalar processor is sort of a mixture of the two. Each instruction processes one data item, but there are multiple redundant functional units within each CPU thus multiple instructions can be processing separate data items concurrently. A superscalar architecture where a processor having multi functional units can be realized to execute multi instructions in a single control step to redundant functional units on the processor when it is also integrated with pipeline features then it maintains its enhanced feature. Superscalar has the redundant resources. Existing binary executable programs have varying degrees of intrinsic parallel During the project above classified models will be included to establish the architecture for the parallel processor. The applications may be the parallel ALU and array processors, i.e. systolic array architecture. Most of today’s algorithms are sequential, they specify a sequence of steps in which each step consists of a single operation. These algorithms are well suited to today’s computers, which basically perform operations in a sequential fashion. Although the speed at which sequential computers operate has been improving at an exponential rate for many years, the improvement is now coming at greater and greater cost. As a consequence, researchers have sought more cost- effective improvements by building parallel computers that perform multiple operations in a single step. In order to solve a problem efficiently on a parallel machine, it is usually necessary to design an algorithm that specifies multiple operations on each step, i.e., a parallel algorithm. As an example, consider the problem of computing the sum of a sequence A of n numbers. The standard algorithm computes the sum by making a single pass through the sequence, keeping running sum of the numbers seen so far. It is not difficult however, to devise an algorithm for computing the sum that performs many operations in parallel. For example, suppose that, in parallel, each element of A with an even index is paired and summed with the next element of A, which has an odd index, i.e., A[0] is paired with A[1], A[2] with A[3], and so on. The result is a new sequence of [n/2] numbers that sum to the same value as the sum that is wish to compute. This pairing and summing Design & Implementation of Systolic Array Architecture 119 step can be repeated until, after [log2n] steps, a sequence consisting of single value is produced, and this value is equal to the final sum. The parallelism in an algorithm can yield improved performance on many deferent kinds of computers. For example, on a parallel computer, the operations in a parallel algorithm can be performed simultaneously by deferent processors. Furthermore, even on a single-processor computer the parallelism in an algorithm can be exploited by using multiple functional units, pipelined functional units, or pipelined memory systems. Thus, it is important to make a distinction between the parallelism in an algorithm and the ability of any particular computer to perform multiple operations in parallel. Of course, in order for a parallel algorithm to run efficiently on any type of computer, the algorithm must contain at least as much parallelism as the computer, for other-wise resources would be left idle. Unfortunately, the converse does not always hold: some parallel computers cannot efficiently execute all algorithms, even if the algorithms contain a great deal of parallelism. Experience has shown that it is more difficult to build a general-purpose parallel machine than a general-purpose sequential machine. OBJECTIVE(S) AND SCOPE Systolic array has been modelled in Verilog Hardware Description Language, which is small integral part of for full search block matching algorithm (FSBMA) for motion estimation and compensation [10-11], which leads to video sequence compression is realized using systolic array architectures. The objective of working on this project is to implement 2D systolic array in which 3*3 matrix multiplications can be performed. It is used in FSBMA. A large number of systolic array designs have been developed and used to perform a broad range of computations. In fact, recent advances in theory and software have allowed some of these systolic arrays to be derived automatically. The following is a representative list of computations for which systolic designs exist. Signal and Image Processing: Digital filters, convolution and correlation, discrete Fourier transform, fast Fourier transform (FFT--q.v.), encoding/ decoding for compression. Matrix Arithmetic: Matrix multiplication, solution of linear systems of equations, solution of Toeplitz linear systems, QR-decomposition, least-squares computation, singular value decomposition, eigenvalue computation, etc. Technology Used To model 2D systolic array matrix multiplication ModelSim is used for compilation and simulation. Leonardo Spectrum is used to obtain Synthesis Report, RTL implementation as well as to View Technology for Xilinx Virtex-II Pro FPGA, PROM & CPLD devices. METHODOLOGY The Systolic Array [1-4] is this design in an integral part of the main processor. The Systolic portion of the Processor is treated as an array of ALUs and it is controlled in very
Details
-
File Typepdf
-
Upload Time-
-
Content LanguagesEnglish
-
Upload UserAnonymous/Not logged-in
-
File Pages12 Page
-
File Size-