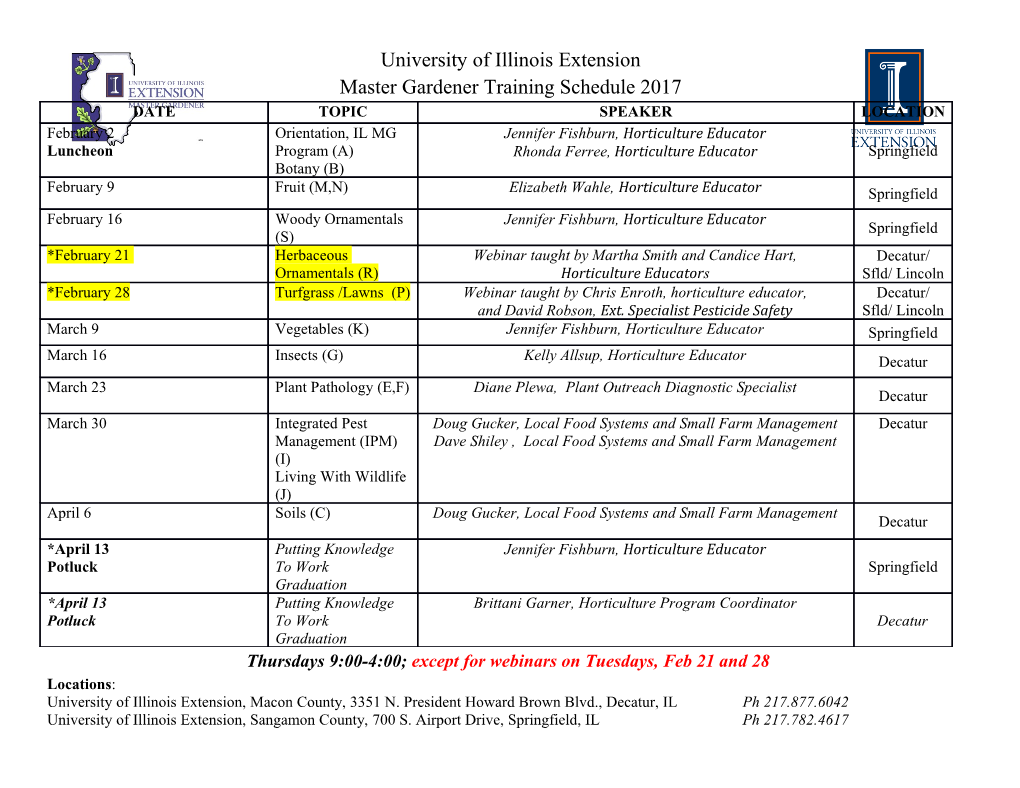
An Event-related fMRI Study of Artificial Grammar Learning in a Balanced Chunk Strength Design Matthew D. Lieberman1, Grace Y. Chang1, Joan Chiao2, Susan Y. Bookheimer1, and Barbara J. Knowlton1 Downloaded from http://mitprc.silverchair.com/jocn/article-pdf/16/3/427/1758106/089892904322926764.pdf by guest on 18 May 2021 Abstract & Artificial grammar learning (Reber, 1967) is a form of involved in using both sources of information as test stimuli implicit learning in which cognitive, rather than motor, were designed to unconfound chunk strength from rule use. implicit learning has been found. After viewing a series of Using functional connectivity analyses, the extent to which letter strings formed according to a finite state rule system, the sources of information are complementary or competi- people are able to classify new letter strings as to whether tive was also assessed. Activation in the right caudate was or not they are formed according to these grammatical rules associated with rule adherence, whereas medial temporal despite little conscious insight into the rule structure. lobe activations were associated with chunk strength. Addi- Previous research has shown that these classification judg- tionally, functional connectivity analyses revealed caudate ments are based on knowledge of abstract rules as well as and medial temporal lobe activations to be strongly superficial similarity (‘‘chunk strength’’) to training strings. negatively correlated (r = À.88) with one another during Here we used event-related fMRI to identify neural regions the performance of this task. & INTRODUCTION ard & Knowlton, 2002; Knowlton & Squire, 1996). How- Having good intuition about ‘‘what fits’’ and ‘‘what’s ever, there is a lack of consensus regarding the brain coming next’’ is essential to achieving one’s goals and regions that support implicit learning, what these re- meeting one’s obligations efficiently and effectively. Life gions contribute computationally, and the extent to is filled with scripts and recipes that have natural which the various neurocognitive mechanisms of implic- sequences and humans routinely take advantage of the it learning operate in a competitive or complementary predictability of these sequences to coordinate their fashion. There are at least three different reasons for this thought and behavior (Lieberman, 2000; Schank & Abel- discord, each embedded in the methodologies used to son, 1977). Automating this knowledge of sequential study the neural substrates of implicit learning. regularities has the additional benefit that the relevant First, most of the existing studies have focused on representations will be activated spontaneously in the neuropsychological populations. Several of these studies presence of the sequential cues. Social interactions suggest that the basal ganglia are involved in implicit which depend on the simultaneous coordination of learning, while medial temporal areas may not be. Pa- multiple processes undoubtedly benefit from an ability tients with Parkinson’s and Huntington’s disease have to automatically infer from facial expressions and tone of increasingly disturbed basal ganglia function over time. voice a great deal of information about another’s inten- Numerous studies have shown implicit learning to be tions, evaluations, and personality (Chartrand & Jefferis, absent or impaired in both groups (Knowlton, Mangels, in press; Lieberman & Rosenthal, 2001; Ambady & Rosen- & Squire, 1996; Knowlton, Squire, Paulsen, Swerdlow, & thal, 1993; Swann, Stein-Seroussi, & McNulty, 1992). Swenson, 1996; Gabrieli, 1995; Ferraro, Balota, & Can- Implicit learning refers to the ability to learn informa- nor, 1993; Knopman & Nissen, 1991; Heindel, Salmon, tional sequences when there is no conscious intent to Shultz, Welicke, & Butters, 1989; Martone, Butters, extract this sequential information and no explicit knowl- Payne, Becker, & Sax, 1984), but spared in patients with edge that this information has been learned (Stadler & medial temporal damage (Knowlton, Mangels, et al., Frensch, 1998; Seger, 1994; Reber, 1993). Presumably, 1996; Knowlton, Squire, et al., 1996; Knopman & Nissen, there are one or more neurocognitive mechanisms that 1987; Cohen & Squire, 1980). There have, however, been are able to learn recognizable features and statistical a number of studies showing that some forms of relationships between cues in sequential patterns (Pack- implicit learning are preserved in patients with Parkin- son’s or Huntington’s disease (Smith, Siegert, & McDo- wall, 2001; Peigneux, Meulemans, Van Der Linden, Salmon, & Petit, 1999; Reber & Squire, 1999; Daum 1University of California, Los Angeles, 2Harvard University et al., 1995; Gabrieli, 1995; Harrington, Haaland, Yeo, & D 2004 Massachusetts Institute of Technology Journal of Cognitive Neuroscience 16:3, pp. 427–438 Downloaded from http://www.mitpressjournals.org/doi/pdf/10.1162/089892904322926764 by guest on 29 September 2021 Marder, 1990). Some of the inherent limitations of five neuroimaging studies of the serial reaction time task neuropsychological investigations may prevent this re- have also implicated the basal ganglia (Berns, Cohen, & search method from completely characterizing the Mintun, 1997; Hazeltine, Grafton, & Ivry, 1997; Grafton, neural bases of implicit learning. Parkinson’s and Hun- Hazeltine, & Ivry, 1995; Rauch et al., 1995, 1997). tington’s disease both have complicated progressions Judgment forms of implicit learning have received involving multiple neural structures over many years, much less attention in terms of their neural loci and and the rate of decline, both neurally and psycholog- thus it is unknown whether these forms of implicit ically, show large individual differences (Grant & learning rely on the same neural structures as motor Adams, 1996). Of course, neuroimaging research has forms of implicit learning. The oldest and most famous Downloaded from http://mitprc.silverchair.com/jocn/article-pdf/16/3/427/1758106/089892904322926764.pdf by guest on 18 May 2021 major limitations of its own, but the combination of judgment implicit learning task is the artificial grammar neuropsychology with neuroimaging helps alleviate each task (Reber, 1967), in which letter strings are generated method’s weaknesses (Kosslyn & Intriligator, 1992). according to the rules of a Markovian grammar chain A second reason for the lack of consensus regarding the (see Figure 1). After being exposed to several ‘‘gram- neural underpinnings of implicit learning follows from matical’’ exemplars that conform to the rules of the the recent suggestion that there are at least two different grammar chain, participants are informed of the gram- forms of implicit learning. Seger (1997) proposed that mar, without being shown the rules, and are asked to there are both motor and judgment-linked forms of judge whether new letter strings conform to the gram- implicit learning.1 For instance, in the serial reaction time mar or not. Participants often express little confidence task that has been used many times, the critical depen- in their judgments, instead relying on their intuition or dent measure is reaction time. In a typical instantiation of ‘‘gut feeling.’’ Nevertheless, unimpaired participants this task, a target appears in a sequence of locations on consistently perform above chance on this task. With the screen and for each presentation, participants are neuropsychological tests of judgment implicit learning, required to press a button corresponding to the quadrant the results have been equivocal at best. Both Hunting- of the screen where the target appears as quickly as ton’s and Parkinson’s patients have shown preserved possible. Unbeknownst to participants, the sequence of learning on the artificial grammar task (Smith et al., presentation locations repeat every eight or so presenta- 2001; Peigneux et al., 1999; Reber & Squire, 1999). tions. Participants get faster over time as would be However, on the weather task, another judgment expected from basic practice effects. Nevertheless, when implicit learning task, both groups were impaired the underlying pattern is changed such that the old (Knowlton, Mangels, et al., 1996; Knowlton, Squire, pattern is no longer present, reaction times increase, et al., 1996), and two neuroimaging studies of the indicating a sensitivity to the underlying pattern despite weather task reported basal ganglia activation (Pol- little conscious knowledge thereof. With this implicit drack, Prabhakaran, Seger, & Gabrieli, 1999; Poldrack motor learning task, several neuropsychological studies et al., 2001). Thus, it is unclear to what extent the basal suggest that good performance depends on intact basal ganglia are involved in judgment implicit learning ganglia (Jackson, Jackson, Harrison, Handerson, & Ken- despite their clear role in motor implicit learning. nard, 1995; Ferraro et al., 1993; Willingham & Koroshetz, The third concern focuses on an aspect of the meth- 1993; Knopman & Nissen, 1991). Along these same lines, odology specific to artificial grammar research. The Figure 1. The Markovian grammar chain used to produce training and test items (taken from Knowlton & Squire, 1996). Strings are made by starting at IN and then following a path of arrows until OUT is reached. For each arrow traversed, the indicated letter is added to the letter string. 428 Journal of Cognitive Neuroscience Volume 16, Number 3 Downloaded from http://www.mitpressjournals.org/doi/pdf/10.1162/089892904322926764 by guest on 29 September
Details
-
File Typepdf
-
Upload Time-
-
Content LanguagesEnglish
-
Upload UserAnonymous/Not logged-in
-
File Pages12 Page
-
File Size-