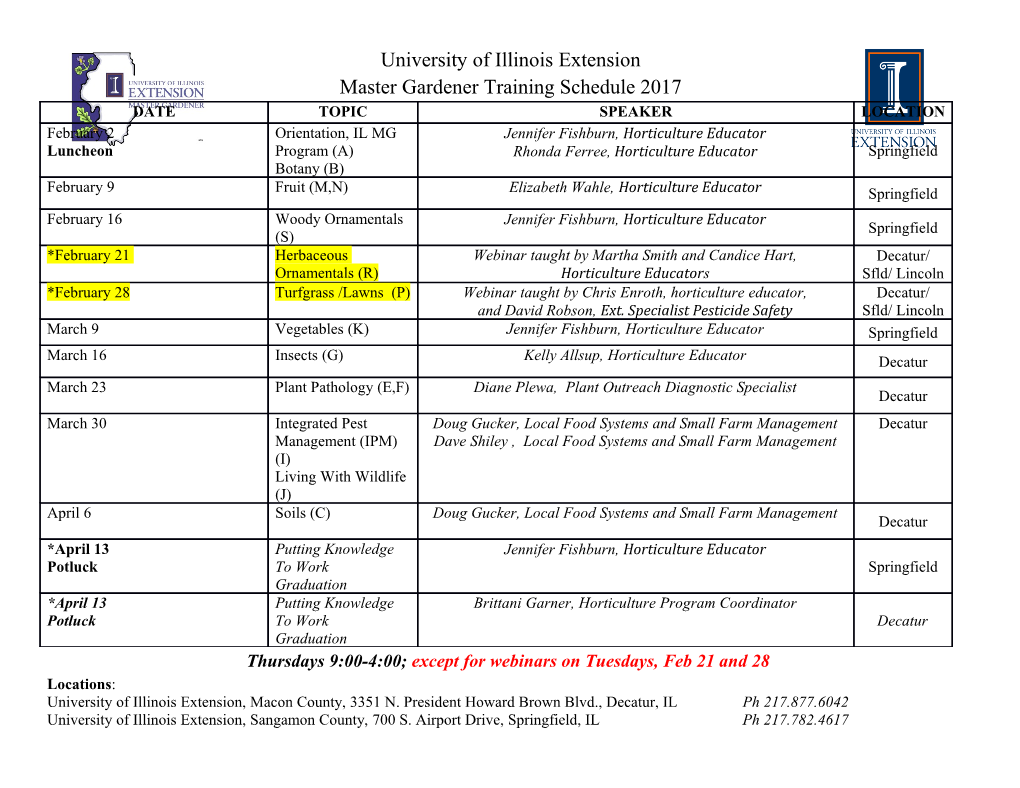
Copyright by Elad Liebman 2019 The Dissertation Committee for Elad Liebman certifies that this is the approved version of the following dissertation: Sequential Decision Making in Artificial Musical Intelligence Committee: Peter Stone, Supervisor Kristen Grauman Scott Niekum Maytal Saar-Tsechansky Roger B. Dannenberg Sequential Decision Making in Artificial Musical Intelligence by Elad Liebman Dissertation Presented to the Faculty of the Graduate School of The University of Texas at Austin in Partial Fulfillment of the Requirements for the Degree of Doctor of Philosophy The University of Texas at Austin May 2019 To my parents, Zipi and Itzhak, who made me the person I am today (for better or for worse), and to my children, Noam and Omer { you are the reason I get up in the morning (literally) Acknowledgments This thesis would not have been possible without the ongoing and unwavering support of my advisor Peter Stone, whose guidance, wisdom and kindness I am forever indebted to. Peter is the kind of advisor who would give you the freedom to work on almost anything, but would be as committed as you are to your research once you've found something you're passionate about. Patient and generous with his time and advice, gracious and respectful in times of disagreement, Peter has the uncanny gift of knowing to trust his students' instincts, but still continually ask the right questions. I could not have hoped for a better advisor. I also wish to thank my other dissertation committee members for their sage advice and useful comments: Roger Dannenberg, Kristen Grauman, Scott Niekum and Maytal Saar-Tsechansky. I must also give thanks to my colleague and friend Corey White, who not only collaborated with me extensively, but also served as my guide into the fields of cognitive modeling and the psychology of decision-making. Peter's academic patronage comes with the gigantic bonus of being part of the best research lab in the world (as far as I am concerned, at least), the UT Austin Learning Agents Research Group (LARG). My years at The University of Texas at Austin would have been a much poorer experience, academically and personally, without the wisdom, kindness, intelligence and companionship of my fellow labmates throughout the years: Michael Albert, Stefano Albrecht, Shani Alkoby, Samuel Barrett, Ishan Durugkar, Katie Genter, Josiah Hanna, Justin Hart, Matthew Hausknecht, Todd Hester, Yuqian Jiang, Piyush Khandelwal, Matteo Leonetti, Shih-Yun Lo, Patrick Macalpine, Jake Menashe, Sanmit Narvekar, Guni Sharon, Jivko Sinapov, Faraz Torabi, Daniel Urieli, Garrett Warnell, Harel Yedidsion, and Shiqi Zhang. I am particularly thankful to Ishan Durugkar, Piyush Khandelwal and Patrick Macalpine, with whom I had the pleasure of collaborating extensively. During my years in the PhD program I have also been fortunate enough to be a member of the UT Austin Villa Standard Platform League RoboCup team. I have greatly enjoyed working in our shared codebase over the years. Being involved in RoboCup has led me to countless mean- v ingful insights, professional and otherwise, and I've also learned valuable lessons about teamwork, performing under stress, and the immutability of real-world deadlines. I would like to thank my M.Sc. advisor, Prof. Benny Chor, for steering me down this treacherous, winding, but ultimately fruitful path. Had Benny not cajoled me into pursuing a graduate degree in computer science, the course of my life might have been completely altered. I will forever treasure his mentorship (and his kindness, and his whip-sharp sense of humor) as I was making my initial steps in academia. Lastly, I would like to thank my family - my parents, Zipi and Itzhak, who supported me in every way possible throughout my life; my sister, Shira, who's always been there for me; my wife, Meital who went on this insane journey with me (hey Meital, we survived!); and my close friends, Jonathan Mueller and Moran Aharoni, for their staunch friendship and support through thick and thin. I would thank my children, Noam and Omer, who certainly did not help me work on my dissertation (quite the opposite), but did feel every waking moment in my life with a sense of determination and purpose. If I have accomplished anything, I have all of you to thank for it. Elad Liebman The University of Texas at Austin May 2019 vi Sequential Decision Making in Artificial Musical Intelligence Publication No. Elad Liebman, Ph.D. The University of Texas at Austin, 2019 Supervisor: Peter Stone Over the past 60 years, artificial intelligence has grown from a largely academic field of research to a ubiquitous array of tools and approaches used in everyday technology. Despite its many recent successes and growing prevalence, certain meaningful facets of computational intelligence have not been as thoroughly explored. Such additional facets cover a wide array of complex mental tasks which humans carry out easily, yet are difficult for computers to mimic. A prime example of a domain in which human intelligence thrives, but machine understanding is still fairly limited, is music. Over the last decade, many researchers have applied computational tools to carry out tasks such as genre identification, music summarization, music database querying, and melodic segmen- tation. While these are all useful algorithmic solutions, we are still a long way from constructing complete music agents, able to mimic (at least partially) the complexity with which humans ap- proach music. One key aspect which hasn't been sufficiently studied is that of sequential decision making in musical intelligence. This thesis strives to answer the following question: Can a sequential decision making perspective guide us in the creation of better music agents, and social vii agents in general? And if so, how? More specifically, this thesis focuses on two aspects of musical intelligence: music recommendation and human-agent (and more generally agent-agent) interaction in the context of music. The key contributions of this thesis are the design of better music playlist recommendation algorithms; the design of algorithms for tracking user preferences over time; new approaches for modeling people's behavior in situations that involve music; and the design of agents capable of meaningful interaction with humans and other agents in a setting where music plays a roll (either directly or indirectly). Though motivated primarily by music-related tasks, and focusing largely on people's musical preferences, this thesis also establishes that insights from music-specific case studies can also be applicable in other concrete social domains, such as different types of content recommendation. Showing the generality of insights from musical data in other contexts serves as evidence for the utility of music domains as testbeds for the development of general artificial intelligence techniques. Ultimately, this thesis demonstrates the overall usefulness of taking a sequential decision making approach in settings previously unexplored from this perspective. viii Contents Acknowledgments v Abstract vii List of Tables xiv List of Figures xv 1 Introduction 1 1.1 Research Question and Contributions..........................2 1.2 Thesis Structure......................................5 2 Background 8 2.1 Reinforcement Learning..................................8 2.2 AI, Music and Agents................................... 10 2.3 Music and Human Behavior................................ 11 2.4 Summary.......................................... 11 3 Playlist Recommendation 12 3.1 Music Playlist Recommendation as an MDP....................... 13 3.2 Modeling.......................................... 15 3.2.1 Modeling Songs................................... 15 3.2.2 Modeling The Listener Reward Function..................... 16 3.2.3 Expressiveness of the Listener Model....................... 17 3.3 Data............................................. 17 3.4 DJ-MC........................................... 19 3.4.1 Learning Initial Song Preferences......................... 20 3.4.2 Learning Initial Transition Preferences...................... 20 3.4.3 Learning on the Fly................................ 21 3.4.4 Planning...................................... 22 3.5 Evaluation in Simulation.................................. 23 ix 3.5.1 Performance of DJ-MC with Feature Dependent Listeners........... 26 3.5.2 A Feature-Dependent DJ-MC........................... 27 3.6 Evaluation on Human Listeners.............................. 29 3.6.1 Experimental Setup................................ 31 3.7 Planning Extensions To DJ-MC.............................. 33 3.7.1 Upper Confidence Bound in Trees (UCT).................... 33 3.8 UCT for Playlist Generation................................ 35 3.9 Planning for Personalization................................ 37 3.10 Planning for Diversity................................... 37 3.11 Summary.......................................... 40 4 Algorithms for Tracking Changes In Preference Distributions 41 4.1 Model Retraining as a Markov Decision Process..................... 43 4.1.1 Markov Decision Processes............................ 44 4.1.2 Formulation of the Model Retraining Problem.................. 44 4.2 Learning a Policy through Approximate Value Iteration................ 45 4.3 Theoretical Intuition.................................... 47 4.4 Distribution Model Retraining.............................. 47 4.4.1 MDP Representation for the Distribution Tracking Problem.......... 48 4.4.2 AVI for Distribution Model Retraining.....................
Details
-
File Typepdf
-
Upload Time-
-
Content LanguagesEnglish
-
Upload UserAnonymous/Not logged-in
-
File Pages237 Page
-
File Size-