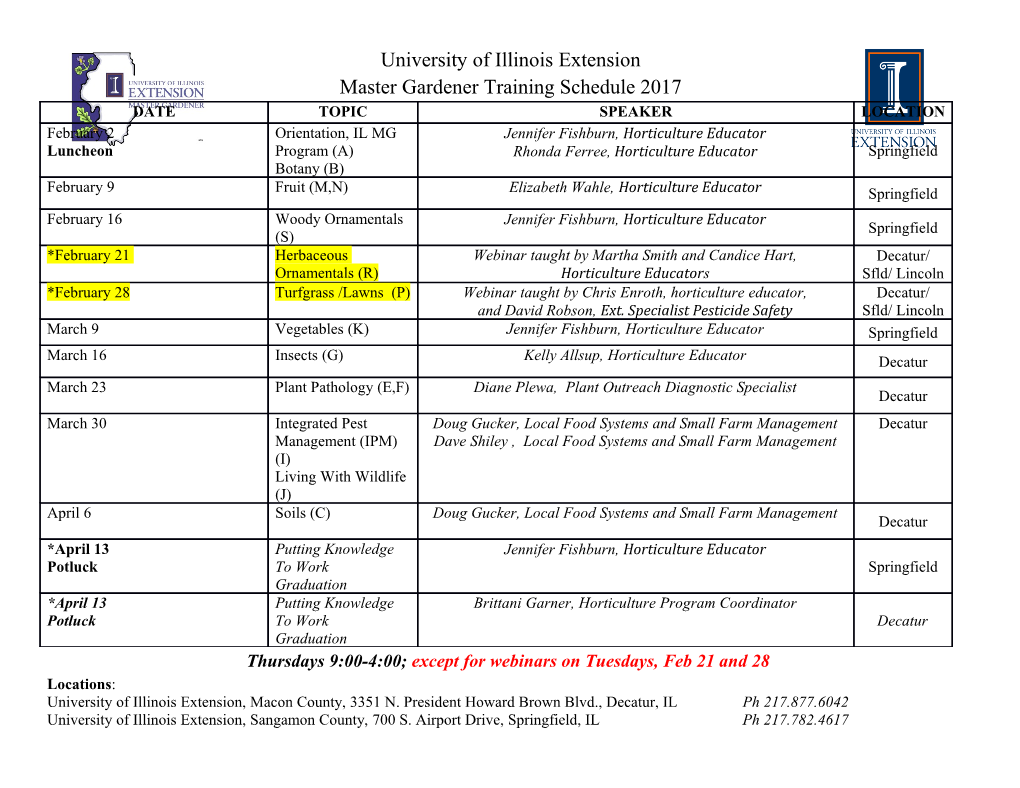
View metadata, citation and similar papers at core.ac.uk brought to you by CORE provided by Newcastle University eTheses Multivariate Statistical Process Monitoring Using Classical Multidimensional Scaling Prepared by: Mohd Yusri Mohd Yunus A Thesis submitted in partial fulfillment of the requirements for the degree of Doctor of Philosophy School of Chemical Engineering and Advanced Materials Newcastle University UK May 2012 ABSTRACT A new Multivariate Statistical Process Monitoring (MSPM) system, which comprises of three main frameworks, is proposed where the system utilizes Classical Multidimensional Scaling (CMDS) as the main multivariate data compression technique instead of using the linear- based Principal Component Analysis (PCA). The conventional method which usually applies variance-covariance or correlation measure in developing the multivariate scores is found to be inappropriately used especially in modelling nonlinear processes, where a high number of principal components will be typically required. Alternatively, the proposed method utilizes the inter-dissimilarity scales in describing the relationships among the monitored variables instead of variance-covariance measure for the multivariate scores development. However, the scores are plotted in terms of variable structure, thus providing different formulation of statistics for monitoring. Nonetheless, the proposed statistics still correspond to the conceptual objective of Hotelling’s T2 and Squared Prediction Errors (SPE). The first framework corresponds to the original CMDS framework, whereas the second utilizes Procrustes Analysis (PA) functions which is analogous to the concept of loading factors in PCA for score projection. Lastly, the final framework employs dynamic mechanism of PA functions as an alternative for enhancing the procedures of the second approach. A simulated system of Continuous Stirred Tank Reactor with Recycle (CSTRwR) has been chosen for the demonstration and the fault detection results were comparatively analyzed to the outcomes of PCA on the grounds of false alarm rates, total number of detected cases and also total number of fastest detection cases. The last two performance factors are obtained through fault detection time. The overall outcomes show that the three CMDS-based systems give almost comparable performances to the linear PCA based monitoring systemwhen dealing the abrupt fault events, whereas the new systems have demonstrated significant improvement over the conventional method in detecting incipient fault cases. More importantly, this monitoring accomplishment can be efficiently executed based on lower compressed dimensional space compared to the PCA technique, thus providing much simpler solution. All of these evidences verified that the proposed approaches are successfully developed conceptually as well as practically for monitoring while complying fundamentally with the principles and technical steps of the conventional MSPM system. ii ACKNOWLEDGEMENT First of all, I would like to thank to both of my respectful supervisors Dr. Jie Zhang and Professor Julian Morris who have relentlessly provided me the guidance, constructive ideas and invaluable advice, enabling me to achieve the objective of this thesis. I am also very grateful to my parents, my wife, my daughter and all of my family members for their endless inspiration, kindness, understanding as well as patience. I am also abundantly indebted to many people, particularly in SAGE as well as SCEAM of Newcastle University, UK, and also Universiti Malaysia Pahang (UMP) as well as Ministry of Higher Educational Malaysia (MoHE), whom have helped me in various ways, to start, continue and complete the study. May God bless us all. “ The goal of the journey is the journey itself...poetically put, it is a journey that takes us far away, and back to ourselves...Humility is my table, respect is my garment, empathy is my food and curiosity is my drink. As for love, it has a thousand names and is by my side at every window.” (Tariq Ramadan, The Quest For Meaning, 2010). iii TABLE OF CONTENTS CHAPTERS TITLES PAGES TOPIC PAGE i ABSTRACT ii ACKNOWLEDGEMENT iii TABLE OF CONTENTS iv LIST OF TABLES viii LIST OF FIGURES x LIST OF ABBREVIATIONS xvi LIST OF SYMBOLS xviii CHAPTER 1 INTRODUCTION 1 1.1 Background 1 1.2 Motivation 4 1.3 Aim and Objectives 8 1.4 Scopes 9 1.5 Contributions 10 1.6 Thesis Organization 12 CHAPTER 2 MULTIVARIATE STATISTICAL PROCESS MONITORING 14 2.1 Introduction 14 2.2 Fundamentals of Conventional MSPM System 15 2.2.1 Phase I: Off-line Modelling and Monitoring 17 iv 2.2.2 Phase II: On-line Monitoring 23 2.3. MSPM Issues and Extensions 27 2.3.1 Issue I: Monitoring Statistics 28 2.3.2 Issue 2: Monitoring Limits 28 2.3.3 Issue 3: Multivariate techniques 30 2.3.4 Issue 4: Fault Diagnosis 36 2.3.5 Issue 5: Data Formulation 38 2.3.6 Issue 6: Automation System 39 2.4. MDS as An Alternative Solution for MSPM System 39 2.4.1 Conceptual Background of MDS 40 2.4.2 Mathematical Fundamentals of MDS 44 2.4.3 Connections between PCA and MDS 50 2.4.4 Previous Works on MDS-based Monitoring Systems 52 2.4.5 Justification of Applying MDS in The MSPM Framework 54 2.5 Summary 55 CHAPTER 3 CASE STUDIES AND PCA-BASED MONITORING PERFORMANCES 56 3.1 Introduction 56 3.2 CSTRwR System 56 3.2.1 Process Descriptions 56 3.2.2 NOC Samples and PCA Monitoring Performances 57 3.2.3 Fault Cases PCA Monitoring Performances 61 3.3 Summary 71 CHAPTER 4 FRAMEWORK I: MDS-BASED MSPM SYSTEM USING MOVING WINDOW CMDS PROJECTION 72 4.1 Introduction 72 4.2 Methodology 72 4.2.1 Phase I Procedures 73 4.2.2 Phase II Procedures 77 v 4.3 Results and Analysis 79 4.4 Results Discussion 100 4.4.1 The Impact of Dissimilarity Measures on The Monitoring Outcomes 100 4.4.2 The Impact of using New Monitoring Statistics on The Monitoring Outcomes 100 4.4.3 The Impact of Applying Various Window Settings on The Monitoring Outcomes 101 4.4.4 The Impact of Applying Smaller Dimensionalities on The Monitoring Outcomes 102 4.5 Summary 103 CHAPTER 5 FRAMEWORK II: MDS-BASED MSPM SYSTEM USING MOVING WINDOW CMDS PROCRUSTES ANALYSIS PROJECTION 104 5.1 Introduction 104 5.2 Methodology 105 5.2.1 Phase I Procedures 105 5.2.2 Phase II Procedures 109 5.3 Results and Analysis 111 5.4 Results Discussion 132 5.4.1 The Impact of Dissimilarity Measures on The Monitoring Outcomes 132 5.4.2 The Impact of using New Monitoring Statistics on The Monitoring Outcomes 132 5.4.3 The Impact of Applying Various Window Settings on The Monitoring Outcomes 133 5.4.4 The Impact of Applying Smaller Dimensionalities on The Monitoring Outcomes 133 vi 5.5 Summary 134 CHAPTER 6 FRAMEWORK III: MDS-BASED MSPM SYSTEM USING MOVING WINDOW CMDS DYNAMICAL PROCRUSTES ANALYSIS PROJECTION 135 6.1 Introduction 135 6.2 Methodology 136 6.2.1 Phase I Procedures 137 6.2.2 Phase II Procedures 138 6.3 Results and Analysis 141 6.4 Results Discussion 154 6.4.1 The Impact of Dissimilarity Measures on The Monitoring Outcomes 154 6.4.2 The Impact of using New Monitoring Statistics on The Monitoring Outcomes 154 6.4.3 The Impact of Applying Various Window Settings on The Monitoring Outcomes 155 6.4.4 The Impact of Applying Smaller Dimensionalities on The Monitoring Outcomes 156 6.5 Summary 157 CHAPTER 7 CONCLUSIONS AND RECOMMENDATIONS 158 7.1 Conclusions 158 7.2 Recommendations for further works 162 REFERENCES 164 APPENDIX A 172 APPENDIX B 173 APPENDIX C 176 vii LIST OF TABLES TABLE NO. TITLES PAGES Table 2.1 Examples of industrial applications of MSPM 27 Table 2.2 Categories of MSPM Issues and Extensions Reviewed in This Study 27 Table 2.3 Extensions of PCA-based monitoring techniques 31 Table 2.4 Dissimilarity matrix based on Euclidean scales used in Figure 2.2 48 Table 3.1 List of variables in the CSTRwR system for monitoring 57 Table 3.2 List of abnormal operations in CSTRwR 61 Table 4.1 Fault detection times of monitoring systems based on sCMDS and PCA for abrupt fault cases 82 Table 4.2 Fault detection times of monitoring systems based on sCMDS and PCA for incipient fault cases 83 Table 5.1 Fault detections times of monitoring systems based on CMDS-PA and PCA for abrupt fault cases 113 Table 5.2 Fault detection times of monitoring systems based on CMDS-PA and PCA for incipient fault cases 114 Table 5.3 Monitoring limits specified for Sr based on sCMDS and CMDS-PA methods 132 Table 6.1 Results of FDT of abrupt fault cases based on CMDS-dPA for CSTRwR 142 Table 6.2 Results of FDT of incipient fault cases based on CMDS-dPA for CSTRwR 143 viii Table 6.3 Monitoring limits specified for Sm2 based on Euclidean scale and window size 5 154 Table 6.3 Monitoring limits specified for Sr based on Euclidean scale and window size 5 155 ix LIST OF FIGURES FIGURE NO. TITLES PAGES Figure 2.1 Conventional MSPM framework 17 Figure 2.2 A numerical example for CMDS 47 Figure 2.3 The reproduction of CMDS scores that demonstrated in Figure 2.2 48 Figure 3.1 CSTRwR system 57 Figure 3.2 Accumulated data variations explained by different PCs for the CSTRwR 58 Figure 3.3 Progressions of T2 (left) and SPE (right) of PCA models with 3 PCs (top), 5 PCs (middle), and 7 PCs (bottom) on the original NOC data 59 Figure 3.4 Progressions of T2 (left) and SPE (right) of PCA models with 3 PCs (top), 5 PCs (middle), and 7 PCs (bottom) on the testing NOC data 60 Figure 3.5 The behaviours of fault number 6 based on trends of variables 1 (top charts), 7 (middle charts) and 13 (bottom charts) 62 Figure 3.6 Progressions of T2 (left) and SPE (right) of PCA models with 3 PCs (top), 5 PCs (middle), and 7 PCs (bottom) for F6a
Details
-
File Typepdf
-
Upload Time-
-
Content LanguagesEnglish
-
Upload UserAnonymous/Not logged-in
-
File Pages199 Page
-
File Size-