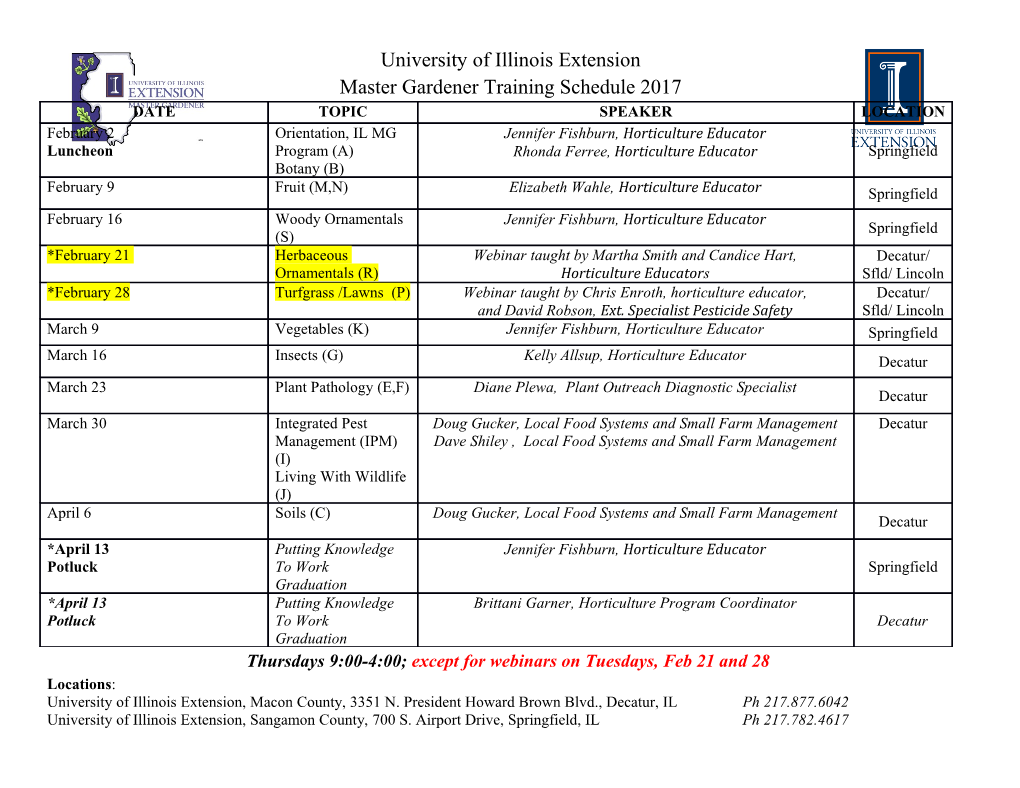
LITERATURE MINING SUSTAINS AND ENHANCES KNOWLEDGE DISCOVERY FROM OMIC STUDIES by Rick Matthew Jordan B.S. Biology, University of Pittsburgh, 1996 M.S. Molecular Biology/Biotechnology, East Carolina University, 2001 M.S. Biomedical Informatics, University of Pittsburgh, 2005 Submitted to the Graduate Faculty of School of Medicine in partial fulfillment of the requirements for the degree of Doctor of Philosophy University of Pittsburgh 2016 UNIVERSITY OF PITTSBURGH SCHOOL OF MEDICINE This dissertation was presented by Rick Matthew Jordan It was defended on December 2, 2015 and approved by Shyam Visweswaran, M.D., Ph.D., Associate Professor Rebecca Jacobson, M.D., M.S., Professor Songjian Lu, Ph.D., Assistant Professor Dissertation Advisor: Vanathi Gopalakrishnan, Ph.D., Associate Professor ii Copyright © by Rick Matthew Jordan 2016 iii LITERATURE MINING SUSTAINS AND ENHANCES KNOWLEDGE DISCOVERY FROM OMIC STUDIES Rick Matthew Jordan, M.S. University of Pittsburgh, 2016 Genomic, proteomic and other experimentally generated data from studies of biological systems aiming to discover disease biomarkers are currently analyzed without sufficient supporting evidence from the literature due to complexities associated with automated processing. Extracting prior knowledge about markers associated with biological sample types and disease states from the literature is tedious, and little research has been performed to understand how to use this knowledge to inform the generation of classification models from ‘omic’ data. Using pathway analysis methods to better understand the underlying biology of complex diseases such as breast and lung cancers is state-of-the-art. However, the problem of how to combine literature- mining evidence with pathway analysis evidence is an open problem in biomedical informatics research. This dissertation presents a novel semi-automated framework, named Knowledge Enhanced Data Analysis (KEDA), which incorporates the following components: 1) literature mining of text; 2) classification modeling; and 3) pathway analysis. This framework aids iv researchers in assigning literature-mining-based prior knowledge values to genes and proteins associated with disease biology. It incorporates prior knowledge into the modeling of experimental datasets, enriching the development process with current findings from the scientific community. New knowledge is presented in the form of lists of known disease-specific biomarkers and their accompanying scores obtained through literature mining of millions of lung and breast cancer abstracts. These scores can subsequently be used as prior knowledge values in Bayesian modeling and pathway analysis. Ranked, newly discovered biomarker-disease-biofluid relationships which identify biomarker specificity across biofluids are presented. A novel method of identifying biomarker relationships is discussed that examines the attributes from the best- performing models. Pathway analysis results from the addition of prior information, ultimately lead to more robust evidence for pathway involvement in diseases of interest based on statistically significant standard measures of impact factor and p-values. The outcome of implementing the KEDA framework is enhanced modeling and pathway analysis findings. Enhanced knowledge discovery analysis leads to new disease-specific entities and relationships that otherwise would not have been identified. Increased disease understanding, as well as identification of biomarkers for disease diagnosis, treatment, or therapy targets should ultimately lead to validation and clinical implementation. v TABLE OF CONTENTS ACKNOWLEDGEMENTS ..................................................................................................... xiv GLOSSARY ……………………………………………………………………..…………….xvi PREFACE ……………………………………………………………………………………....xx 1.0 INTRODUCTION ………………..……………………….………………….......…….1 1.1 THE PROBLEM ……….………………………………………………...……....4 1.1.1 Obtaining and organizing prior information from literature mining .5 1.1.2 Combining prior knowledge with experimental data ………..…..……7 1.1.3 Interpreting downstream results .…….…..….………………………….8 1.2 THE APPROACH …....…..………………………………………………………9 1.2.1 Theses ...…................……………………………………………………12 1.3 SIGNIFICANCE …………............……………………………………………...13 1.4 DISSERTATION OVERVIEW …….....……………………………………..…14 2.0 BACKGROUND ………...........……………........……………………………………15 2.1 MOLECULAR ASPECTS OF LUNG AND BREAST CANCER ….........…..15 2.2 LITERATURE MINING ……….....……..………………..……………………25 2.3 CLASSIFICATION MODELING.……...……....................………..………….34 2.3.1 Bayesian analysis …….……………….....……………………...………..35 2.4 PATHWAY ANALYSIS …..…….......……………………………………….....41 2.5 PRIOR KNOWLEDGE USE IN BIOINFORMATICS …….…......…………46 vi 3.0 IMPLEMENTATION OF KEDA FRAMEWORK …....…………………………..65 3.1 KEDA FRAMEWORK OVERVIEW...…………………..………...……………65 3.2 DESCRIPTION OF KEDA COMPONENTS ………………...…………………66 3.2.1 Literature mining methodology …………….……….…...…………...66 3.2.1.1 Information retrieval …………………………......….…….67 3.2.1.2 Named entity recognition ………………………......…..….71 3.2.1.3 Entity extraction ………...………………...……………….72 3.2.1.4 Dictionary ………………...………...………………………72 3.2.1.5 Z-score calculation……………...……......………………....72 3.2.1.6 Verification of relationships ……………………….......…..75 3.2.1.7 Error rate determination ………………………...…….…..78 3.2.2 Classification modeling methodology ……………….…………….......78 3.2.2.1 Experimental datasets …………………...………………....79 3.2.2.2 Preprocessing steps ……………….……..………….……....92 3.2.2.3 Transforming counts to prior probabilities …...……...…..96 3.2.2.4 Bayesian Rule Learner ………………...…………...………96 3.2.2.5 Execution of the BRL algorithm ………..……………........99 3.2.2.5.1 BRL input ……….………………...……..……..…99 3.2.2.5.2 BRL output ……….………...………….…...........100 3.2.2.6 Confirmatory research ……………..……………...….…..101 3.2.3 Pathway analysis methodology ………………..……….………..........101 3.2.3.1 Pathway Express…………………………..…………..…...102 3.2.3.2 Execution of Pathway Express algorithm…..…..….....…..103 vii 3.2.3.2.1 Pathway Express input ……....……….…..…….103 3.2.3.2.2 Pathway Express output …………...…...........…104 4.0 EVALUATION OF KEDA ……………..…………….............…...........….....……..107 4.1 LITERATURE MINING RESULTS …………..……….…..……….……......107 4.1.1 Z-score threshold optimization .………………………………….......108 4.1.2 Known markers per biofluid ……………………………………........109 4.1.3 Known markers found significant vs. non-significant ……………...110 4.1.4 Newly discovered markers found significant vs. non-significant ......111 4.1.5 Potential marker biofluid specificity ………………………………...112 4.1.6 Manual verification of findings ………………………...………….....115 4.1.7 Error rate estimation of new discoveries ………...…….……...…......115 4.2 CLASSIFICATION MODELING RESULTS ……………...………………....117 4.2.1 Experimental design using literature mining results ……….………117 4.2.2 Dataset size effects on accuracy ………………………..……...…......118 4.2.3 Weighting effects on accuracy…………………………...………..…119 4.2.4 Accuracy across different types of data ...……...…...…...…......120 4.2.5 Statistical significance ....………..……………………………...…..122 4.2.6 Examining modeling attributes used ……………………......…122 4.2.7 Confirmatory research …………….……………………….……..........123 4.3 PATHWAY ANALYSIS RESULTS ………...…………………………..….....127 4.3.1 Experimental design using literature mining results ……………….128 4.3.2 Breast cancer datasets ………………………………………………...129 4.3.3 Lung cancer datasets ……………………………………………….....136 viii 4.4 SUMMARY …………..…....……………..………………………....………...142 5.0 CONCLUSIONS, LIMITATIONS, AND FUTURE WORK ……...……...……..144 5.1 CONCLUSIONS ……………………………………………….……………...144 5.2 LIMITATIONS ……………………………………………….…………...…..146 5.3 FUTURE WORK ………..……………………………..…………….……..…147 5.3.1 Informatics …………………………………………………………….147 5.3.1.1 Resources …………………….……...……….…..………...147 5.3.1.2 Tools …...............………………………………………...…148 5.3.1.2.1 Literature mining ……..……………....………..148 5.3.1.2.2 Modeling …….…………….............…….....……149 5.3.1.2.3 Pathway analysis….…........................................ 150 5.3.2 Laboratory verification ...………………………………………….....150 APPENDIX A - PYTHON SCRIPT FOR KEDA TEXT-MINING COMPONENT …….152 APPENDIX B – INPUT DATASET DESCRIPTIONS FROM GEO ………………..……159 APPENDIX C - BIOMARKERS FOUND BY KEDA TEXT-MINING AND MODELING COMPONENTS………………………..........……………………………...170 BIBLIOGRAPHY …………………………………………………………………………….201 ix LIST OF TABLES Table 1. Pathway analysis tools ……………………………………………………………….....42 Table 2. Size of the abstract sets returned from breast and lung cancer PubMed queries ……….69 Table 3. Breast cancer-related genes from text-mining final table …………………………........74 Table 4. Known Breast Cancer Biomarkers ……………………………………………………..76 Table 5. Known Lung Cancer Biomarkers ……………………………………………………...77 Table 6. Breast and lung cancer dataset summary…………...………………...…………..…….82 Table 7. Input files for matching script ………………………………………………………….95 Table 8. Matching script output files ……………………………………………………………95 Table 9. Number of markers identified for each disease-biofluid combination …………...…..111 Table 10. Identification of the significant validated potential markers found to be in common to several biofluids or biofluid specific for breast and lung cancer………...113 Table 11. Manually verified biomarker table …………………,………………………………116 Table 12. Number of input genes in pathway from breast cancer datasets ………….....……....130 Table 13. Impact factors from breast cancer datasets …………………………………….........134 Table 14. P-values from breast cancer datasets
Details
-
File Typepdf
-
Upload Time-
-
Content LanguagesEnglish
-
Upload UserAnonymous/Not logged-in
-
File Pages249 Page
-
File Size-