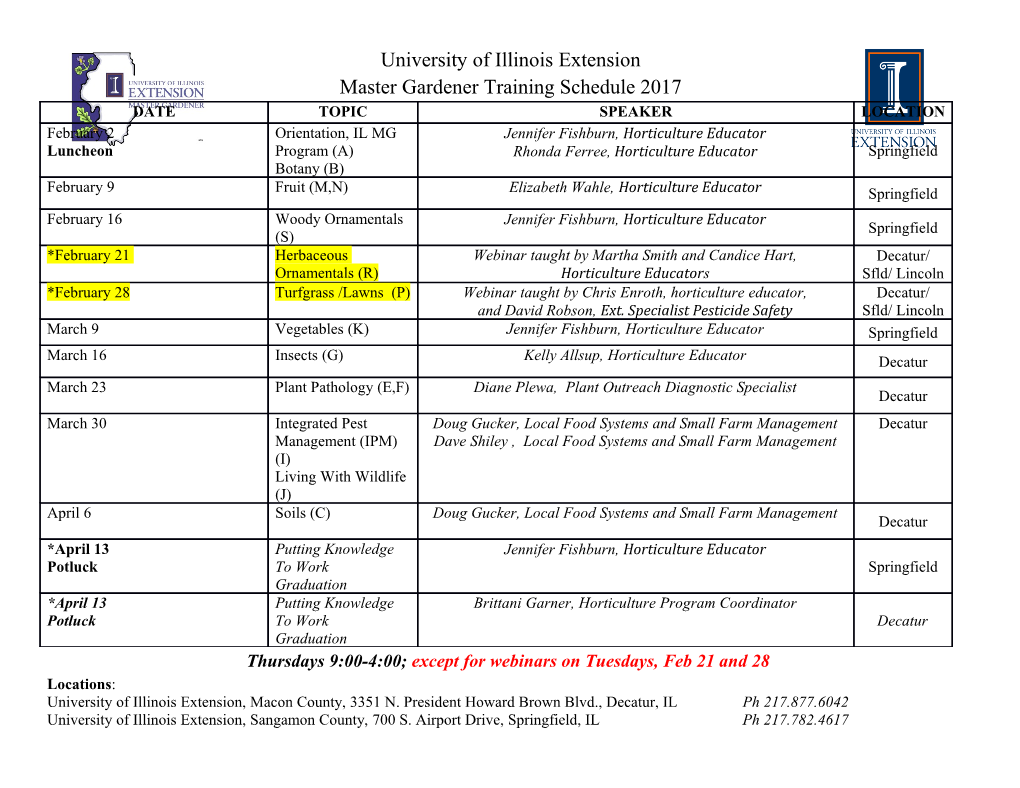
Research Statement Bill Fefferman, University of Maryland/NIST Since the discovery of Shor's algorithm in the mid 1990's, it has been known that quan- tum computers can efficiently solve integer factorization, a problem of great practical relevance with no known efficient classical algorithm [1]. The importance of this result is impossible to overstate: the conjectured intractability of the factoring problem provides the basis for the se- curity of the modern internet. However, it may still be a few decades before we build universal quantum computers capable of running Shor's algorithm to factor integers of cryptographically relevant size. In addition, we have little complexity theoretic evidence that factoring is com- putationally hard. Consequently, Shor's algorithm can only be seen as the first step toward understanding the power of quantum computation, which has become one of the primary goals of theoretical computer science. My research focuses not only on understanding the power of quantum computers of the indefinite future, but also on the desire to develop the foundations of computational complexity to rigorously analyze the capabilities and limitations of present-day and near-term quantum devices which are not yet fully scalable quantum computers. Furthermore, I am interested in using these capabilities and limitations to better understand the potential for cryptography in a fundamentally quantum mechanical world. 1 Comparing quantum and classical nondeterministic computation Starting with the foundational paper of Bernstein and Vazirani it has been conjectured that quantum computers are capable of solving problems whose solutions cannot be found, or even verified efficiently on a classical computer [2]. In particular, they conjecture the existence of an oracle problem solvable by efficient quantum computation that cannot be solved in NP, or even more generally, by the Polynomial-time Hierarchy, or PH. This has since become one of the most important and central questions in quantum complexity theory (see e.g., [3,4,5]). We have developed the only currently feasible route toward validating this con- jecture via a novel connection between quantum algorithms and classical pseu- dorandom generators [6,7]. These are classical algorithms that take a small number of uniformly random input bits and produce a much longer output that cannot be distinguished from uniform with a classical computer. We show that the output distribution of a particular instantiation of the ubiquitous Nisan-Wigderson pseudorandom generator [8] can be distin- guished from the uniform distribution with a quantum computer. Likewise, we prove that these distributions cannot be distinguished by any NP algorithm. Proving that this distribution can- not be distinguished from uniform by any PH algorithm would fully confirm the conjecture of Bernstein and Vazirani, and has been a major goal in my research. In addition to the obvious quantum motivation, we have discovered new connections between attaining this separation and the development of pseudorandom generators with stronger parameters and new methods for proving classical circuit lower bounds (see [7]). 2 Quantum advantage from sampling experiments An exciting line of work has established the existence of distributions samplable by quantum computers that cannot be sampled exactly by randomized classical computation, under mild hardness assumptions [9, 10]. Crucially, these results hold even for restrictive quantum samplers that are not universal quantum computers. As such, it is plausible that present-day laboratory devices are already capable of harnessing quantum effects to demonstrate computational tasks that are beyond the reach of even the fastest classical computers. In the popular BosonSampling proposal, for example, such a sampling task is achieved by producing single photons, sending them through a network of beamsplitters and phase shifters, and performing photon-number resolving measurements [11]. A number of experimental groups have now performed demonstrations of small-scale BosonSampling experiments [12, 13, 14, 15, 16, 17]. However, the theory has not completely caught up with the experiment. While the photonic platform for quantum information processing has much in its favor, it suffers from imperfect detector efficiency and photon loss which gets worse as the number of optical components is scaled up. It is not understood whether the hardness-of-sampling proofs can be generalized to apply to experimentally realistic parameter regimes (see e.g., [11, 18, 19, 20, 21]). To model experimental error, one would at least want an \approximate hardness" result ruling out efficient classical sampling from any distribution close in total variation distance to the ideal quantum distribution. In the case of BosonSampling, hardness of approximate sampling can only be obtained under unproven complexity theoretic hardness assumptions about the hardness of approximating the Permanent. My interest in this line of work is multifaceted. I would like to understand the minimal hard- ness assumption needed to obtain strong approximate sampling results. We showed that a very simple quantum algorithm that applies some classical computation in quantum superposition followed by a quantum Fourier transform is capable of sampling from distributions that cannot be sampled approximately classically, under any of a broad array of hardness assumptions that formally weakens those needed by BosonSampling [22]. This result provides the strongest provable evidence for the superiority of quantum computation under some of the weakest assumptions. In future work, I hope to use surprising similarities between this quan- tum Fourier sampling distribution and the BosonSampling distribution to develop new methods for the verification of BosonSampling experiments. Thinking about these sampling results led me to consider alterative physical systems ca- pable of outperforming classical computation. Very recently, we showed that sampling from the output distribution of a different experimentally realizable quantum system (namely, an Ising model with no transverse field), is hard classically [23]. This result has already had the- oretical implications concerning the complexity theoretic classification of two qubit commuting Hamiltonians [24]. 3 Characterizing the power of quantum computation with restricted re- sources Long before we see fully scalable quantum computers, we will have quantum devices with restricted resources. Theoretical models motivated by the limitations of real-world experiments involve restricting the standard quantum circuit model either in time (gate count), in space (number of qubits) or in depth. Ta-Shma gave the first evidence that quantum computers could attain a quadratic advantage in space usage over classical computation, which is known to be the maximum possible [25, 26]. In particular, he demonstrated an algorithm for \well-conditioned" matrix1 inversion, that runs in quantum O(log n)-space. Quantum algorithms for matrix inversion are of direct interest due to their many applications in scientific computing. Recent work has investigated applications of matrix inversion to prob- lems ranging from machine learning [27], to calculation of radar scattering crossections [28]. The performance advantages of these quantum algorithms over classical computation are not well understood [29], a situation that could be improved by research into the complexity-theoretic foundations of space-limited quantum computation. We recently gave another reason to care about the matrix inversion problem: it completely characterizes quantum space complexity. In particular we show that a 1A well-conditioned n × n matrix is one in which the condition number, or ratio between largest to smallest singular value, is bounded by a polynomial in n. suitably parameterized matrix inversion problem is the hardest problem solvable by unitary quantum circuits that act on a bounded number of qubits. To do this, we improve on Ta- Shma's result both by giving a new algorithm for space efficient matrix inversion (avoiding the need for intermediate measurements) and by showing a matching hardness result [30, 31, 32]. This characterization is interesting, as the ability to invert certain other classes of matrices has been shown to be equivalent in power to quantum computation with bounded time [33]. Consequently, one can use the matrix inversion problem to give a direct comparison of the power of space-bounded quantum computations to time-bounded quantum computations, and explore how these resources trade off. I am very interested in using these results, possibly together with results about quantum depth vs time and space tradeoffs (see e.g., [34, 35]), to characterize the power of quantum computation with restricted depth. The desire to analyze the power of quantum depth is moti- vated experimentally, where the effects of decoherence make reliably implementing a quantum circuit with more than a small number of layers very challenging (see e.g., [35, 36]). It is also motivated theoretically, where it is known that Shor's algorithm can be solved with efficient polylogarithmic depth quantum circuits supplemented by classical computation [37]. Can all quantum algorithms be simulated in this way? Understanding this question has long been cited as a major challenge in quantum complexity theory (see e.g., [36, 38]). Finally, I am interested in developing new error amplification protocols for these restric- tive forms of quantum computation. We recently gave the first method for
Details
-
File Typepdf
-
Upload Time-
-
Content LanguagesEnglish
-
Upload UserAnonymous/Not logged-in
-
File Pages8 Page
-
File Size-