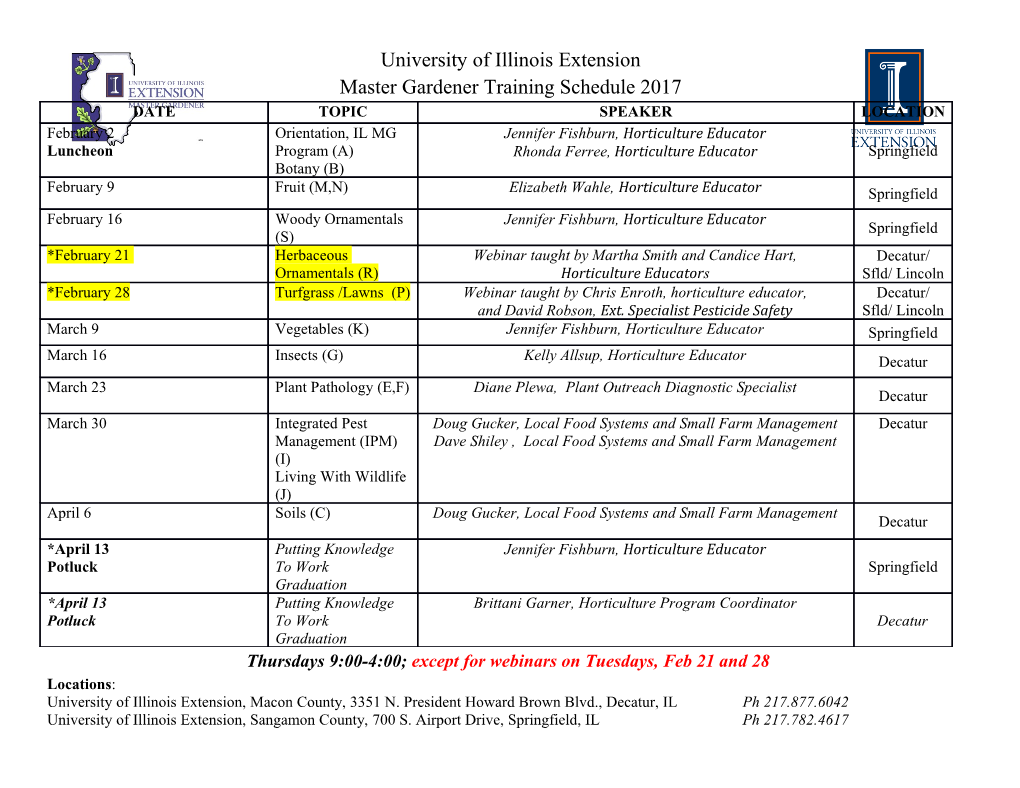
Causal Inference: Classical Approaches Si Yi (Cathy) Meng Feb 5, 2020 UBC MLRG Outline • Potential Outcomes • Confounding and Causal DAGs • Granger Causality • ICA for Causal Discovery AssociationalInference • Universe � • For each unit � ∈ �: • Attribute variable X(�) • Observed variable �(�) • Inference: • �(� = �|� = �) AssociationalInference Causal Inference • Universe � • Universe � • For each unit � ∈ �: • For each unit � ∈ �: • Attribute variable X(�) • Treatment variable � � ∈ {1,0} • Observed variable �(�) • Potential outcome �3 � , �4(�) • Inference: • Inference: • �(� = �|� = �) • �3 � − �4(�) Rubin’s Framework • For each unit � ∈ �: • Treatment variable � � ∈ {1,0} • Potential outcomes �3 � , �4(�) • the outcome that would be observed if treatment was set to � = 0 or 1, on the same unit. • (before) • If �(�) is set to 1 • �3 � is the observed outcome • �4 � is the counterfactual outcome • (after) Causal Effects • �3 � − �4 � is the causal effect of treatment 1 (relative to 0) on �. • Abbreviated as �3 and �4 Causal Effects • �3 � − �4 � is the causal effect of treatment 1 (relative to 0) on �. • Abbreviated as �3 and �4 • Fundamental Problem of Causal Inference • It is impossible to observe both �3 and �4 on the same unit, and therefore it is impossible to observe the causal effect. THE END Scientific solution to the Fundamental Problem • Assume temporal stability and causal transience • The value of �4 does not depend on when � = 0 is applied and measured. • The effect of � = 0 and the measurement process that gives rise to �4 does not change � enough to affect �3 measured later. Scientific solution to the Fundamental Problem • Assume temporal stability and causal transience • The value of �4 does not depend on when � = 0 is applied and measured. • The effect of � = 0 and the measurement process that gives rise to �4 does not change � enough to affect �3 measured later. • With these two assumptions, we can simply measure both �4 and �3 by applying � = 0 then � = 1, taking the measurement after each exposure. • Widely used in experiments involving physical devices. Scientific solution to the Fundamental Problem • Assume temporal stability and causal transience • The value of �4 does not depend on when � = 0 is applied and measured. • The effect of � = 0 and the measurement process that gives rise to �4 does not change � enough to affect �3 measured later. • With these two assumptions, we can simply measure both �4 and �3 by applying � = 0 then � = 1, taking the measurement after each exposure. • Widely used in experiments involving physical devices. • Assume unit homogeneity • For two units �3 and �6, we assume �4 �3 = �4 �6 and �3 �3 = �3 �6 . Scientific solution to the Fundamental Problem • Assume temporal stability and causal transience • The value of �4 does not depend on when � = 0 is applied and measured. • The effect of � = 0 and the measurement process that gives rise to �4 does not change � enough to affect �3 measured later. • With these two assumptions, we can simply measure both �4 and �3 by applying � = 0 then � = 1, taking the measurement after each exposure. • Widely used in experiments involving physical devices. • Assume unit homogeneity • For two units �3 and �6, we assume �4 �3 = �4 �6 and �3 �3 = �3 �6 . • Causal effect can then be computed using �3 �3 − �4 �6 . • Implies the constant effect assumption: �3 � − �4 � is the same for all � ∈ �. Scientific solution to the Fundamental Problem • Assume temporal stability and causal transience • The value of �4 does not depend on when � = 0 is applied and measured. • The effect of � = 0 and the measurement process that gives rise to �4 does not change � enough to affect �3 measured later. • With these two assumptions, we can simply measure both �4 and �3 by applying � = 0 then � = 1, taking the measurement after each exposure. • Widely used in experiments involving physical devices. • Assume unit homogeneity • For two units �3 and �6, we assume �4 �3 = �4 �6 and �3 �3 = �3 �6 . • Causal effect can then be computed using �3 �3 − �4 �6 . • Implies the constant effect assumption: �3 � − �4 � is the same for all � ∈ �. • It’s very difficult to argue that these are valid… Statistical solution to the Fundamental Problem • “What would have happened if I had not taken the flu shot” --> “What would the flu rate be if everyone got the flu shot vs if no one did?” Statistical solution to the Fundamental Problem • “What would have happened if I had not taken the flu shot” --> “What would the flu rate be if everyone got the flu shot vs if no one did?” • Average causal effect of � = 1 (relative to � = 0) over �: • � �3 − �4 = � �3) − �(�4 • Imagine parallel universes with the same population… • Can’t observe this. • Observed data can only give us information about the average of the outcome over � ∈ � exposed to � = �. • � �3|� = 1) − �(�4|� = 0 Statistical solution to the Fundamental Problem • “What would have happened if I had not taken the flu shot” --> “What would the flu rate be if everyone got the flu shot vs if no one did?” • Average causal effect of � = 1 (relative to � = 0) over �: • � �3 − �4 = � �3) − �(�4 • Imagine parallel universes with the same population… • Can’t observe this. • Observed data can only give us information about the average of the outcome over � ∈ � exposed to � = �. • � �3|� = 1) − �(�4|� = 0 • In general, � �:) ≠ �(�:|� = t Statistical solution to the Fundamental Problem • “What would have happened if I had not taken the flu shot” --> “What would the flu rate be if everyone got the flu shot vs if no one did?” • Average causal effect of � = 1 (relative to � = 0) over �: • � �3 − �4 = � �3) − �(�4 • Imagine parallel universes with the same population… • Can’t observe this. • Observed data can only give us information about the average of the outcome over � ∈ � exposed to � = �. • � �3|� = 1) − �(�4|� = 0 • In general, � �:) ≠ �(�:|� = t • Independence assumption hold via randomized treatment assignment allows equality to hold, which lets us compute the ACE above. Other assumptions • Stable Unit Treatment Value Assumption (SUVTA) • No interference: units do not interact with each other. • One version of treatment. • Consistency • The potential outcome �: is equal to the observed outcome if the actual treatment received is � = �. • Positivity • ℙ(�(�) = �) > 0 for all � and �. Other assumptions • Stable Unit Treatment Value Assumption (SUVTA) • No interference: units do not interact with each other. • One version of treatment. • Consistency • The potential outcome �: is equal to the observed outcome if the actual treatment received is � = �. • Positivity • ℙ(�(�) = �) > 0 for all � and �. • Ignorability (aka no unmeasured confounders assumption) • �4, �3 ⊥ T|X • Among people with the same features X, we can think of treatment T as being randomly assigned. Outline • Potential Outcome • Confounding and Causal DAGs • Granger Causality • ICA for Causal Discovery DAGs Court Order (CO) • Useful for identifying dependencies and ways to factor and simplify the joint distribution. B • � �3, … , �B = ∏ DE3 �(�D|� FG D ) Captain (C) Soldier A Soldier B Prisoner D Firing squad example [Pearl, 2018] DAGs Court Order (CO) • Useful for identifying dependencies and ways to factor and simplify the joint distribution. B • � �3, … , �B = ∏ DE3 �(�D|� FG D ) Captain • Two variables � and � are d-separated by a set of variables (C) � if � and � are conditionally independent given �. • � �, �|� = � � � �(�|�) • Chain Soldier A Soldier B • Fork • Inverted fork Prisoner D Firing squad example [Pearl, 2018] Causal DAGs Court Order (CO) • DAGs where directions of the edges represent causal relationships. • In contrast to Rubin’s potential outcome framework, this is a Captain structural approach to causal inference which Pearl (C) advocates. • They are shown to be mathematically equivalent. Soldier A Soldier B Prisoner D Firing squad example [Pearl, 2018] Intervention and Pearl’s do-calculus Court Order (CO) • ��() operator signals an intervention on a variable. • Replace that variable with the actual value that we assign. • Removes all incoming edges to that node. Captain (C) A=0 Soldier B Prisoner D Firing squad example [Pearl, 2018] Intervention and Pearl’s do-calculus Court Order (CO) • ��() operator signals an intervention on a variable. • Replace that variable with the actual value that we assign. • Removes all incoming edges to that node. Captain (C) • Instead of � � � = 0 • We want � � ��(� = 0 ) • The causal effect of � = 0 on D. A=0 Soldier B Prisoner D Firing squad example [Pearl, 2018] Confounding • Confounders: variables that influences both treatment and outcome. A B • Want: identify a set of variables so that ignorability holds. • We don’t need to identity specific confounders • We just need to be able to control for confounding. T Y • Need to block backdoor paths from � to �. D Frontdoor paths A B A B T Y T Z Y • We are not concerned about frontdoor paths. • We don’t want to control anything along the frontdoor paths. • Unless we care about the magnitude of the causal effect… Backdoor paths • Begins with a parent of � and ends at �. A B • Need to control these paths as they confound our causal effect. • How? • Identify the set of variables that blocks all backdoor paths from � to �. T Y D Backdoor criterion • A set of variables � satisfies the backdoor criterion if 1. it blocks all backdoor paths from � to �, and A B 2. It does not include any descendants of �. T Y D Backdoor criterion • A set of variables � satisfies the backdoor criterion if 1. it blocks all backdoor paths from � to �, and A B 2. It does not include any descendants of �. • � = �, � • Alternatively, � = �, � , � = �, �, � T Y D Backdoor criterion • A set of variables � satisfies the backdoor criterion if 1. it blocks all backdoor paths from � to �, and A B 2. It does not include any descendants of �. • � = �, � • Alternatively, � = �, � , � = �, �, � T Y • Controlling any of these sets allow us to control for confounding. D Backdoor criterion • A set of variables � satisfies the backdoor criterion if 1. it blocks all backdoor paths from � to �, and A B 2. It does not include any descendants of �.
Details
-
File Typepdf
-
Upload Time-
-
Content LanguagesEnglish
-
Upload UserAnonymous/Not logged-in
-
File Pages64 Page
-
File Size-